A compelling new examine from Germany critiques the EU AI Act’s definition of the time period ‘deepfake’ as overly imprecise, significantly within the context of digital picture manipulation. The authors argue that the Act’s emphasis on content material resembling actual individuals or occasions – but probably showing pretend – lacks readability.
Additionally they spotlight that the Act’s exceptions for ‘normal modifying’ (i.e., supposedly minor AI-aided modifications to pictures) fail to think about each the pervasive affect of AI in client purposes and the subjective nature of creative conventions that predate the appearance of AI.
Imprecise laws on these points offers rise to 2 key dangers: a ‘chilling impact,’ the place the regulation’s broad interpretive scope stifles innovation and the adoption of latest methods; and a ‘scofflaw impact,’ the place the regulation is disregarded as overreaching or irrelevant.
In both case, imprecise legal guidelines successfully shift the duty of building sensible authorized definitions onto future courtroom rulings – a cautious and risk-averse method to laws.
AI-based image-manipulation applied sciences stay notably forward of laws’s capability to deal with them, it appears. For example, one noteworthy instance of the rising elasticity of the idea of AI-driven ‘automated’ post-processing, the paper observes, is the ‘Scene Optimizer’ operate in latest Samsung cameras, which can change user-taken photos of the moon (a difficult topic), with an AI-driven, ‘refined’ picture:
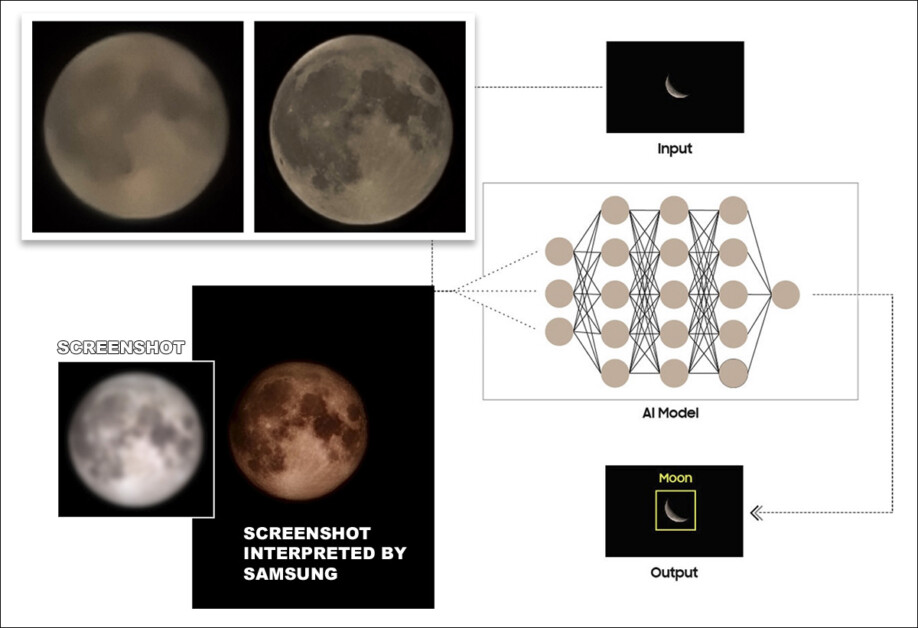
High left, an instance from the brand new paper of an actual user-taken picture of the moon, to the left of a Samsung-enhanced model routinely created with Scene Optimizer; Proper, Samsung’s official illustration of the method behind this; decrease left, examples from the Reddit consumer u/ibreakphotos, displaying (left) a intentionally blurred picture of the moon and (proper), Samsung’s re-imagining of this picture – although the supply photograph was an image of a monitor, and never the actual moon. Sources (clockwise from top-left): https://arxiv.org/pdf/2412.09961; https://www.samsung.com/uk/help/mobile-devices/how-galaxy-cameras-combine-super-resolution-technologies-with-ai-to-produce-high-quality-images-of-the-moon/; https:/reddit.com/r/Android/feedback/11nzrb0/samsung_space_zoom_moon_shots_are_fake_and_here/
Within the lower-left of the picture above, we see two photos of the moon. The one on the left is a photograph taken by a Reddit consumer. Right here, the picture has been intentionally blurred and downscaled by the consumer.
