Over the past 2-3 years, synthetic intelligence (AI) brokers have change into extra embedded within the software program improvement course of. In accordance with Statista, three out of 4 builders, or round 75%, use GitHub Copilot, OpenAI Codex, ChatGPT, and different generative AI of their day by day chores.
Nonetheless, whereas AI exhibits promise in directing software program improvement duties, it creates a wave of authorized uncertainty.
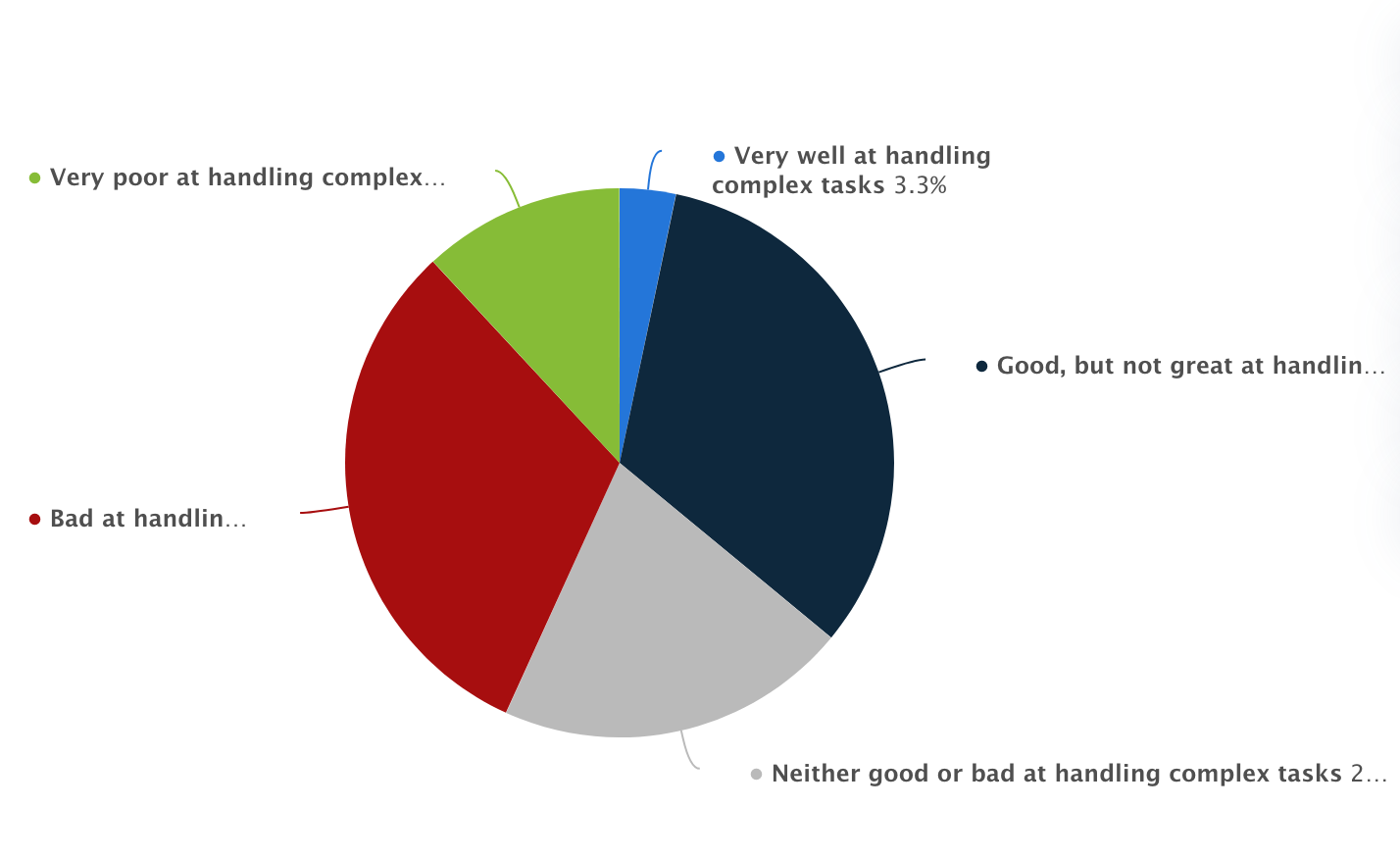
Capacity of Synthetic Intelligence in Managing Complicated Duties, Statista
Who owns the code written by an AI? What occurs if AI-made code infringes on another person’s mental property? And what are the privateness dangers when industrial information is processed by way of AI fashions?
