A brand new collaboration between College of California Merced and Adobe provides an advance on the state-of-the-art in human picture completion – the much-studied process of ‘de-obscuring’ occluded or hidden components of photos of individuals, for functions akin to digital try-on, animation and photo-editing.
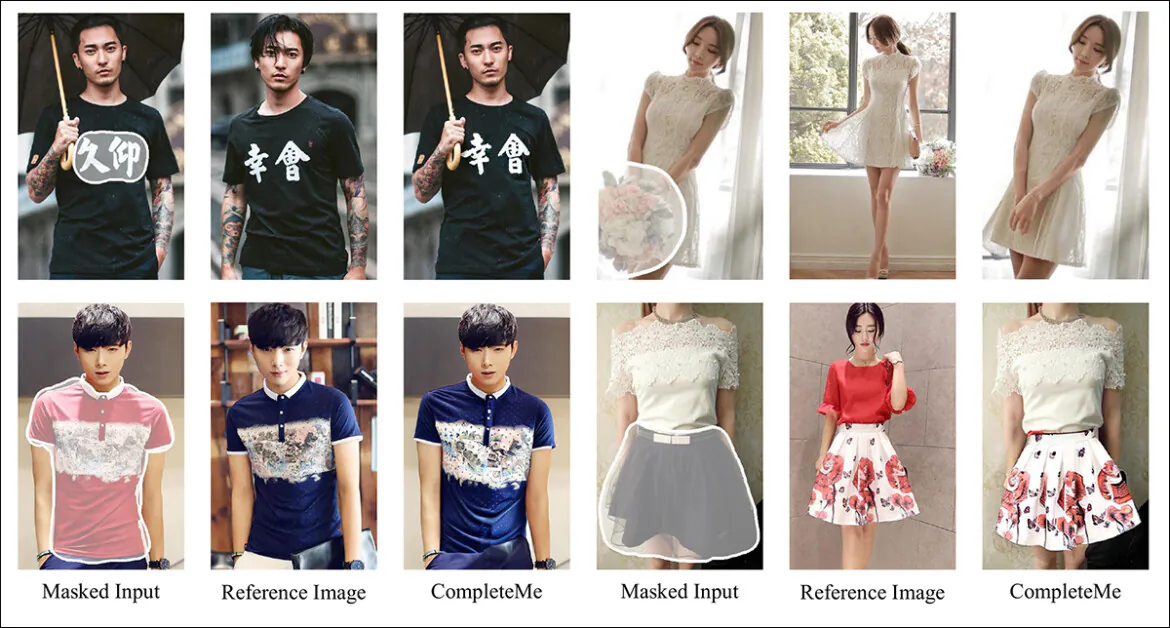
In addition to repairing broken photos or altering them at a person’s whim, human picture completion techniques akin to CompleteMe can impose novel clothes (by way of an adjunct reference picture, as within the center column in these two examples) into current photos. These examples are from the intensive supplementary PDF for the brand new paper. Supply: https://liagm.github.io/CompleteMe/pdf/supp.pdf
The new method, titled CompleteMe: Reference-based Human Picture Completion, makes use of supplementary enter photos to ‘recommend’ to the system what content material ought to change the hidden or lacking part of the human depiction (therefore the applicability to fashion-based try-on frameworks):
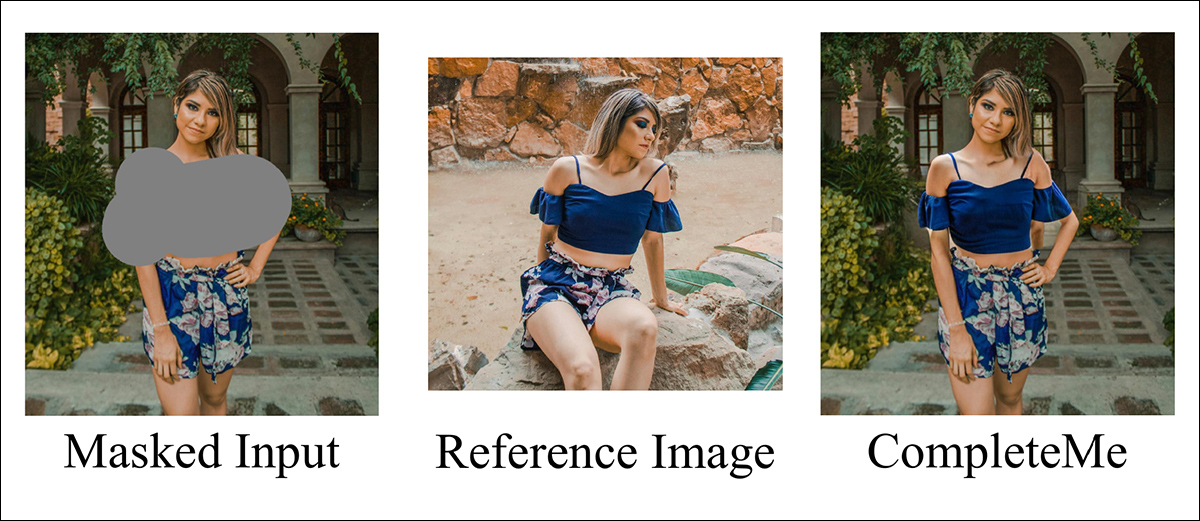
The CompleteMe system can conform reference content material to the obscured or occluded a part of a human picture.