To its proper we see a photograph of the identical degraded picture taken with a Samsung digicam with AI-driven post-processing enabled. The digicam has routinely ‘augmented’ the acknowledged ‘moon’ object, although it was not the actual moon.
The paper ranges deeper criticism on the Greatest Take characteristic integrated into Google’s latest smartphones – a controversial AI characteristic that edits collectively the ‘greatest’ components of a gaggle photograph, scanning a number of seconds of a pictures sequence in order that smiles are shuffled ahead or backward in time as needed – and no-one is proven in the midst of blinking.
The paper contends this type of composite course of has the potential to misrepresent occasions:
‘[In] a typical group photograph setting, a median viewer would in all probability nonetheless think about the ensuing photograph as genuine. The smile which is inserted existed inside a few seconds from the remaining photograph being taken.
‘Then again, the ten second time-frame of the very best take characteristic is ample for a temper change. An individual may need stopped smiling whereas the remainder of the group laughs a couple of joke at their expense.
‘As a consequence, we assume that such a gaggle photograph might properly represent a deep pretend.’
The new paper is titled What constitutes a Deep Pretend? The blurry line between respectable processing and manipulation underneath the EU AI Act, and comes from two researchers on the Computational Regulation Lab on the College of Tübingen, and Saarland College.
Previous Methods
Manipulating time in pictures is much older than consumer-level AI. The brand new paper’s authors observe the existence of a lot older methods that may be argued as ‘inauthentic’, such because the concatenation of a number of sequential photos right into a Excessive Dynamic Vary (HDR) photograph, or a ‘stitched’ panoramic photograph.
Certainly, among the oldest and most amusing photographic fakes have been historically created by school-children working from one finish of a faculty group to a different, forward of the trajectory of the particular panoramic cameras that have been as soon as used for sports activities and college group pictures – enabling the pupil to look twice in the identical picture:
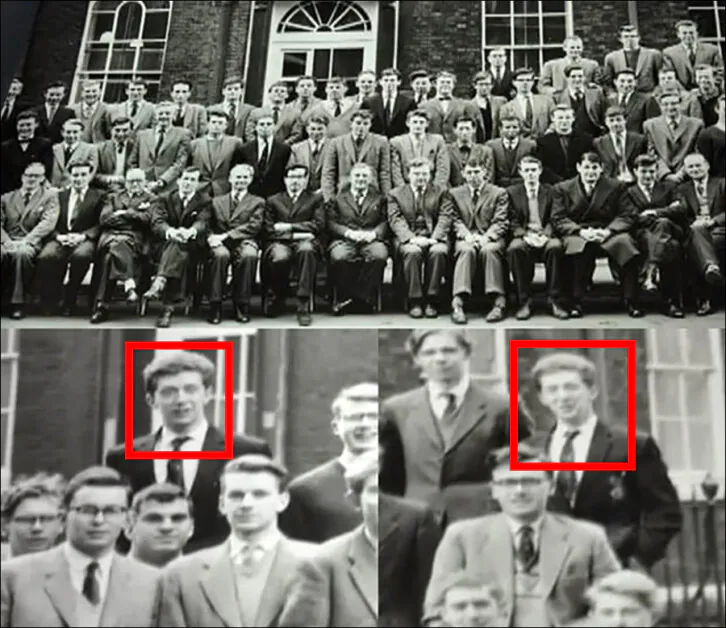
The temptation to trick panoramic cameras throughout group pictures was an excessive amount of to withstand for a lot of college students, who have been keen to threat a foul session on the head’s workplace to be able to ‘clone’ themselves at school pictures. Supply: https://petapixel.com/2012/12/13/double-exposure-a-clever-photo-prank-from-half-a-century-ago/
Except you are taking a photograph in RAW mode, which principally dumps the digicam lens sensor to a really giant file with none type of interpretation, it is doubtless that your digital pictures usually are not fully genuine. Digicam methods routinely apply ‘enchancment’ algorithms resembling picture sharpening and white steadiness, by default – and have finished so because the origins of consumer-level digital pictures.
