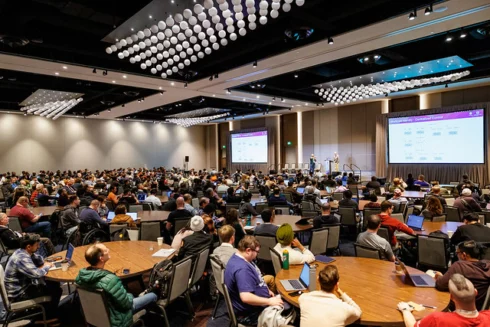
I used to be a visitor of the Cloud Native Computing Basis (CNCF) at its EU KubeCon convention in London the primary week of April. Most of my conversations with the distributors on the occasion may be grouped below three fundamental themes: multi-cluster administration, AI workloads, and lowering Kubernetes prices on the cloud.
Multi-cluster administration
Working Kubernetes clusters turns into a problem as soon as the variety of clusters grows unwieldy. In massive enterprises with purposes operating 100s of clusters at scale, there’s a want for multi-cluster administration (additionally known as fleet administration), which is quick turning into a spotlight within the cloud native vendor group. These options present a unified dashboard to handle clusters throughout multi-cloud environments, private and non-private, offering visibility into what can flip into cluster sprawl, and apply FinOps to optimize prices. They assist DevOps groups handle scalability and workloads, in addition to play a component in excessive availability and catastrophe restoration by replicating workloads throughout clusters in several areas, for instance. DevOps CI/CD and platform engineering develop into important to handle massive numbers of clusters.
I spoke with a number of distributors at KubeCon who’re addressing this problem, e.g., SUSE is launching a Rancher multi-cluster administration characteristic for EKS.
Mirantis can also be tuning into this pattern seeing cluster progress throughout distributed programs on the edge, regulatory management with want for sovereign cloud and separation of knowledge, and hybrid cloud, all main to higher multi-cluster administration. To deal with this Mirantis launched k0rdent in Feb 2025, an open supply Kubernetes-native distributed container administration answer that may run on public clouds, on-premises, and on the edge, providing unified administration for a number of Kubernetes clusters. Some k0rdent key options are declarative configuration making it simple to scale out, observability with FinOps to manage prices, and a providers supervisor to allow providers constructed on high of the answer. Mirantis acknowledges how Kubernetes has matured to develop into a de facto cloud native normal throughout multi-cloud environments which permit the cloud agnostic options from Mirantis to offer portability throughout a number of environments.
Mirantis’s dedication to open supply was bolstered with its k0s (edge) Kubernetes and k0smotron multi-cluster administration device becoming a member of the CNCF Sandbox initiatives. k0rdent is constructed on high of those basis initiatives and goes past the essential cluster administration in K0smotron.
Amazon EKS Hybrid Nodes launched at AWS re:Invent 2024 permits current on-premises and edge infrastructure for use as nodes in Amazon EKS clusters, unifying Kubernetes administration throughout totally different environments. This companions with Amazon EKS Anyplace which is designed for disconnected environments, whereas with EKS Hybrid Nodes it’s potential to have connectivity and a completely managed Kubernetes management aircraft throughout all environments. A use case is enabling prospects to reinforce their AWS GPU capability with preexisting GPU investments on-premises.
So, AWS’s edge choices: EKS Anyplace is absolutely disconnected from the cloud and the Kubernetes management aircraft is managed by the shopper; EKS Hybrid Node provides on-premises infrastructure and the Kubernetes management aircraft is managed by AWS; lastly, AWS Outposts has the management aircraft and the infrastructure all managed by AWS.
I spoke with Kevin Wang, lead of cloud native open supply crew at Huawei, co-founder of a number of CNCF initiatives: KubeEdge, Karmada, and Volcano. Kevin identified that Huawei has been contributing to Kubernetes from its earliest days and that it’s imaginative and prescient has at all times been to work with open requirements. Karmada (incubating CNCF challenge) is an open, multi-cloud, multi-cluster Kubernetes orchestration system for operating cloud native purposes throughout a number of Kubernetes clusters and clouds. Key options embrace centralized multi-cloud administration, excessive availability, failure restoration, and site visitors scheduling. Instance use circumstances of Karmada embrace journey.com which used Karmada to construct a management aircraft for a hybrid multi-cloud, lowering migration prices throughout heterogeneous environments, and Australian Institute for Machine Studying makes use of Karmada to handle edge clusters alongside GPU-enabled clusters, guaranteeing compatibility with numerous compute sources.