To reply all these burning questions, we’ll clarify how AI improvement is regarded from the authorized facet, particularly in outsourcing instances, and dive into all issues firms ought to perceive earlier than permitting these instruments to combine into their workflows.
What Is AI in Customized Software program Improvement?
The marketplace for AI applied sciences is huge, amounting to round $244 billion in 2025. Usually, AI is split into machine studying and deep studying and additional into pure language processing, pc imaginative and prescient, and extra.
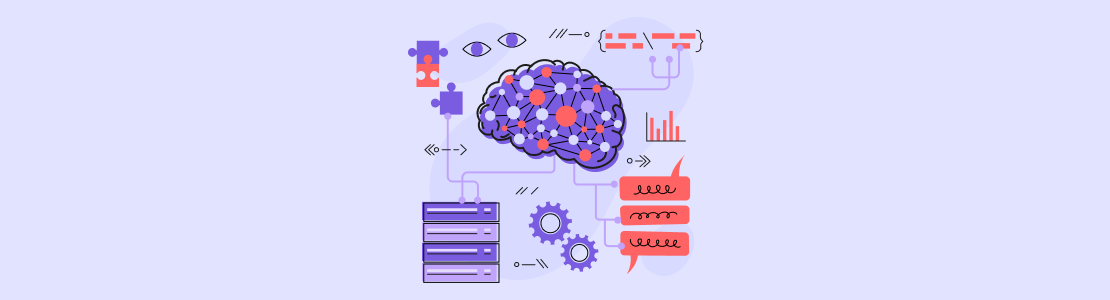
In software program improvement, AI instruments discuss with clever techniques that may help or automate components of the programming course of. They will recommend strains of code, full features, and even generate complete modules relying on context or prompts supplied by the developer.
Within the context of outsourcing tasks—the place velocity is not any much less essential than high quality—AI applied sciences are rapidly turning into staples in improvement environments.
They increase productiveness by taking redundant duties, lower the time spent on boilerplate code, and help builders who could also be working in unfamiliar frameworks or languages.
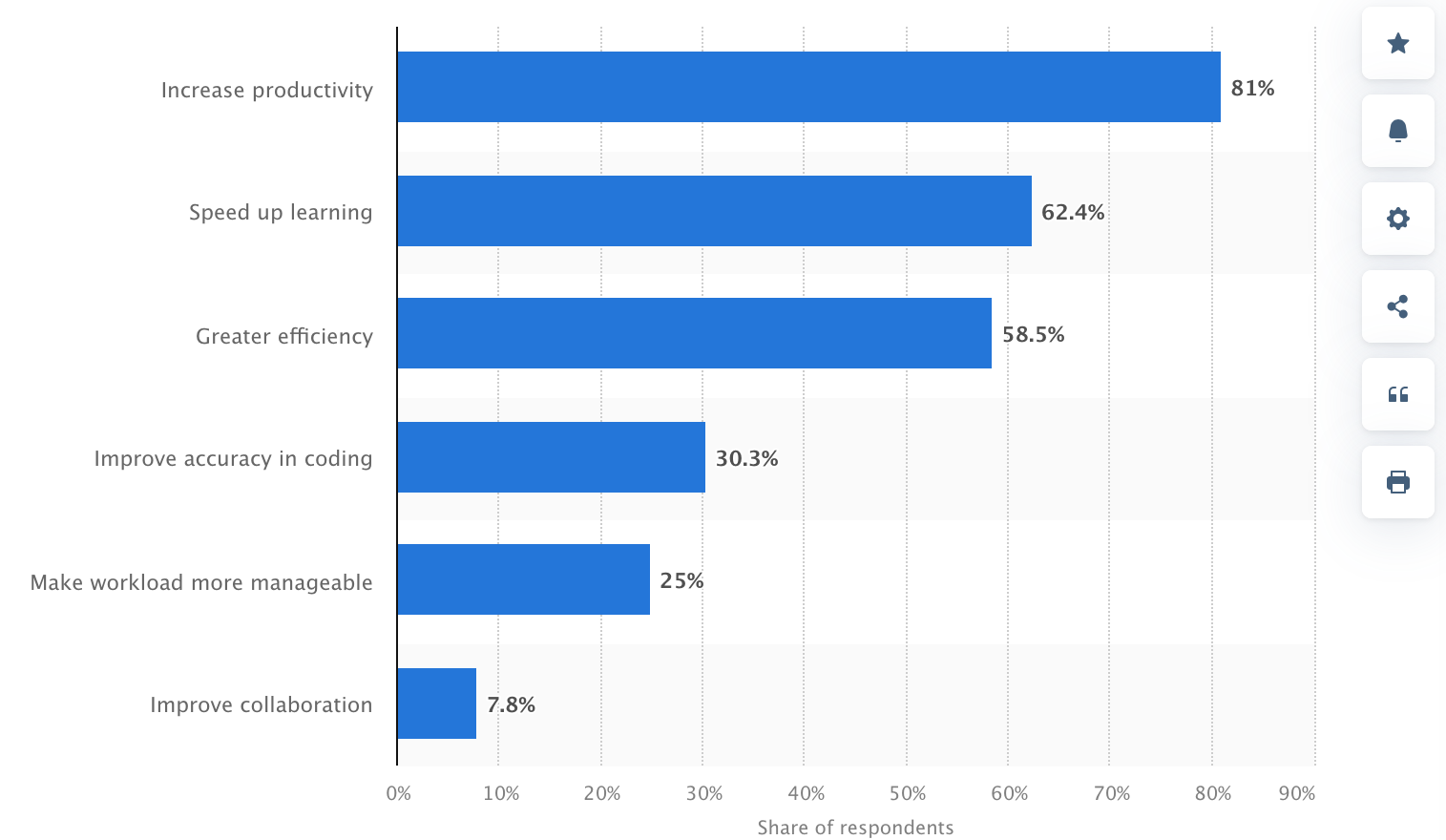
Advantages of Utilizing Synthetic Intelligence, Statista
How AI Instruments Can Be Built-in in Outsourcing Initiatives
Synthetic intelligence in 2025 has change into the specified ability for practically all technical professions.
Whereas the uncommon bartender or plumber might not require AI mastery to the identical stage, it has change into clear that including an AI ability to a software program developer’s arsenal is a should as a result of within the context of software program improvement outsourcing, AI instruments can be utilized in some ways:
- Code Technology: GitHub Copilot and different AI instruments help outsourced builders in coding by making hints or auto-filling features as they code.
- Bug Detection: As an alternative of ready for human verification in software program testing, AI can flag errors or dangerous code so groups can repair flaws earlier than they change into irreversible points.
- Writing Assessments: AI can independently generate take a look at instances from the code, thus the testing turns into faster and extra exhaustive.
- Documentation Assist: AI can depart feedback and draw up documentation explaining what the code does.
- Multi-language Assist: If the challenge wants to change programming languages, AI may also help translate or re-write segments of code with a purpose to decrease the necessity for specialised information for each programming language.
