The iPhone’s video digicam has gotten so good that it’s usually used as an alternative of a costlier skilled digicam. Add the truth that an iPhone is far smaller–you’ll be able to’t put {most professional} video cameras in a pocket–and the iPhone has grow to be a sexy videographer’s instrument.
Conventional video cameras do have a bonus in that they provide extra capabilities for fine-tuning. However the iPhone is making an attempt to compete at that degree, too, with Apple’s launch of Ultimate Reduce Digital camera for iPhone. Packed into the app is the power to tweak digicam settings resembling white stability, ISO, and shutter velocity. Handbook focus and frame-rate changes additionally add to the general minutia of potentialities.
When used as a companion to the brand new Ultimate Reduce Professional for iPad 2.0, you’ll discover a well-oiled video manufacturing ecosystem. Now you’ll be able to unlock Dwell Multicam, along with the standard enhancing capabilities which have been enhancing on the iPad expertise over time. For a content material creator or fanatic, right here’s a have a look at how the mixture of the Ultimate Reduce Digital camera app and Ultimate Reduce Professional for iPad 2.0 works.
Ultimate Reduce Professional for iPad 2.0
Professional’s Ranking
Professionals
- Nice for enhancing whereas cell with fast-to-insert options
- Dwell Multicam performance presents good flexibility
- New enhancing options make the app extra strong
Cons
- Not appropriate for complicated workflows or nuanced enhancing
- iPadOS limitations can disrupt workflow
Our Verdict
Whereas the Mac model presents higher instruments and workflow for classy tasks, Ultimate Reduce Professional for iPad 2.0 turns into extra strong with its Dwell Multicam help and new instruments for shade grading, soundtracks, and extra.