The brand new system makes use of a twin U-Internet structure and a Area-Targeted Consideration (RFA) block that marshals sources to the pertinent space of the picture restoration occasion.
The researchers additionally provide a brand new and difficult benchmark system designed to judge reference-based completion duties (since CompleteMe is a part of an current and ongoing analysis strand in laptop imaginative and prescient, albeit one which has had no benchmark schema till now).
In exams, and in a well-scaled person research, the brand new technique got here out forward in most metrics, and forward total. In sure circumstances, rival strategies had been completely foxed by the reference-based method:
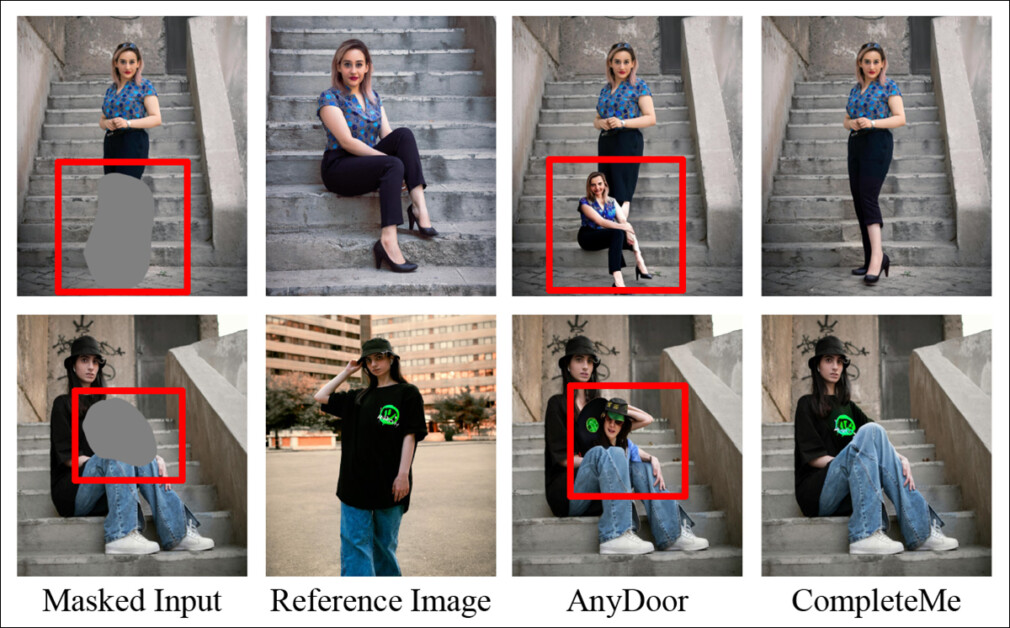
From the supplementary materials: the AnyDoor technique has specific problem deciding easy methods to interpret a reference picture.
The paper states:
‘In depth experiments on our benchmark display that CompleteMe outperforms state-of-the-art strategies, each reference-based and non-reference-based, by way of quantitative metrics, qualitative outcomes and person research.
‘Significantly in difficult situations involving complicated poses, intricate clothes patterns, and distinctive equipment, our mannequin persistently achieves superior visible constancy and semantic coherence.’
Sadly, the challenge’s GitHub presence comprises no code, nor guarantees any, and the initiative, which additionally has a modest challenge web page, appears framed as a proprietary structure.

Additional instance of the brand new system’s subjective efficiency towards prior strategies. Extra particulars later within the article.