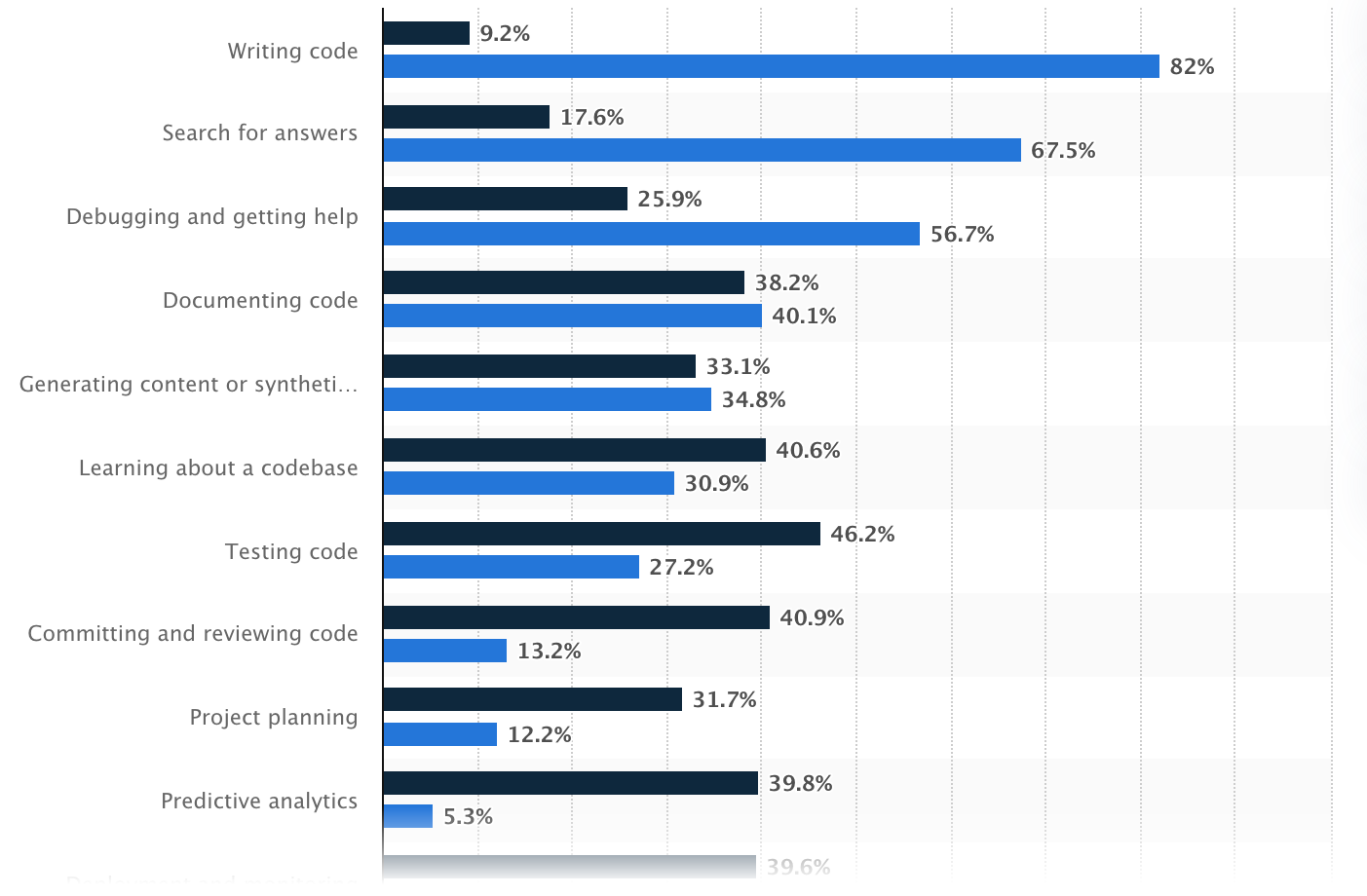
Hottest makes use of of AI within the improvement, Statista
Authorized Implications of Utilizing AI in Customized Software program Improvement
AI instruments might be extremely useful in software program improvement, particularly when outsourcing. However utilizing them additionally raises some authorized questions companies want to pay attention to, primarily round possession, privateness, and accountability.
Mental Property (IP) Points
When builders use AI instruments like GitHub Copilot, ChatGPT, or different code-writing assistants, it’s pure to ask: Who truly owns the code that will get written? This is among the trickiest authorized questions proper now.
At the moment, there’s no clear international settlement. Normally, AI doesn’t personal something, and the developer who makes use of the software is taken into account the “writer,” nonetheless, this may occasionally range.
The catch is that AI instruments study from tons of present code on the web. Generally, they generate code that’s very related (and even an identical) to the code they had been educated on, together with open-source tasks.