Ultimate Reduce Professional for iPad 2.0 brings varied enhancements. Contemplating the brand new and highly effective M4 iPad Professional, any replace that will increase performance to take extra benefit of the brand new {hardware} is welcomed. iPadOS nonetheless lacks the extra highly effective file system performance of macOS, nevertheless, making group for big tasks harder. For smaller tasks resembling social media posts, the iPadOS does a superb job of offering velocity and effectivity with the Ultimate Reduce Professional app.
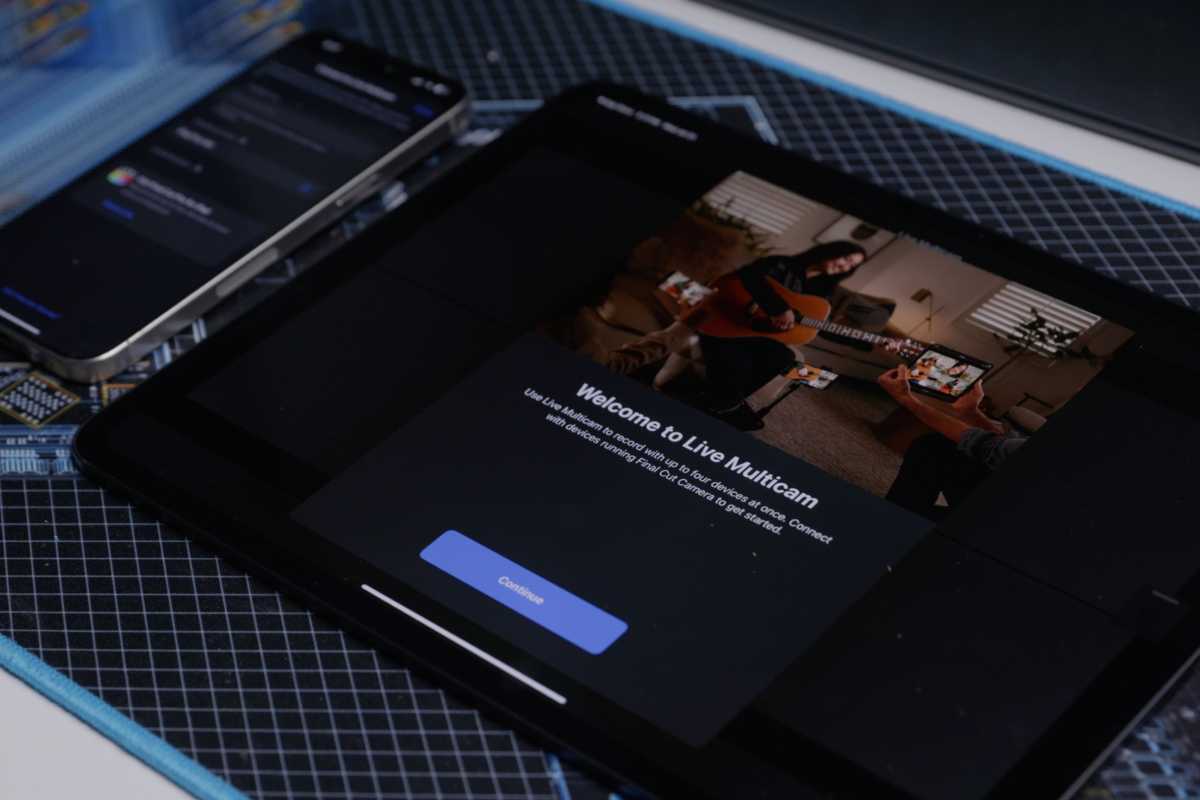
Dwell Multicam is a tour de pressure characteristic for Ultimate Reduce Professional for iPad.
Thiago Trevisan/Foundry
Ultimate Reduce Professional for iPad has a subscription price of $4.99 month-to-month or $49.99 yearly. Whereas apps resembling iMovie are free, the added performance supplied definitely provides worth to the paid Ultimate Reduce Professional for iPad for the appropriate consumer.
Dwell Multicam
The largest addition to Ultimate Reduce Professional for iPad is the combination with Ultimate Reduce Digital camera and help for Dwell Multicam. It permits customers to file as much as 4 completely different angles, depending on the variety of linked iPhones/iPads. For those who join your iPhone to your iPad, you’ll be able to see two completely different angles, for instance. It’s then only a matter of intelligent positioning and a few planning for good digicam angles.
Multicam photographs are sometimes executed with a number of conventional cameras and will be tough to correctly implement. The best way that Ultimate Reduce Professional for iPad easily permits this performance to seamlessly movement is appreciated. Whereas utilizing the Ultimate Reduce Digital camera app, you too can management the opposite linked cameras. With the power to cease solely the iPhone digicam or all cameras, there’s good flexibility right here. This remote-control performance is one thing that historically requires a number of operators and complicated digicam gear to drag off.
The prowess of the M4 iPad Professional makes enhancing footage from the iPhone clean and straightforward, even when a number of angles and cameras are used. The power for iPhone and iPad to have exterior storage gadgets with their USB-C connections is nice for big file sizes and workflow.