Methodology
The CompleteMe framework is underpinned by a Reference U-Internet, which handles the combination of the ancillary materials into the method, and a cohesive U-Internet, which accommodates a wider vary of processes for acquiring the ultimate end result, as illustrated within the conceptual schema beneath:
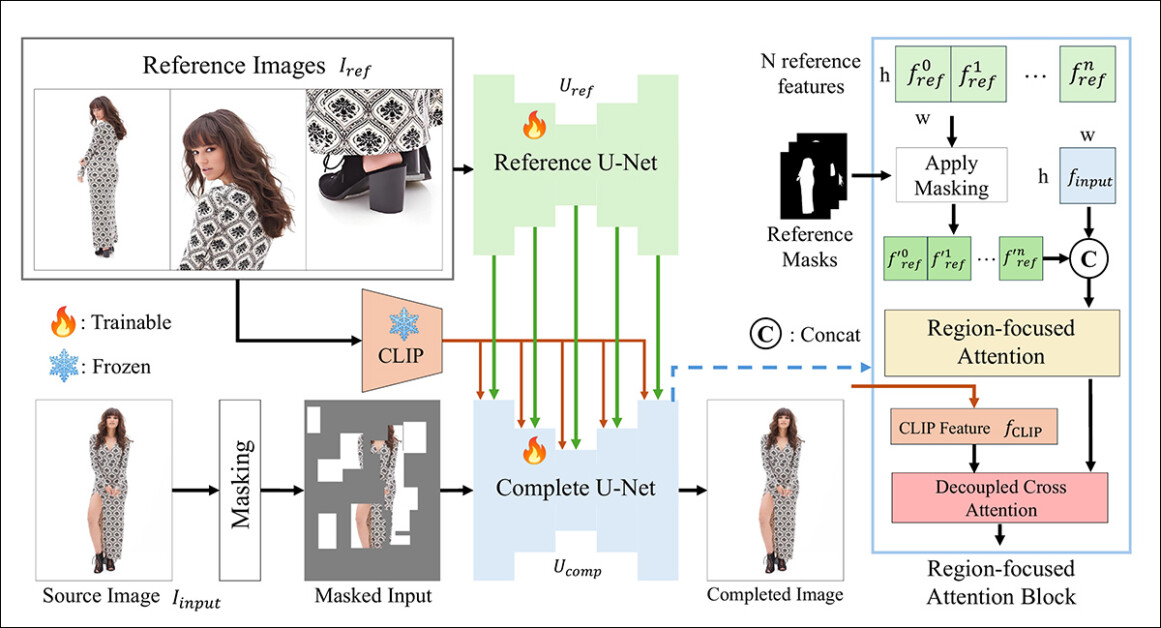
The conceptual schema for CompleteMe. Supply: https://arxiv.org/pdf/2504.20042
The system first encodes the masked enter picture right into a latent illustration. On the similar time, the Reference U-Internet processes a number of reference photos – every displaying completely different physique areas – to extract detailed spatial options.
These options move via a Area-focused Consideration block embedded within the ‘full’ U-Internet, the place they’re selectively masked utilizing corresponding area masks, making certain the mannequin attends solely to related areas within the reference photos.
The masked options are then built-in with international CLIP-derived semantic options via decoupled cross-attention, permitting the mannequin to reconstruct lacking content material with each nice element and semantic coherence.
To boost realism and robustness, the enter masking course of combines random grid-based occlusions with human physique form masks, every utilized with equal likelihood, rising the complexity of the lacking areas that the mannequin should full.
For Reference Solely
Earlier strategies for reference-based picture inpainting usually relied on semantic-level encoders. Initiatives of this type embody CLIP itself, and DINOv2, each of which extract international options from reference photos, however usually lose the nice spatial particulars wanted for correct identification preservation.
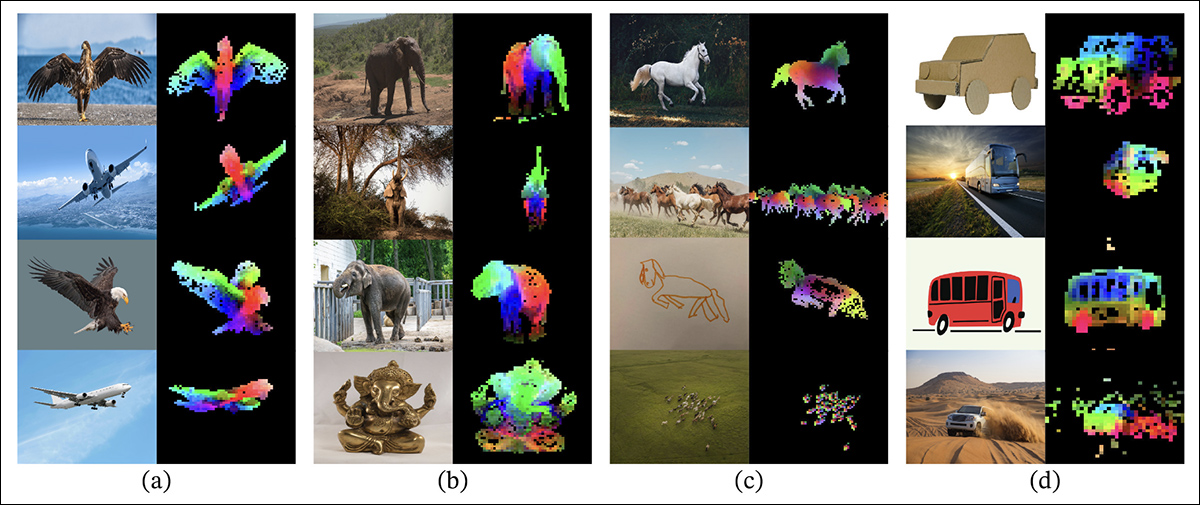
From the discharge paper for the older DINOV2 method, which is included compared exams within the new research: The coloured overlays present the primary three principal elements from Principal Part Evaluation (PCA), utilized to picture patches inside every column, highlighting how DINOv2 teams comparable object components collectively throughout diverse photos. Regardless of variations in pose, model, or rendering, corresponding areas (like wings, limbs, or wheels) are persistently matched, illustrating the mannequin’s capability to be taught part-based construction with out supervision. Supply: https://arxiv.org/pdf/2304.07193
CompleteMe addresses this side via a specialised Reference U-Internet initialized from Secure Diffusion 1.5, however working with out the diffusion noise step*.