If that code is copied too intently, and it’s beneath a strict open-source license, you would run into authorized issues, particularly in the event you didn’t notice it or comply with the license guidelines.
Outsourcing could make it much more problematic. For those who’re working with an outsourcing group they usually use AI instruments throughout improvement, you have to be further clear in your contracts:
- Who owns the ultimate code?
- What occurs if the AI software unintentionally reuses licensed code?
- Is the outsourced group allowed to make use of AI instruments in any respect?
To 100% keep on the protected facet, you may:
- Be sure that contracts clearly state who owns the code.
- Double-check that the code doesn’t violate any licenses.
- Think about using instruments that run regionally or restrict what the AI sees to keep away from leaking or copying restricted content material.
Knowledge Safety and Privateness
When utilizing AI instruments in software program improvement, particularly in outsourcing, one other main consideration is information privateness and safety. So what’s the danger?
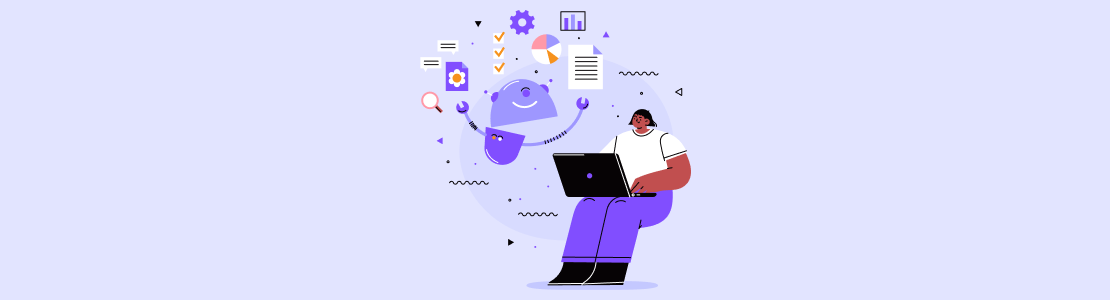
The vast majority of AI instruments like ChatGPT, Copilot, and others usually run within the cloud, which implies the knowledge builders put into them could also be transmitted to outer servers.
If builders copy and paste proprietary code, login credentials, or industrial information into these instruments, that data may very well be retained, reused, and later revealed. The state of affairs turns into even worse if:
- You’re giving confidential enterprise data
- Your challenge issues buyer or person particulars
- You’re in a regulated trade akin to healthcare or finance
So what does the regulation say concerning it? Certainly, totally different international locations have totally different laws, however essentially the most noticeable are:
- GDPR (Europe): In easy phrases, GDPR protects private information. For those who collect information from folks within the EU, you must clarify what you’re amassing, why you want it, and get their permission first. Folks can ask to see their information, rectify something unsuitable, or have it deleted.
- HIPAA (US, healthcare): HIPAA covers non-public well being data and medical data. Submitting to HIPAA, you may’t simply paste something associated to affected person paperwork into an AI software or chatbot—particularly one which runs on-line. Additionally, in the event you work with different firms (outsourcing groups or software program distributors), they should comply with the identical decrees and signal a particular settlement to make all of it authorized.
- CCPA (California): CCPA is a privateness regulation that provides folks extra management over their private information. If your small business collects information from California residents, you must allow them to know what you’re gathering and why. Folks can ask to see their information, have it deleted, or cease you from sharing or promoting it. Even when your organization is predicated some other place, you continue to must comply with CCPA in the event you’re processing information from folks in California.
The obvious and logical query right here is how one can defend information. First, don’t put something delicate (passwords, buyer information, or non-public firm information) into public AI instruments until you’re certain they’re protected.
For tasks that concern confidential data, it’s higher to make use of AI assistants that run on native computer systems and don’t ship something to the web.
Additionally, take a great take a look at the contracts with any outsourcing companions to ensure they’re following the correct practices for protecting information protected.
Accountability and Duty
AI instruments can perform many duties however they don’t take accountability when one thing goes unsuitable. The blame nonetheless falls on folks: the builders, the outsourcing group, and the enterprise that owns the challenge.
