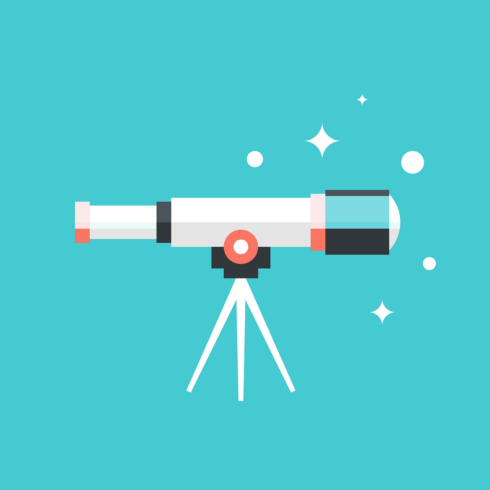
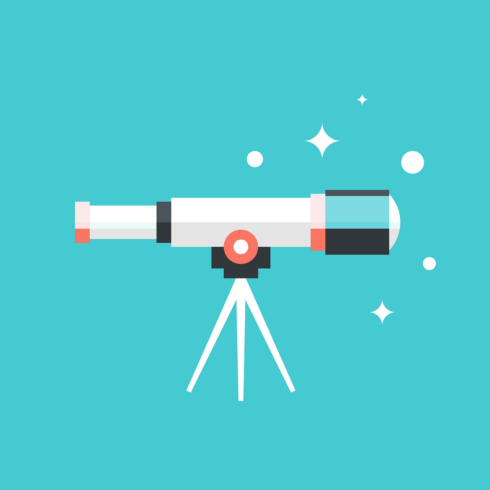
In at the moment’s quickly evolving digital panorama, the complexity of distributed methods and microservices architectures has reached unprecedented ranges. As organizations try to take care of visibility into their more and more intricate tech stacks, observability has emerged as a crucial self-discipline.
On the forefront of this subject stands OpenTelemetry, an open-source observability framework that has gained important traction lately. OpenTelemetry helps SREs generate observability knowledge in constant (open requirements) knowledge codecs for simpler evaluation and storage whereas minimizing incompatibility between vendor knowledge sorts. Most business analysts consider that OpenTelemetry will turn into the de facto normal for observability knowledge within the subsequent 5 years.
Nonetheless, as methods develop extra advanced and the quantity of information grows exponentially, so do the challenges in troubleshooting and sustaining them. Generative AI guarantees to enhance the SRE expertise and tame complexity. Specifically, AI assistants based mostly on retrieval augmented technology (RAG) are accelerating root trigger evaluation (RCA) and enhancing buyer experiences.
The observability problem
Observability supplies full visibility into system and software conduct, efficiency, and well being utilizing a number of indicators equivalent to logs, metrics, traces, and profiling. But, the fact usually must catch up. DevOps groups and SREs steadily discover themselves drowning in a sea of logs, metrics, traces, and profiling knowledge, struggling to extract significant insights shortly sufficient to forestall or resolve points. Step one is to leverage OpenTelemetry and its open requirements to generate observability knowledge in constant and comprehensible codecs. That is the place the intersection of OpenTelemetry, GenAI, and observability turns into not simply priceless, however important.
RAG-based AI assistants: A paradigm shift
RAG represents a major leap ahead in AI expertise. Whereas LLMs can present priceless insights and proposals leveraging public area experience from OpenTelemetry information bases within the public area, the ensuing steering will be generic and of restricted use. By combining the ability of enormous language fashions (LLMs) with the flexibility to retrieve and leverage particular, related inner info (equivalent to GitHub points, runbooks, buyer points, and extra), RAG-based AI Assistants provide a stage of contextual understanding and problem-solving functionality that was beforehand unattainable. Moreover, the RAG-based AI Assistant can retrieve and analyze real-time telemetry from OTel and correlate logs, metrics, traces, and profiling knowledge with suggestions and finest practices from inner operational processes and the LLM’s information base.
In analyzing incidents with OpenTelemetry, AI assistants that may assist SREs:
- Perceive advanced methods: AI assistants can comprehend the intricacies of distributed methods, microservices architectures, and the OpenTelemetry ecosystem, offering insights that bear in mind the total complexity of recent tech stacks.
- Provide contextual troubleshooting: By analyzing patterns throughout logs, metrics, and traces, and correlating them with identified points and finest practices, RAG-based AI assistants can provide troubleshooting recommendation that’s extremely related to the precise context of every distinctive atmosphere.
- Predict and forestall points: Leveraging huge quantities of historic knowledge and patterns, these AI assistants will help groups transfer from reactive to proactive observability, figuring out potential points earlier than they escalate into crucial issues.
- Speed up information dissemination: In quickly evolving fields like observability, maintaining with finest practices and new strategies is difficult. RAG-based AI assistants can function always-up-to-date information repositories, democratizing entry to the newest insights and methods.
- Improve collaboration: By offering a typical information base and interpretation layer, these AI assistants can enhance collaboration between growth, operations, and SRE groups, fostering a shared understanding of system conduct and efficiency.
Operational effectivity
For organizations seeking to keep aggressive, embracing RAG-based AI assistants for observability isn’t just an operational determination—it’s a strategic crucial. It helps total operational effectivity by way of:
- Diminished imply time to decision (MTTR): By shortly figuring out root causes and suggesting focused options, these AI assistants can dramatically cut back the time it takes to resolve points, decrease downtime, and enhance total system reliability.
- Optimized useful resource allocation: As an alternative of getting extremely expert engineers spend hours sifting by way of logs and metrics, RAG-based AI assistants can deal with the preliminary evaluation, permitting human consultants to deal with extra advanced, high-value duties.
- Enhanced decision-making: With AI assistants offering data-driven insights and proposals, groups could make extra knowledgeable choices about system structure, capability planning, and efficiency optimization.
- Steady studying and enchancment: As these AI Assistants accumulate extra knowledge and suggestions, their capability to offer correct and related insights will frequently enhance, making a virtuous cycle of enhanced observability and system efficiency.
- Aggressive benefit: Organizations that efficiently leverage RAG AI Assistants of their observability practices will be capable of innovate sooner, keep extra dependable methods, and finally ship higher experiences to their prospects.
Embracing the AI-augmented future in observability
The mix of RAG-based AI assistants and open supply observability frameworks like OpenTelemetry represents a transformative alternative for organizations of all sizes. Elastic, which is OpenTelemetry native, and provides a RAG-based AI assistant, is an ideal instance of this mixture. By embracing this expertise, groups can transcend the restrictions of historically siloed monitoring and troubleshooting approaches, transferring in direction of a way forward for proactive, clever, and extremely environment friendly system administration.
As leaders within the tech business, it’s crucial that we not solely acknowledge this shift however actively put together our organizations to leverage it. This implies investing in the fitting instruments and platforms, upskilling our groups, and fostering a tradition that embraces AI as a collaborator in our quest to attain the promise of observability.
The way forward for observability is right here, and it’s powered by synthetic intelligence. Those that acknowledge and act on this actuality at the moment might be finest positioned to thrive within the advanced digital ecosystems of tomorrow.
To study extra about Kubernetes and the cloud native ecosystem, be part of us at KubeCon + CloudNativeCon North America, in Salt Lake Metropolis, Utah, on November 12-15, 2024.