Every reference picture, protecting completely different physique areas, is encoded into detailed latent options via this U-Internet. International semantic options are additionally extracted individually utilizing CLIP, and each units of options are cached for environment friendly use throughout attention-based integration. Thus, the system can accommodate a number of reference inputs flexibly, whereas preserving fine-grained look data.
Orchestration
The cohesive U-Internet manages the ultimate phases of the completion course of. Tailored from the inpainting variant of Secure Diffusion 1.5, it takes as enter the masked supply picture in latent type, alongside detailed spatial options drawn from the reference photos and international semantic options extracted by the CLIP encoder.
These numerous inputs are introduced collectively via the RFA block, which performs a essential function in steering the mannequin’s focus towards probably the most related areas of the reference materials.
Earlier than coming into the eye mechanism, the reference options are explicitly masked to take away unrelated areas after which concatenated with the latent illustration of the supply picture, making certain that spotlight is directed as exactly as potential.
To boost this integration, CompleteMe incorporates a decoupled cross-attention mechanism tailored from the IP-Adapter framework:
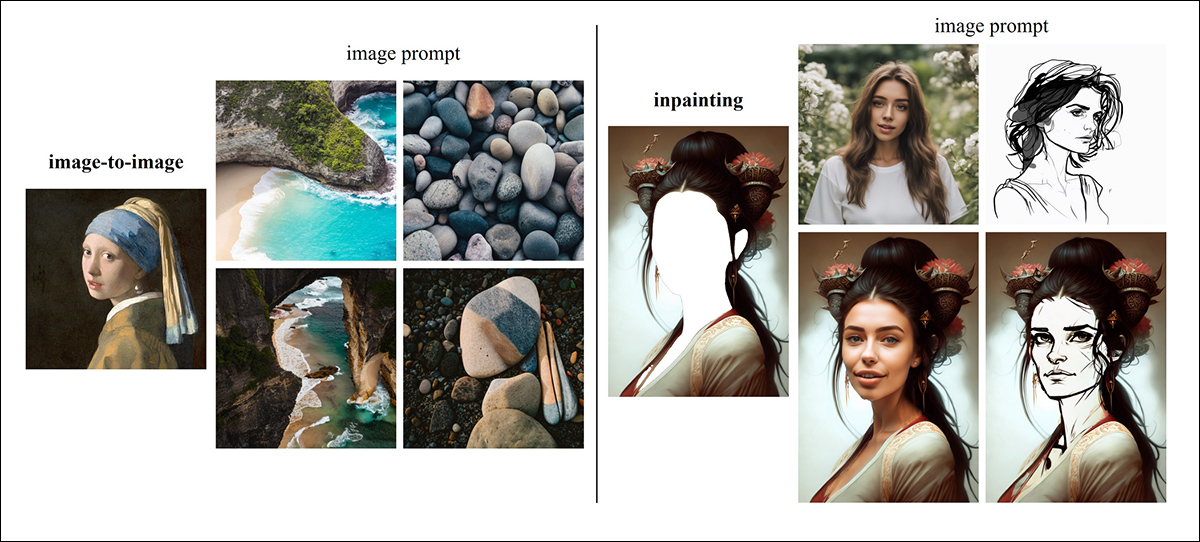
IP-Adapter, a part of which is integrated into CompleteMe, is likely one of the most profitable and often-leveraged initiatives from the final three tumultuous years of improvement in latent diffusion mannequin architectures. Supply: https://ip-adapter.github.io/
This enables the mannequin to course of spatially detailed visible options and broader semantic context via separate consideration streams, that are later mixed, leading to a coherent reconstruction that, the authors contend, preserves each identification and fine-grained element.
Benchmarking
Within the absence of an apposite dataset for reference-based human completion, the researchers have proposed their very own. The (unnamed) benchmark was constructed by curating choose picture pairs from the WPose dataset devised for Adobe Analysis’s 2023 UniHuman challenge.
