It is taking place: The robots are taking our jobs. No sick days, lavatory breaks, and no extra curly hairs in your buns. Simply chilly, arduous effectivity. Extra particularly, BurgerBot is a brand new fast-food joint the place robots are doing all of the work that people aren’t fascinated with, like burger meeting traces.
In Los Gatos, California, one of many San Francisco Bay Space’s extra prosperous areas, a shiny new fancy fast-food idea has simply popped up within one in every of its fashionable upscale brunch spots. ABB Robotics and BurgerBots have teamed up and unleashed a pair of IRB 360 FlexPickers and YuMi cobots (collaborative robots) to slap out some tasty burgers for the plenty – in 27 seconds, flat.
These machines do not simply stack US$18 all-beef patties, particular sauce, lettuce, cheese, pickles, onions on a sesame seed bun with surgical precision onto a QR-coded tray – they’re claimed to make completely constant burgers each single time with zero perspective. A far cry from little Billy, who’s by no means had a job earlier than and is on day one in every of coaching and experiencing rush hour for the primary time.
However earlier than we pull out the protest indicators and begin a picket line, here is some meals for thought: A full employees of people is employed on the restaurant. The bots (for now) solely deal with the burger manufacturing operation – from grinding the meat and griddling it as much as tossing it onto a conveyor belt meeting line. They then assemble the substances and kick out a whole, ready-to-eat burger again to a human server to be dished out to a ready visitor.
The robots do the repetitive stuff whereas supposedly leaving individuals extra time for hospitality and different people-y issues.
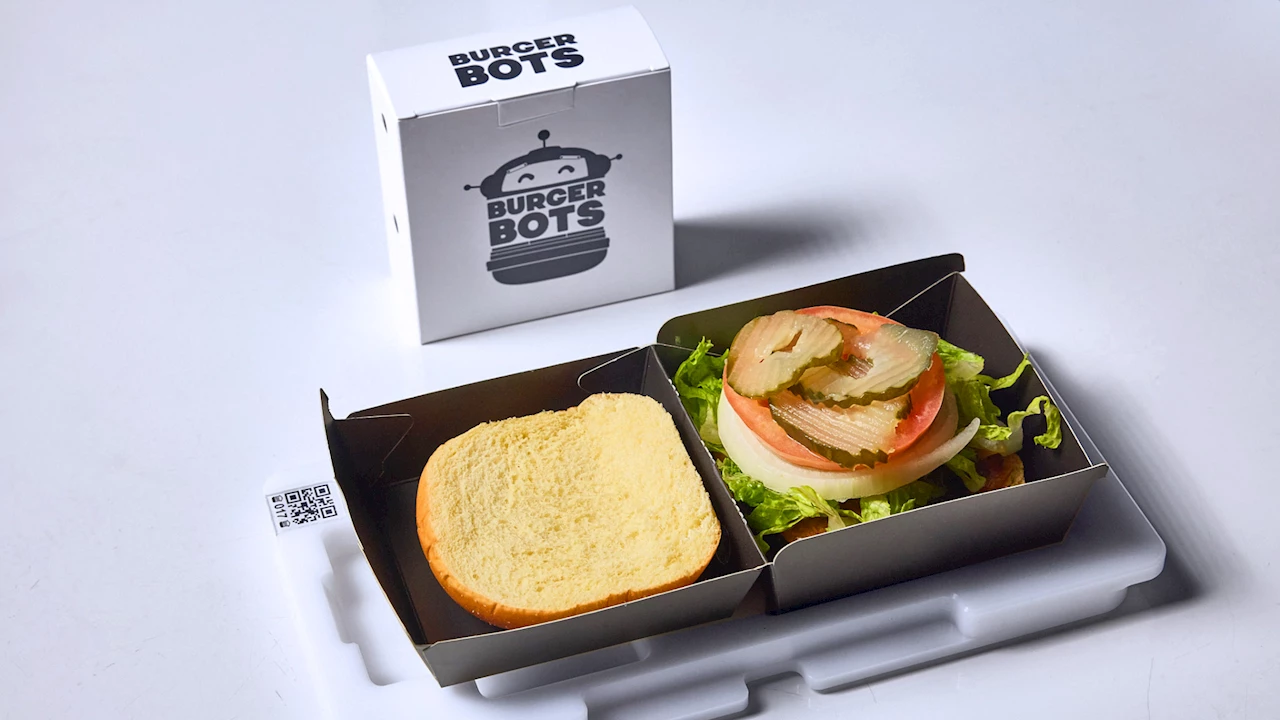
ABB
“The meals service business is dynamic and demanding, and our know-how brings industrial-grade consistency, effectivity and reliability to this area,” stated Marc Segura, President of ABB Robotics Division. “By taking up repetitive and time-consuming duties, robots permit employees to give attention to what issues most – creating memorable eating experiences.”
ABB surveyed 1,250 hospitality staff and located that 67% truly need robots to take over boring, gross, and harmful duties, and 63% had been excited by the prospect of a robotic making their job simpler. Ultimately, automation is not essentially about changing people, it is about upgrading all the system.
When the washer changed the washboard, individuals all over the place rejoiced.
BurgerBots is not only a Silicon Valley tech gimmick. It is designed for scalability, hygiene, and effectivity. ABB’s compact robotic cell combines the FlexPicker 360 – which grabs and stacks veggies and the like – and the YuMi robotic for remaining meeting as a field rolls down a conveyor. The system makes use of real-time stock monitoring from lettuce to condiments and all the things in between.
Robotic burger-making in 27 seconds!
BurgerBots has solely been open for roughly 24 hours on the time of writing, so time will inform the way it performs … although this is not ABB’s first foray into robotic meals prep. In 2021, ABB’s IRB 4600 ‘bot helped energy Roboeatz’s ARK (Autonomous Robotic Kitchen) in Latvia – claimed to be the world’s most superior autonomous kitchen that may whip up over 1,000 recipes from 80 recent substances.
In line with 2025 information from the World Financial Discussion board, automation and AI might result in the lack of roughly 92 million human jobs (about 8% of all jobs) by 2030. In direction of the highest of the potential record are positions like cashiers and fast-food staff. The primary duties to seemingly substitute human staff might be harmful, repetitive, or tedious jobs – and roles that do not significantly require excessive social or emotional intelligence.
That being stated, on the BurgerBot web site, the corporate is accepting resumes from certified people. Simply not for the burger-making place.
Supply: ABB Robotics