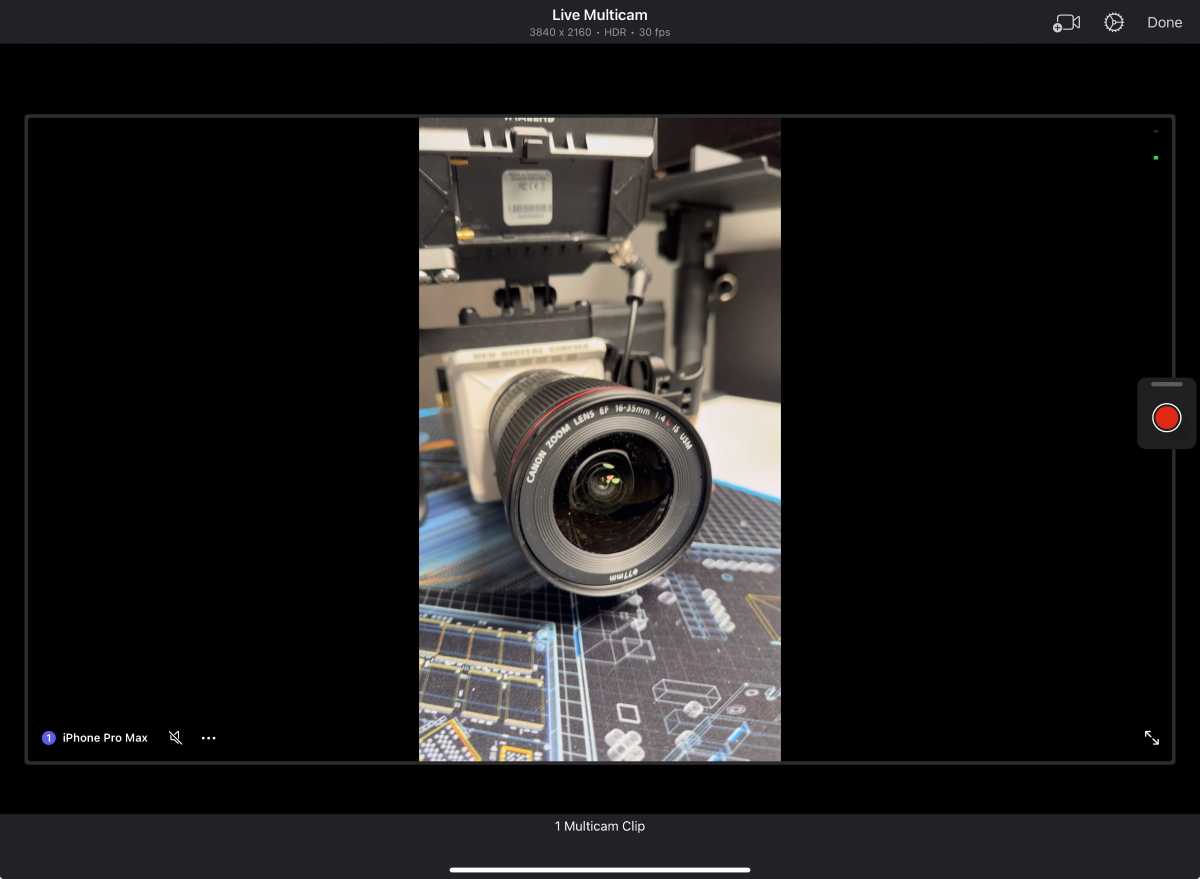
Dwell Multicam
makes Ultimate Reduce Professional for iPad a instrument that may develop
video manufacturing affordably.
Thiago Trevisan/Foundry
Shade grading
Shade grading is a staple within the workflow of each novice {and professional} video editors alike. With 12 completely different shade grading presets jammed in, there’s now far more utility when utilizing the iPad for enhancing. These are presets, so customized shade grading for high-level work remains to be off-limits. The nuanced shade grading of huge complicated tasks remains to be extra appropriate for Ultimate Reduce Professional on a Mac.
For a lot of, the flexibleness of the iPad provides loads of cause to make use of to make use of the quicker presets, nevertheless. Lots of the presets can look nice with a easy click on, particularly for social-media content material creators on the go along with time constraints for fine-tuning.
Shade scopes for gauging your visuals are right here, however there are some limitations with look-up tables (LUTs). You’ll be able to solely use the built-in LUTs in Ultimate Reduce Professional For iPad, and never customized LUTs as you’ll usually use on Ultimate Reduce Professional for Mac.
Whereas the colour grading pipeline has these simpler out-of-the-box experiences for editors, the arduous limits on capabilities might push some in direction of the macOS model.