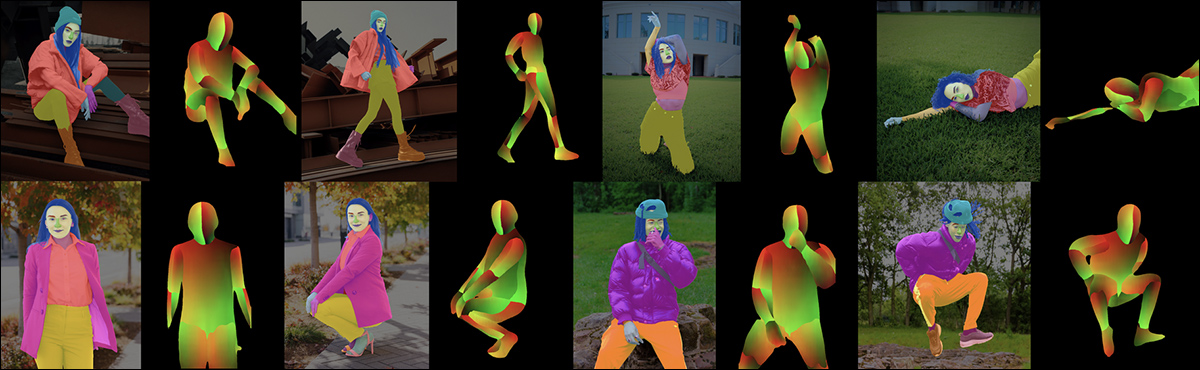
Examples of poses from the Adobe Analysis 2023 UniHuman challenge. Supply: https://github.com/adobe-research/UniHuman?tab=readme-ov-file#data-prep
The researchers manually drew supply masks to point the inpainting areas, finally acquiring 417 tripartite picture teams constituting a supply picture, masks, and reference picture.
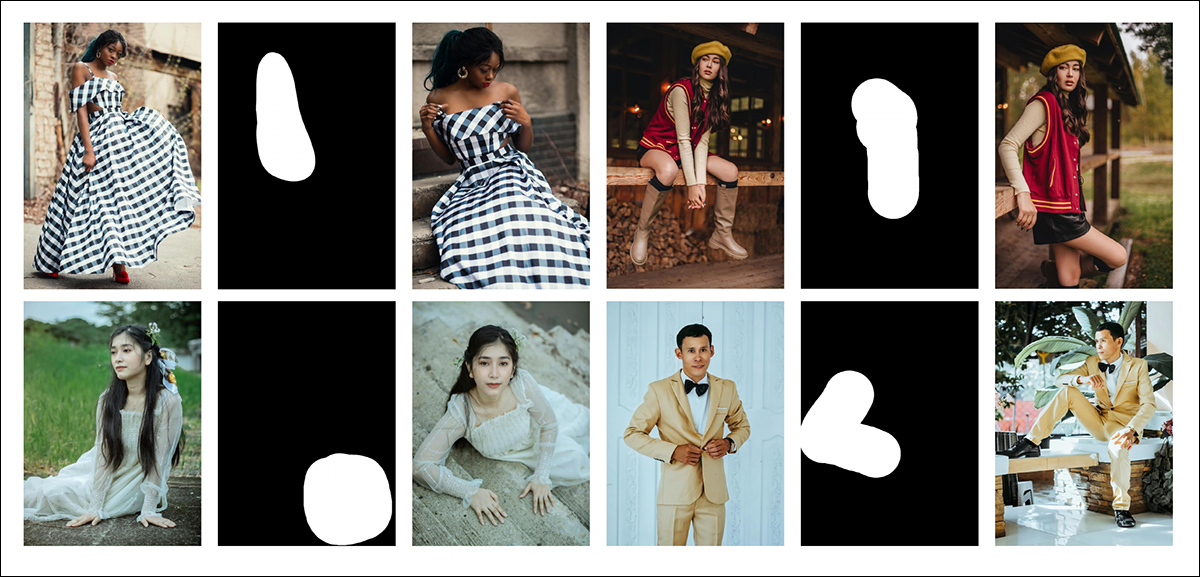
Two examples of teams derived initially from the reference WPose dataset, and curated extensively by the researchers of the brand new paper.
The authors used the LLaVA Massive Language Mannequin (LLM) to generate textual content prompts describing the supply photos.
Metrics used had been extra intensive than normal; moreover the standard Peak Sign-to-Noise Ratio (PSNR), Structural Similarity Index (SSIM) and Discovered Perceptual Picture Patch Similarity (LPIPS, on this case for evaluating masked areas), the researchers used DINO for similarity scores; DreamSim for era end result analysis; and CLIP.
Information and Exams
To check the work, the authors utilized each the default Secure Diffusion V1.5 mannequin and the 1.5 inpainting mannequin. The system’s picture encoder used the CLIP Imaginative and prescient mannequin, along with projection layers – modest neural networks that reshape or align the CLIP outputs to match the interior function dimensions utilized by the mannequin.
Coaching befell for 30,000 iterations over eight NVIDIA A100† GPUs, supervised by Imply Squared Error (MSE) loss, at a batch dimension of 64 and a studying price of two×10-5. Numerous parts had been randomly dropped all through coaching, to forestall the system overfitting on the information.
The dataset was modified from the Components to Entire dataset, itself based mostly on the DeepFashion-MultiModal dataset.