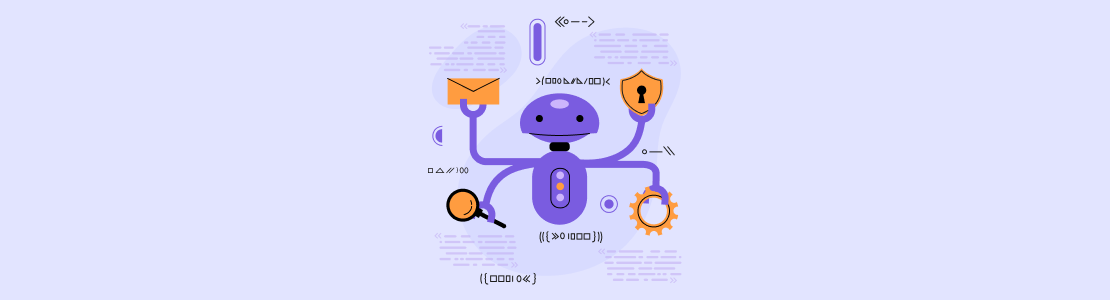
If the code has a flaw, creates a security hole, or causes injury, it’s not the AI’s guilt—it’s the folks utilizing it who’re accountable. If nobody takes possession, small compromises can flip into massive (and costly) points.
To keep away from this case, companies want clear instructions and human oversight:
- At all times evaluate AI-generated code. It’s simply a place to begin, not a completed product. Builders nonetheless must probe, debug, and confirm each single half.
- Assign accountability. Be it an in-house group or an outsourced accomplice, ensure somebody is clearly accountable for high quality management.
- Embody AI in your contracts. Your settlement with an outsourcing supplier ought to say:
- Whether or not they can apply AI instruments.
- Who’s accountable for reviewing the AI’s work.
- Who pays for fixes if one thing goes unsuitable due to AI-generated code.
- Preserve a report of AI utilization. Doc when and the way AI instruments are utilized, particularly for main code contributions. That means, if issues emerge, you may hint again what occurred.
Case Research and Examples
AI in software program improvement is already a standard observe utilized by many tech giants although statistically, smaller firms with fewer workers are extra probably to make use of synthetic intelligence than bigger firms.
Beneath, we have now compiled some real-world examples that present how totally different companies are making use of AI and the teachings they’re studying alongside the best way.
Nabla (Healthcare AI Startup)
Nabla, a French healthtech firm, built-in GPT-3 (by way of OpenAI) to help medical doctors with writing medical notes and summaries throughout consultations.
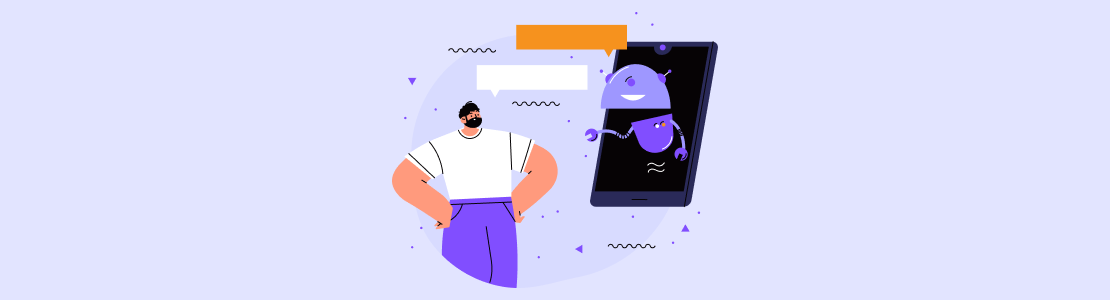
How they use it:
- AI listens to patient-doctor conversations and creates structured notes.
- The time medical doctors spend on admin work visibly shrinks.
Authorized & privateness actions:
- As a result of they function in a healthcare setting, Nabla deliberately selected to not use OpenAI’s API straight resulting from issues about information privateness and GDPR compliance.
- As an alternative, they constructed their very own safe infrastructure utilizing open-source fashions like GPT-J, hosted regionally, to make sure no affected person information leaves their servers.