VMware’s answer for multi-cluster Kubernetes environments has been re-branded VMware vSphere Kubernetes Service (VKS), previously often called VMware Tanzu Kubernetes Grid (TKG) Service, which is a core part of the VMware Cloud Basis. VMware provides two approaches to operating cloud native workloads: by way of Kubernetes and by way of Cloud Foundry. Maybe confusingly, Cloud Foundry has the Korifi challenge which gives a Cloud Foundry expertise on high of Kubernetes and which additionally underpins VMware Tanzu Platform for Cloud Foundry. The purpose of VMware providing two strands, is that the Kubernetes expertise is for DevOps/platform engineers aware of that eco-system, whereas the Cloud Foundry expertise is extra opinionated however with a consumer pleasant interface.
I met with startup Spectro Cloud, launched in 2020 and now 250 robust, it was co-founded by serial tech entrepreneurs. Spectro Cloud provides an enterprise-grade Kubernetes administration platform known as Palette, for simplifying at scale the total lifecycle of Kubernetes clusters throughout numerous environments: public clouds, personal clouds, naked metallic, and edge places. Key options are: declarative multi-cluster Kubernetes administration, and a unified platform for containers, VMs, and edge AI. Palette EdgeAI provides a light-weight Kubernetes optimized for AI workloads. Customers can handle hundreds of clusters with Palette, which is decentralized so there are not any pricey administration servers or regional situations, Palette enforces every cluster coverage regionally. To handle hundreds of clusters Palette operates not within the Kubernetes management aircraft, however in a administration aircraft that sits above it. On the sting Spectro Cloud leverages CNCF challenge Kairos. Kairos transforms current Linux distributions into immutable, safe, and declaratively managed OS photographs which might be optimized for cloud-native infrastructure.
Palette lets customers select over 50 better of breed elements when deploying stacks, from Kubernetes distributions to CI/CD instruments and repair meshes and these packs are validated and supported for compatibility. Containers and VMs are supported out-of-the-box with little consumer configuration. Palette makes use of a personalized model of the open supply Kubernetes, named Palette eXtended Kubernetes, as default, however Spectro Cloud helps a number of widespread Kubernetes distros (RKE2, k3s, microk8s, cloud-managed providers), and prospects don’t have to configure these on their very own. Moreover, Spectro Cloud factors out it’s distro-agnostic, adopting distros primarily based on buyer demand. With half of Spectro Cloud’s enterprise coming from the sting, it’s making edge computing extra practicable for AI workloads.
AI workloads and the important thing position of the Mannequin Context Protocol
AI workloads will develop to develop into a significant a part of the compute site visitors in an enterprise, and the cloud native group is popping to creating this transition as seamless as potential. A problem is easy methods to navigate the complexities of connecting a number of AI brokers with different instruments and programs. There’s a want for device discovery and integration, a unified registry, the problem of connectivity and multiplexing, and safety and governance.
Anthropic created and open sourced a normal for AI brokers to find and work together with exterior instruments by defining how they describe their capabilities and the way brokers can invoke them, known as Mannequin Context Protocol (MCP).
Solo.io, a cloud native vendor, introduced at KubeCon their evolution of MCP known as MCP Gateway, which is constructed on their API gateway known as kgateway (previously Gloo). With instruments adopting this normal, MCP Gateway gives a centralized level for integrating and governing AI brokers throughout toolchains. MCP Gateway virtualizes a number of MCP instruments and servers right into a unified, safe entry layer, offering AI builders with a single endpoint to work together with a variety of instruments, significantly simplifying and aiding agentic AI software improvement. Further key options embrace: automated discovery and registration of MCP device servers; a central registry of MCP instruments throughout numerous environments; MCP multiplexing, permitting entry to a number of MCP instruments by way of a single endpoint; enhanced safety with the MCP Gateway offering authentication and authorization controls, and guaranteeing safe interplay between AI brokers and instruments; improved observability of AI agent and instruments efficiency by way of centralized metrics, logging, and tracing.
Moreover, Solo.io sees MCP Gateway as laying the inspiration for an agent mesh, an infrastructure layer for networking throughout AI brokers, corresponding to agent-to-LLM, agent-to-tool, and agent-to-agent communication.
Persevering with on the theme of AI safety , working with enterprise AI purposes carries two vital dangers: first, compliance with laws within the native jurisdiction, for instance within the EU with GDPR and the EU AI Act. And second, easy methods to deal with firm confidential knowledge, for instance placing delicate knowledge in a SaaS primarily based AI software places that knowledge out on the cloud and leaves the potential for it to leak out.
One method to lowering these dangers is taken by SUSE, its SUSE AI is a safe, personal, and enterprise-grade AI platform for deploying generative AI (genAI) purposes. Delivered as a modular answer, customers can use the options they want and in addition prolong it. This scalable platform additionally gives the insights prospects have to run and optimize their genAI apps.