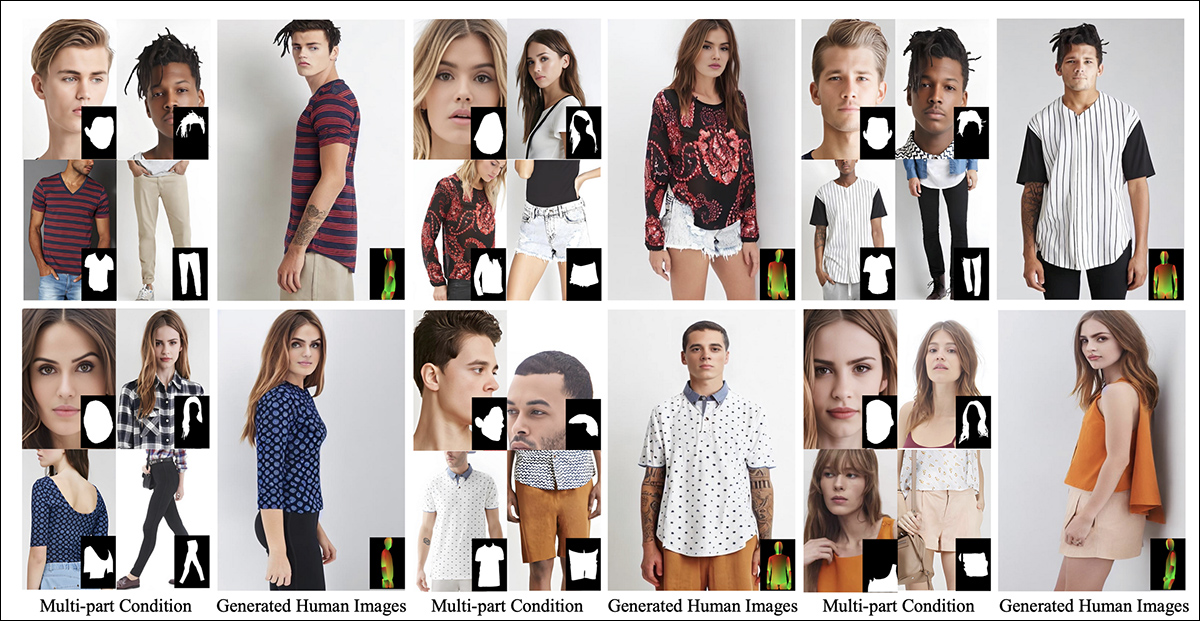
Examples from the Components to Entire dataset, used within the improvement of the curated knowledge for CompleteMe. Supply: https://huanngzh.github.io/Parts2Whole/
The authors state:
‘To satisfy our necessities, we [rebuilt] the coaching pairs by utilizing occluded photos with a number of reference photos that seize numerous features of human look together with their brief textual labels.
‘Every pattern in our coaching knowledge consists of six look sorts: higher physique garments, decrease physique garments, entire physique garments, hair or headwear, face, and sneakers. For the masking technique, we apply 50% random grid masking between 1 to 30 occasions, whereas for the opposite 50%, we use a human physique form masks to extend masking complexity.
‘After the development pipeline, we obtained 40,000 picture pairs for coaching.’
Rival prior non-reference strategies examined had been Massive occluded human picture completion (LOHC) and the plug-and-play picture inpainting mannequin BrushNet; reference-based fashions examined had been Paint-by-Instance; AnyDoor; LeftRefill; and MimicBrush.
The authors started with a quantitative comparability on the previously-stated metrics:
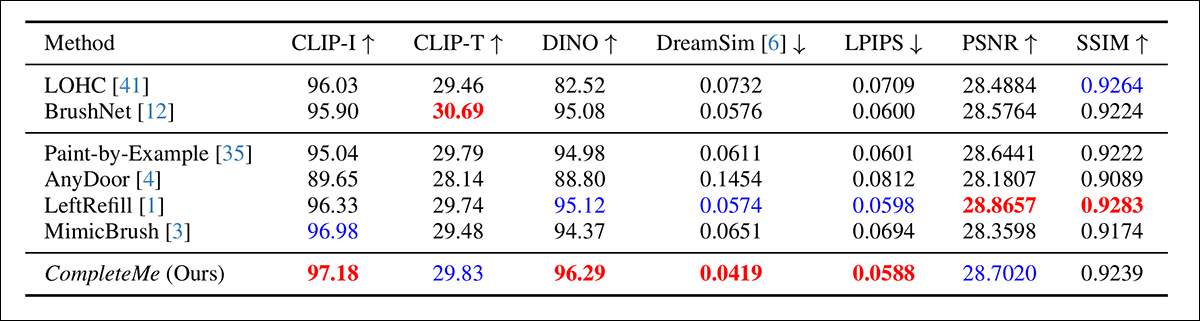
Outcomes for the preliminary quantitative comparability.
Relating to the quantitative analysis, the authors word that CompleteMe achieves the best scores on most perceptual metrics, together with CLIP-I, DINO, DreamSim, and LPIPS, that are meant to seize semantic alignment and look constancy between the output and the reference picture.
Nonetheless, the mannequin doesn’t outperform all baselines throughout the board. Notably, BrushNet scores highest on CLIP-T, LeftRefill leads in SSIM and PSNR, and MimicBrush barely outperforms on CLIP-I.