The authors of the brand new paper argue that even these older kinds of digital photograph augmentation don’t characterize ‘actuality’, since such strategies are designed to make pictures extra pleasing, no more ‘actual’.
The examine means that the EU AI Act, even with later amendments resembling recitals 123–27, locations all photographic output inside an evidentiary framework unsuited to the context through which pictures are produced as of late, versus the (nominally goal) nature of safety digicam footage or forensic pictures. Most photos addressed by the AI Act usually tend to originate in contexts the place producers and on-line platforms actively promote artistic photograph interpretation, together with the usage of AI.
The researchers recommend that pictures ‘have by no means been an goal depiction of actuality’. Issues such because the digicam’s location, the depth of area chosen, and lighting decisions, all contribute to make {a photograph} deeply subjective.
The paper observes that routine ‘clean-up’ duties – resembling eradicating sensor mud or undesirable energy strains from an in any other case well-composed scene – have been solely semi-automated earlier than the rise of AI: customers needed to manually choose a area or provoke a course of to attain their desired consequence.
At this time, these operations are sometimes triggered by a consumer’s textual content prompts, most notably in instruments like Photoshop. On the client degree, such options are more and more automated with out consumer enter – an consequence that’s apparently regarded by producers and platforms as ‘clearly fascinating’.
The Diluted That means of ‘Deepfake’
A central problem for laws round AI-altered and AI-generated imagery is the anomaly of the time period ‘deepfake’, which has had its which means notably prolonged during the last two years.
Initially the phrases utilized solely to video output from autoencoder-based methods resembling DeepFaceLab and FaceSwap, each derived from nameless code posted to Reddit in late 2017.
From 2022, the approaching of Latent Diffusion Fashions (LDMs) resembling Secure Diffusion and Flux, in addition to text-to-video methods resembling Sora, would additionally permit identity-swapping and customization, at improved decision, versatility and constancy. Now it was potential to create diffusion-based fashions that might depict celebrities and politicians. For the reason that time period’ deepfake’ was already a headline-garnering treasure for media producers, it was prolonged to cowl these methods.
Later, in each the media and the analysis literature, the time period got here additionally to incorporate text-based impersonation. By this level, the unique which means of ‘deepfake’ was all however misplaced, whereas its prolonged which means was continually evolving, and more and more diluted.
However because the phrase was so incendiary and galvanizing, and was by now a strong political and media touchstone, it proved inconceivable to surrender. It attracted readers to web sites, funding to researchers, and a spotlight to politicians. This lexical ambiguity is the primary focus of the brand new analysis.
Because the authors observe, article 3(60) of the EU AI Act outlines 4 situations that outline a ‘deepfake’.
1: True Moon
Firstly, the content material have to be generated or manipulated, i.e., both created from scratch utilizing AI (technology) or altered from current information (manipulation). The paper highlights the issue in distinguishing between ‘acceptable’ image-editing outcomes and manipulative deepfakes, on condition that digital pictures are, in any case, by no means true representations of actuality.
The paper contends {that a} Samsung-generated moon is arguably genuine, because the moon is unlikely to vary look, and because the AI-generated content material, skilled on actual lunar photos, is subsequently more likely to be correct.
Nonetheless, the authors additionally state that because the Samsung system has been proven to generate an ‘enhanced’ picture of the moon in a case the place the supply picture was not the moon itself, this might be thought of a ‘deepfake’.
It might be impractical to attract up a complete listing of differing use-cases round this type of advert hoc performance. Due to this fact the burden of definition appears to go, as soon as once more, to the courts.
2: TextFakes
Secondly, the content material have to be within the type of picture, audio, or video. Textual content content material, whereas topic to different transparency obligations, isn’t thought of a deepfake underneath the AI Act. This isn’t coated in any element within the new examine, although it could possibly have a notable bearing on the effectiveness of visible deepfakes (see under).
3: Actual World Issues
Thirdly, the content material should resemble current individuals, objects, locations, entities, or occasions. This situation establishes a connection to the actual world, which means that purely fabricated imagery, even when photorealistic, wouldn’t qualify as a deepfake. Recital 134 of the EU AI Act emphasizes the ‘resemblance’ facet by including the phrase ‘appreciably’ (an obvious deferral to subsequent authorized judgements).