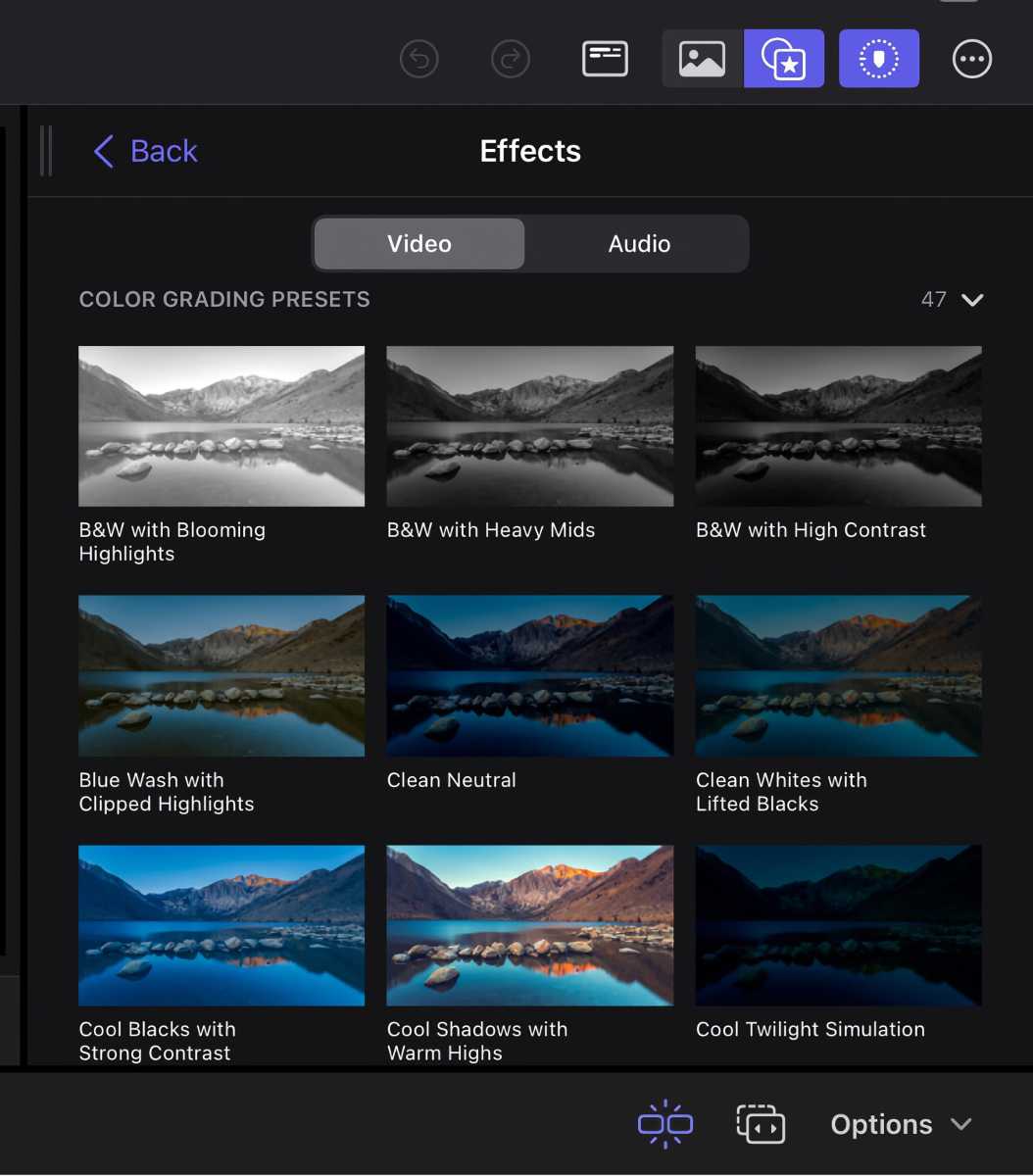
The brand new shade gradients are preset
so it’s good to go to the Mac to do fine-tuning
.
Foundry
Soundtracks
What’s a superb film with out a good soundtrack to accompany it? On Ultimate Reduce Professional for iPad, 20 soundtracks are added for drop-in ease of use whereas enhancing. No must subscribe to third-party music companies if these suffice, which is what many on-the-go editors are on the lookout for. By going to the soundtracks within the browser, you’ll be able to peruse the picks out there earlier than making a selection. Merely drag it to your timeline, and also you now have a soundtrack to your video. The soundtracks are dynamic–they robotically alter and match into no matter video size you could have going. That reduces the time wanted to edit the audio, which is one other characteristic that reveals the deal with effectivity for editors.