Whereas CompleteMe exhibits persistently sturdy outcomes total, the efficiency variations are modest in some circumstances, and sure metrics stay led by competing prior strategies. Maybe not unfairly, the authors body these outcomes as proof of CompleteMe’s balanced energy throughout each structural and perceptual dimensions.
Illustrations for the qualitative exams undertaken for the research are far too quite a few to breed right here, and we refer the reader not solely to the supply paper, however to the intensive supplementary PDF, which comprises many further qualitative examples.
We spotlight the first qualitative examples offered in the principle paper, together with a collection of further circumstances drawn from the supplementary picture pool launched earlier on this article:
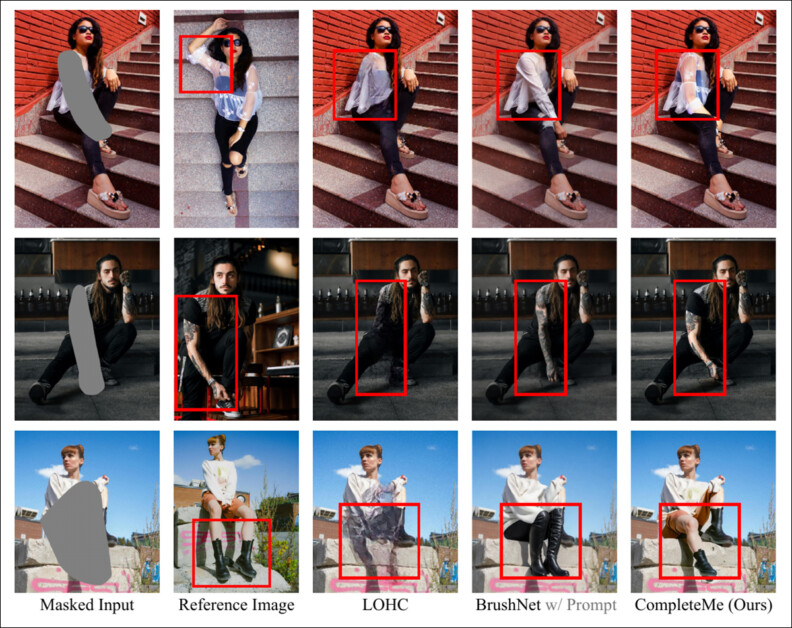
Preliminary qualitative outcomes offered in the principle paper. Please check with the supply paper for higher decision.
Of the qualitative outcomes displayed above, the authors remark:
‘Given masked inputs, these non-reference strategies generate believable content material for the masked areas utilizing picture priors or textual content prompts.
‘Nonetheless, as indicated within the Purple field, they can not reproduce particular particulars akin to tattoos or distinctive clothes patterns, as they lack reference photos to information the reconstruction of similar data.’
A second comparability, a part of which is proven beneath, focuses on the 4 reference-based strategies Paint-by-Instance, AnyDoor, LeftRefill, and MimicBrush. Right here just one reference picture and a textual content immediate had been supplied.
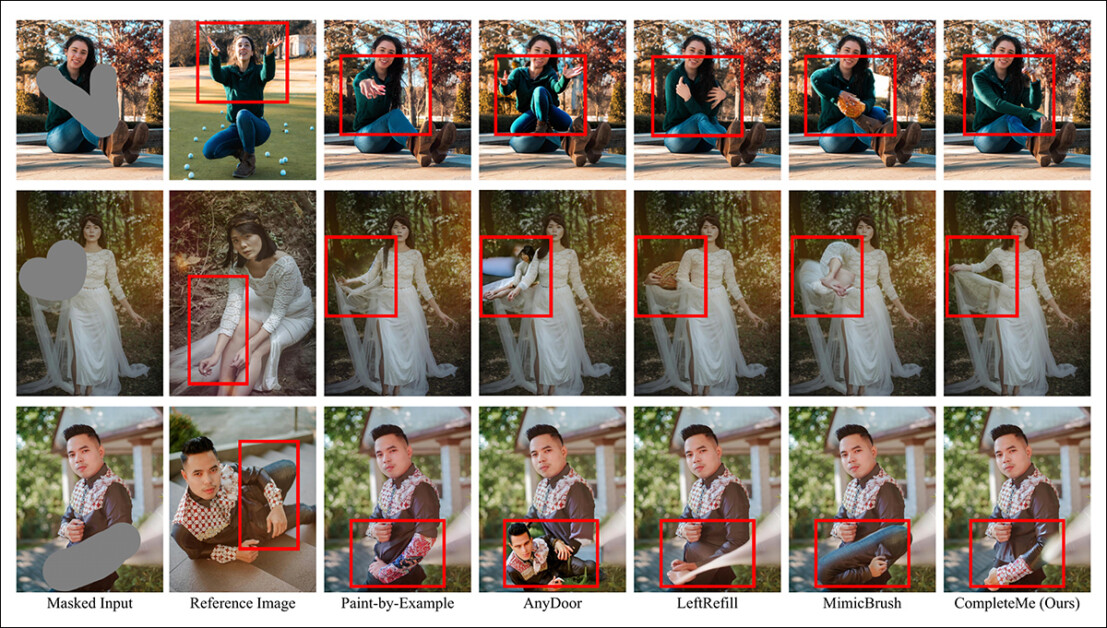
Qualitative comparability with reference-based strategies. CompleteMe produces extra lifelike completions and higher preserves particular particulars from the reference picture. The crimson packing containers spotlight areas of specific curiosity.