Lesson realized: In privacy-sensitive industries, utilizing self-hosted or non-public AI fashions is usually a safer path than counting on industrial cloud-based APIs.
Replit and Ghostwriter
Replit, a collaborative on-line coding platform, developed Ghostwriter, its personal AI assistant just like Copilot.
The way it’s used:
- Ghostwriter helps customers (together with newcomers) write and full code proper within the browser.
- It’s built-in throughout Replit’s improvement platform, usually utilized in training and startups.
Problem:
- Replit has to stability ease of use with license compliance and transparency.
- The corporate offers disclaimers encouraging customers to evaluate and edit the generated code, underlining it is just a tip.
Lesson realized: AI-generated code is highly effective however not all the time protected to make use of “as is.” Even platforms that construct AI instruments themselves push for human evaluate and warning.
Amazon’s Inner AI Coding Instruments
Amazon has developed its personal inside AI-powered instruments, just like Copilot, to help its builders.
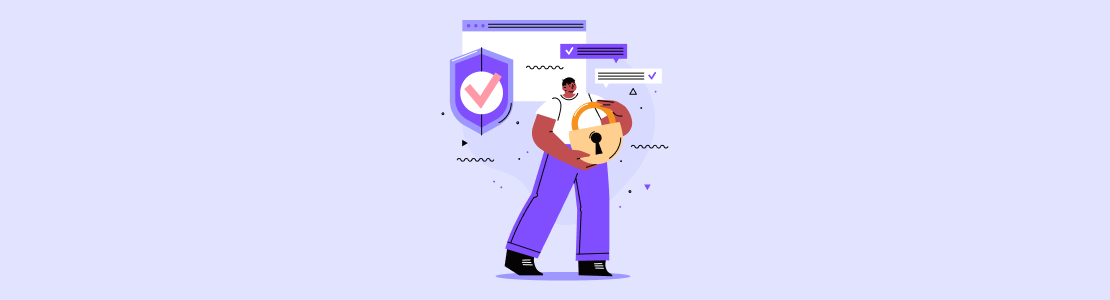
How they use it:
- AI helps builders write and evaluate code throughout a number of groups and companies.
- It’s used internally to enhance developer productiveness and velocity up supply.
Why they don’t use exterior instruments like Copilot:
- Amazon has strict inside insurance policies round mental property and information privateness.
- They like to construct and host instruments internally to sidestep authorized dangers and defend proprietary code.
Lesson realized: Giant enterprises usually keep away from third-party AI instruments resulting from issues about IP leakage and lack of management over prone information.
How one can Safely Use AI Instruments in Outsourcing Initiatives: Basic Suggestions
Utilizing AI instruments in outsourced improvement can deliver sooner supply, decrease prices, and coding productiveness. However to do it safely, firms must arrange the correct processes and protections from the beginning.
First, it’s essential to make AI utilization expectations clear in contracts with outsourcing companions. Agreements ought to specify whether or not AI instruments can be utilized, beneath what circumstances, and who’s accountable for reviewing and validating AI-generated code.
These contracts also needs to embrace robust mental property clauses, spelling out who owns the ultimate code and what occurs if AI unintentionally introduces open-source or third-party licensed content material.
Knowledge safety is one other important concern. If builders use AI instruments that ship information to the cloud, they have to by no means enter delicate or proprietary data until the software complies with GDPR, HIPAA, or CCPA.
In extremely regulated industries, it’s all the time safer to make use of self-hosted AI fashions or variations that run in a managed surroundings to reduce the danger of information openness.
To keep away from authorized and high quality points, firms also needs to implement human oversight at each stage. AI instruments are nice for recommendation, however they don’t perceive enterprise context or authorized necessities.
Builders should nonetheless take a look at, audit, and reanalyze all code earlier than it goes stay. Establishing a code evaluate workflow the place senior engineers double-check AI output ensures security and accountability.
It’s additionally clever to doc when and the way AI instruments are used within the improvement course of. Holding a report helps hint again the supply of any future defects or authorized issues and exhibits good religion in regulatory audits.