The authors, citing earlier work, think about whether or not an AI-generated face want belong to an actual particular person, or whether or not it want solely be adequately comparable to an actual particular person, to be able to fulfill this definition.
For example, how can one decide whether or not a sequence of photorealistic photos depicting the politician Donald Trump has the intent of impersonation, if the pictures (or appended texts) don’t particularly point out him? Facial recognition? Consumer surveys? A decide’s definition of ‘frequent sense’?
Returning to the ‘TextFakes’ challenge (see above), phrases usually represent a good portion of the act of a visible deepfake. For example, it’s potential to take an (unaltered) picture or video of ‘particular person a’, and say, in a caption or a social media put up, that the picture is of ‘particular person b’ (assuming the 2 individuals bear a resemblance).
In resembling case, no AI is required, and the consequence could also be strikingly efficient – however does such a low-tech method additionally represent a ‘deepfake’?
4: Retouch, Transform
Lastly, the content material should seem genuine or truthful to an individual. This situation emphasizes the notion of human viewers. Content material that’s solely acknowledged as representing an actual particular person or object by an algorithm would not be thought of a deepfake.
Of all of the situations in 3(60), this one most clearly defers to the later judgment of a courtroom, because it doesn’t permit for any interpretation through technical or mechanized means.
There are clearly some inherent difficulties in reaching consensus on such a subjective stipulation. The authors observe, for example, that totally different individuals, and various kinds of individuals (resembling kids and adults), could also be variously disposed to imagine in a selected deepfake.
The authors additional observe that the superior AI capabilities of instruments like Photoshop problem conventional definitions of ‘deepfake.’ Whereas these methods might embody fundamental safeguards in opposition to controversial or prohibited content material, they dramatically increase the idea of ‘retouching.’ Customers can now add or take away objects in a extremely convincing, photorealistic method, attaining knowledgeable degree of authenticity that redefines the boundaries of picture manipulation.
The authors state:
‘We argue that the present definition of deep fakes within the AI act and the corresponding obligations usually are not sufficiently specified to deal with the challenges posed by deep fakes. By analyzing the life cycle of a digital photograph from the digicam sensor to the digital modifying options, we discover that:
‘(1.) Deep fakes are ill-defined within the EU AI Act. The definition leaves an excessive amount of scope for what a deep pretend is.
‘(2.) It’s unclear how modifying capabilities like Google’s “greatest take” characteristic might be thought of as an exception to transparency obligations.
‘(3.) The exception for considerably edited photos raises questions on what constitutes substantial modifying of content material and whether or not or not this modifying have to be perceptible by a pure particular person.’
Taking Exception
The EU AI Act incorporates exceptions that, the authors argue, might be very permissive. Article 50(2), they state, gives an exception in instances the place nearly all of an authentic supply picture isn’t altered. The authors observe:
‘What might be thought of content material within the sense of Article 50(2) in instances of digital audio, photos, and movies? For instance, within the case of photos, do we have to think about the pixel-space or the seen house perceptible by people? Substantive manipulations within the pixel house won’t change human notion, and then again, small perturbations within the pixel house can change the notion dramatically.’
The researchers present the instance of including a hand-gun to the photograph an individual who’s pointing at somebody. By including the gun, one is altering as little as 5% of the picture; nevertheless, the semantic significance of the modified portion is notable. Due to this fact plainly this exception doesn’t take account of any ‘commonsense’ understanding of the impact a small element can have on the general significance of a picture.
Part 50(2) additionally permits exceptions for an ‘assistive operate for traditional modifying’. For the reason that Act doesn’t outline what ‘normal modifying’ means, even post-processing options as excessive as Google’s Greatest Take would appear to be protected by this exception, the authors observe.
Conclusion
The said intention of the brand new work is to encourage interdisciplinary examine across the regulation of deepfakes, and to behave as a place to begin for brand new dialogues between laptop scientists and authorized students.
Nonetheless, the paper itself succumbs to tautology at a number of factors: it regularly makes use of the time period ‘deepfake’ as if its which means have been self-evident, while taking intention on the EU AI Act for failing to outline what really constitutes a deepfake.
First revealed Monday, December 16, 2024