The authors state:
‘Given a masked human picture and a reference picture, different strategies can generate believable content material however usually fail to protect contextual data from the reference precisely.
‘In some circumstances, they generate irrelevant content material or incorrectly map corresponding components from the reference picture. In distinction, CompleteMe successfully completes the masked area by precisely preserving similar data and accurately mapping corresponding components of the human physique from the reference picture.’
To evaluate how nicely the fashions align with human notion, the authors performed a person research involving 15 annotators and a couple of,895 pattern pairs. Every pair in contrast the output of CompleteMe towards one among 4 reference-based baselines: Paint-by-Instance, AnyDoor, LeftRefill, or MimicBrush.
Annotators evaluated every end result based mostly on the visible high quality of the finished area and the extent to which it preserved identification options from the reference – and right here, evaluating total high quality and identification, CompleteMe obtained a extra definitive end result:
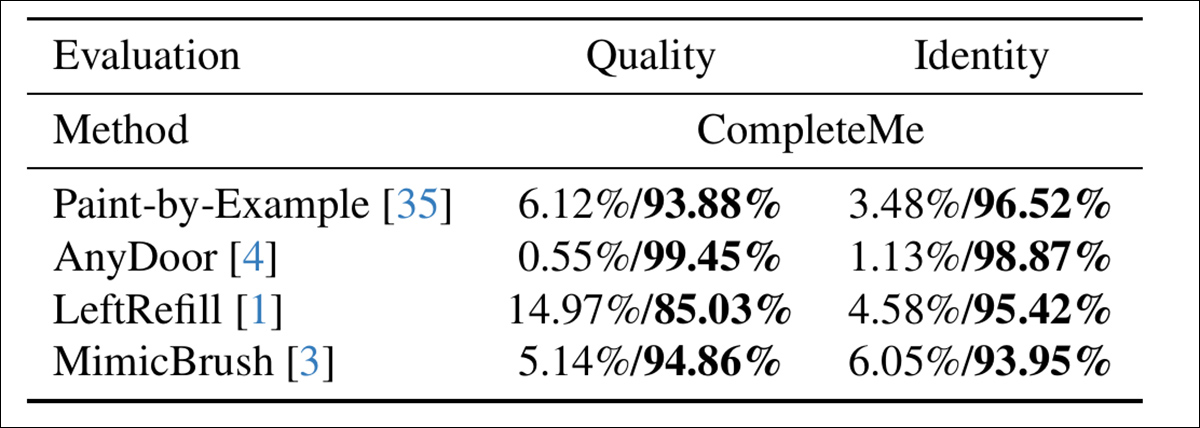
Outcomes of the person research.
Conclusion
If something, the qualitative outcomes on this research are undermined by their sheer quantity, since shut examination signifies that the brand new system is a simplest entry on this comparatively area of interest however hotly-pursued space of neural picture modifying.
Nonetheless, it takes a little bit further care and zooming-in on the unique PDF to understand how nicely the system adapts the reference materials to the occluded space compared (in practically all circumstances) to prior strategies.
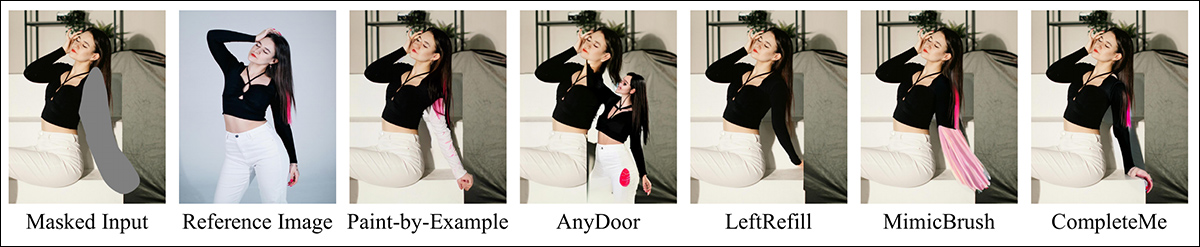
We strongly advocate the reader to fastidiously look at the initially complicated, if not overwhelming avalanche of outcomes offered within the supplementary materials.
* It’s fascinating to notice how the now severely-outmoded V1.5 launch stays a researchers’ favourite – partly because of legacy like-on-like testing, but additionally as a result of it’s the least censored and probably most simply trainable of all of the Secure Diffusion iterations, and doesn’t share the censorious hobbling of the FOSS Flux releases.
† VRAM spec not given – it will be both 40GB or 80GB per card.
First printed Tuesday, April 29, 2025