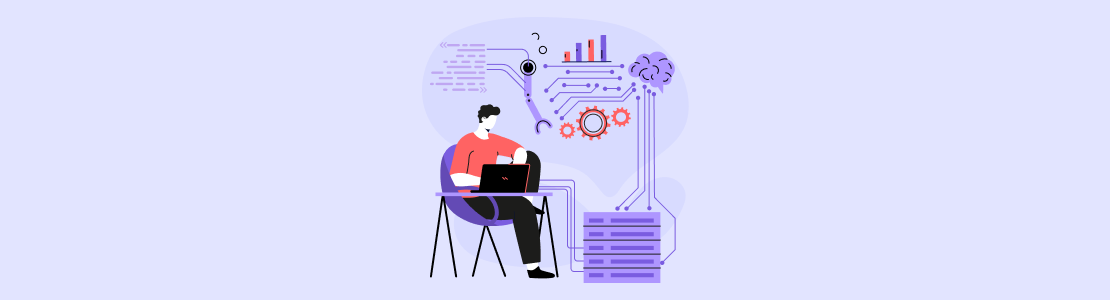
Lastly, ensure your group (or your outsourcing accomplice’s group) receives primary coaching in AI finest practices. Builders ought to perceive the restrictions of AI strategies, how one can detect licensing dangers, and why it’s essential to validate code earlier than delivery it.
FAQ
Q: Who owns the code generated by AI instruments?
Possession often goes to the corporate commissioning the software program—however provided that that’s clearly acknowledged in your settlement. The complication comes when AI instruments generate code that resembles open-source materials. If that content material is beneath a license, and it’s not attributed correctly, it might increase mental property points. So, clear contracts and guide checks are key.
Q: Is AI-generated code protected to make use of as-is?
Not all the time. AI instruments can unintentionally reproduce licensed or copyrighted code, particularly in the event that they had been educated on public codebases. Whereas the strategies are helpful, they need to be handled as beginning factors—builders nonetheless must evaluate, edit, and confirm the code earlier than it’s used.
Q: Is it protected to enter delicate information into AI instruments like ChatGPT?
Often, no. Until you’re utilizing a non-public or enterprise model of the AI that ensures information privateness, you shouldn’t enter any confidential or proprietary data. Public instruments course of information within the cloud, which might expose it to privateness dangers and regulatory violations.
Q: What information safety legal guidelines ought to we contemplate?
This is determined by the place you use and what sort of information you deal with. In Europe, the GDPR requires consent and transparency when utilizing private information. Within the U.S., HIPAA protects medical information, whereas CCPA in California offers customers management over how their private data is collected and deleted. In case your AI instruments contact delicate information, they have to adjust to these laws.
Q: Who’s accountable if AI-generated code causes an issue?
Finally, the accountability falls on the event group—not the AI software. Meaning whether or not your group is in-house or outsourced, somebody must validate the code earlier than it goes stay. AI can velocity issues up, however it will probably’t take accountability for errors.
Q: How can we safely use AI instruments in outsourced tasks?
Begin by placing the whole lot in writing: your contracts ought to cowl AI utilization, IP possession, and evaluate processes. Solely use trusted instruments, keep away from feeding in delicate information, and ensure builders are educated to make use of AI responsibly. Most significantly, hold a human within the loop for high quality assurance.
Q: Does SCAND use AI for software program improvement?
Sure, however supplied that the consumer agrees. If public AI instruments are licensed, we use Microsoft Copilot in VSCode and Cursor IDE, with fashions like ChatGPT 4o, Claude Sonnet, DeepSeek, and Qwen. If a consumer requests a non-public setup, we use native AI assistants in VSCode, Ollama, LM Studio, and llama.cpp, with the whole lot saved on safe machines.
Q: Does SCAND use AI to check software program?
Sure, however with permission from the consumer. We use AI instruments like ChatGPT 4o and Qwen Imaginative and prescient for automated testing and Playwright and Selenium for browser testing. When required, we robotically generate unit exams utilizing AI fashions in Copilot, Cursor, or regionally accessible instruments like Llama, DeepSeek, Qwen, and Starcoder.