Take into accout if you wish to deliver this challenge externally to a Mac, you have to to export the challenge with the audio file being an AAC audio file.
Dynamic glitch backgrounds
Including some aptitude to a video is one thing that takes time, however gadgets resembling glitch backgrounds assist to quicken the workflow and get typically good outcomes. There are numerous different background choices additionally out there so as to add some pizzaz. Added patterns and different shapes will be added, in a straight-forward method with no need an excessive amount of customization.
Transitions are additionally out there, with computerized cross-fading of audio if it was already a part of the video. In fact, movie grain and different related results are also within the iPad’s toolbox.
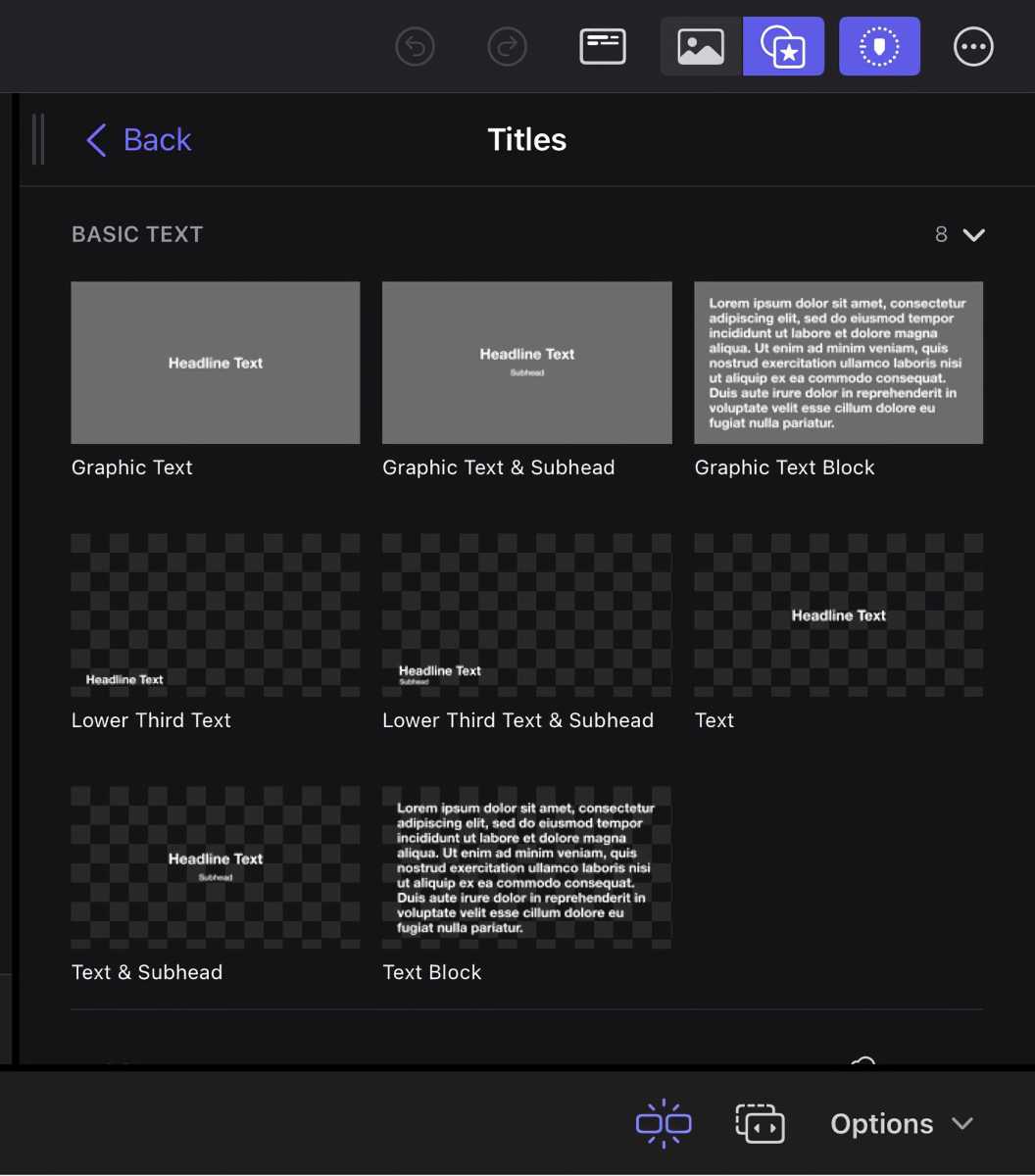
Foundry
New primary textual content titles
Titles are necessary, and textual content title choices will let you get a superb begin on framing what it’s you wish to convey to your viewers. Along with lower-third titles and bumpers, you could have the choice to make your titles static or animate them. As soon as once more, including a title is so simple as dragging it to the timeline, and you’ll fine-tune the length. For titles, you’ll have to obtain them individually from throughout the app.
Ultimate Reduce Digital camera for iPhone is free, appropriate solely with an iPhone working iOS 17.4 or later. Ultimate Reduce Digital camera can work on an iPad, however the iPad’s digicam is sorely missing in comparison with the iPhone. However if you wish to create a multicam setup, you should utilize a mix of iPhones and iPads.

Ultimate Reduce Digital camera presents extra adjustment choices than the iPhone Digital camera app.
Thiago Trevisan/Foundry
Whereas iPhone {hardware} has improved each era with a heavy deal with its cameras, the unique Digital camera app nonetheless lacks the performance {that a} skilled desires. Ultimate Reduce Digital camera gives loads of nuance to tinker with. For those who use your iPhone as an alternative of a standard digicam, the expertise of adjusting the digicam may be very related, other than the iPhone contact interface.
With Ultimate Reduce Digital camera, all of it begins with selecting the baseline attributes for what you propose to movie. Selections resembling body charge, codec, and focal size all mirror what a standard digicam presents.
Apple ProRes and HEVC H.265 are the 2 codec choices out there, with ProRes being extra storage heavy–in the event you’re utilizing iPhone 15 Professional or Professional Max, you should utilize these telephones’ skill to file on to an exterior SSD hooked up by way of USB-C. Additionally, in the case of processing the video, Apple silicon can chew by means of both codec with out breaking a sweat on the iPad Professional or Mac.
The power to decide on between HDR and SDR Rec. 709 can also be right here, which is apt for the reason that iPad Professional is HDR-capable. To high it off, 720p, 1080p, and 4K resolutions are all choices. The perfect decision selection will rely upon how giant of a file measurement you desire to balanced out with the sharpness of the decision.
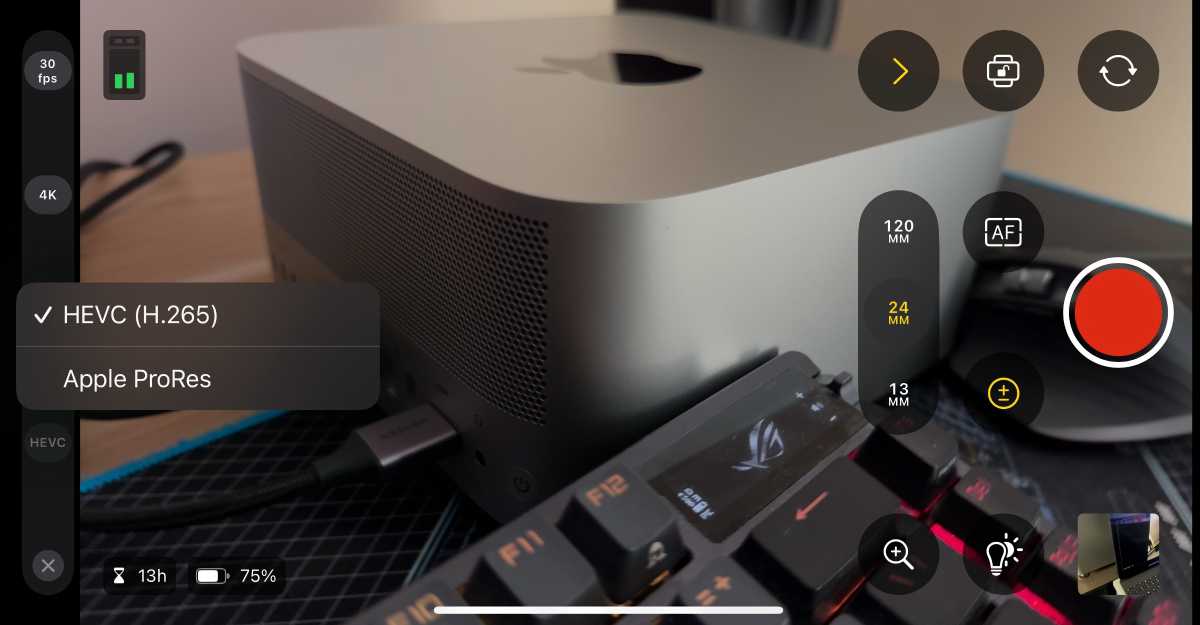
Apple ProRes and HEVC H.265 are the 2 codec choices out there, with ProRes requiring extra cupboard space.
Thiago Trevisan/Foundry
For body charges, 24 to 60 fps choices can be found. PAL or 25 fps can be chosen for elevated flexibility. White stability and publicity are additionally right here in a camera-friendly format that’s simple to digest and use.
You’ll have some selection in focal size to your lens. With the iPhone 15 Professional Max’s three-lens digicam, the widest lens can be 13mm. A standard 24mm is subsequent, topped off with a long-range 120mm focal size. These are fastened focal lengths, however you’ll be able to nonetheless pinch to zoom. In Ultimate Reduce Digital camera, the main focus will be set robotically or be manually managed. The handbook focus is correct and satisfying to make use of, with predictably clean transitions. Very similar to a standard digicam and lens, you’ll be able to rack focus as you see match with the visible assist of the screens as a information. Within the settings, you can find the choice to show focus peaking on, which can assist you fine-tune your focus throughout use.
Audio choices are additionally out there in settings, permitting you to decide on the enter supply. The default can be “This Gadget”–the iPhone getting used. This can permit customers to attach different microphones and related setups for extra difficult multicam utilization.
A strong mixture
The simplicity of the iPhone’s Digital camera app is interesting on a grand scale, however professionals want extra management. Ultimate Reduce Professional Digital camera provides pro-level performance. The truth that it’s free is a good plus and one thing that needs to be extra akin to the inventory digicam app going ahead.
Third-party apps such because the Blackmagic Digital camera can be found and generally provide extra performance than the Ultimate Reduce Digital camera app. Nevertheless, the combination with Ultimate Reduce Professional for iPad is seamless and offers additional video usability to each gadgets. Multicam Dwell is the massive promoting level right here, coupled with the power to effortlessly edit video on the iPad.
Whereas the desktop Ultimate Reduce Professional is extra totally featured, the on-the-go nature of the iPad model can match the invoice for a lot of workflows. The subscription worth is a turn-off but it surely does present worth to these searching for to utilize the multicam options coupled with the Ultimate Reduce Digital camera app for iPhone. The addition of recent goodies, resembling extra color-grading presets, soundtracks, and titles, make it a superb all-in-one bundle.
Ultimate Reduce Professional for iPad app is targeted on quick turnaround. You seemingly won’t substitute your macOS enhancing software program anytime quickly in the event you demand extra complicated workflows, However with enhancing {hardware}, the iPad software program can also be present process nuanced adjustments to make it extra viable and seamless. With extra succesful cameras seemingly coming with the iPhone 16 Professional, the longer term is vibrant with potentialities because the {hardware} improves. With each energy and ease of use, the iPhone can proceed to erode a few of the conventional use instances that had been the staple of normal digicam methods.