Huawei is concerned within the CNCF initiatives to handle AI workloads, corresponding to Kubeflow. Kubeflow began out as a machine studying lifecycle administration system, orchestrating the pipeline for ML workloads throughout the lifecycle, from improvement by way of to manufacturing. It has since advanced to handle LLM workloads, leveraging Kubernetes for distributed coaching throughout massive clusters of GPUs, offering fault tolerance, and managing inter-process communications. Different options embrace mannequin serving at scale with KServe (initially developed as a part of the KFServing challenge inside Kubeflow, KServe is within the Linux AI Basis however there may be speak of transferring it into CNCF), providing autoscaling of AI site visitors hundreds, and performing optimization corresponding to mannequin weight quantization that reduces reminiscence footprint and enhances pace. Huawei can also be a co-founder of the Volcano challenge for batch scheduling AI workloads throughout a number of pods contemplating inter-dependencies, in order that workloads are scheduled within the right order.
long term analysis, Huawei is engaged on how AI workloads work together in manufacturing, with purposes operating on the edge and in robots, and the way machines talk with people and with different machines, and the way this scales, for instance, throughout a fleet of robots working in a warehouse for route planning and collision avoidance. This work falls throughout the scope of KubeEdge (incubating CNCF challenge), an open supply edge computing framework for extending Kubernetes to edge gadgets, addressing the challenges of useful resource constraints, intermittent connectivity, and distributed infrastructure. Part of this analysis falls below Sedna, an “edge-cloud synergy AI challenge” operating inside KubeEdge. Sedna allows collaborative coaching and inference, integrating seamlessly with current AI instruments corresponding to TensorFlow, PyTorch, PaddlePaddle, and MindSpore.
Pink Hat is exploiting AI in its instruments, for instance it launched model 0.1 of Konveyor AI for utilizing LLMs and static code evaluation to assist upgrading current/legacy purposes and is a part of Konveyor (a sandbox CNCF challenge), an accelerator for the modernization and migration of legacy purposes to Kubernetes and cloud-native environments. Within the Pink Hat OpenShift console there may be now a digital AI assistant known as OpenShift Lightspeed for customers to work together with OpenShift utilizing pure language, and it’s skilled on the consumer’s knowledge, so it has correct context. To assist AI workloads, there may be OpenShift AI for creating, deploying, and managing AI workloads throughout hybrid cloud environments.
VMware is supporting AI workloads on the infrastructure layer with VMware Non-public AI Basis (constructed on VMware Cloud Basis, the VMware personal cloud), guaranteeing databases for RAG and storage can be found, but additionally rolling up all of the elements which might be wanted for operating AI workloads on Kubernetes, automating the deployment, making it simple for customers. This providing is in partnership with Nvidia and consists of its NeMo framework, for constructing, fine-tuning, and deploying generative AI fashions, and helps NVIDIA GPUs and NVIDIA NIM for optimized inference on a variety of LLMs.
Managing Kubernetes prices on the cloud
Zesty, a startup launched in 2019, has discovered methods of lowering prices operating Kubernetes on the cloud, making use of Kubernetes’s connections to the cloud supplier. As soon as put in in a cluster, Zesty Kompass can carry out pod right-sizing, the place it tracks the CPU, reminiscence, server, storage quantity utilization and dynamically adjusts these, up or all the way down to the wants of the workloads. Zesty finds customers provision an excessive amount of capability for the necessity of the workloads truly run and adjusting these capacities just isn’t simple to carry out dynamically. Most corporations hold a buffer of servers in readiness for spike calls for, so Zesty places these extra servers into hibernation, which reduces the price of retaining these servers significantly decrease. Zesty Kompass may also assist customers exploit spot situations on their chosen cloud. The answer runs inside a cluster to keep up one of the best safety stage, and usually a number of clusters are deployed to keep up segregation, nonetheless, by putting in Kompass in a number of clusters, its dashboard gives a worldwide view of Kompass exercise inside every cluster it’s deployed in. Most not too long ago Zesty introduced that Kompass now consists of full pod scaling capabilities, with the addition of Vertical Pod Autoscaler (VPA) alongside the prevailing Horizontal Pod Autoscaler (HPA).
Amazon EKS Auto Mode (launched at AWS re:Invent 2024) is constructed on open supply challenge Karpenter. Karpenter manages a node lifecycle inside Kubernetes, lowering prices by robotically provisioning nodes (up and down) primarily based on scheduling wants of pods. When deploying workloads the consumer specifies the scheduling constraints within the pod specs, Karpenter makes use of these to handle provisioning. With EKS Auto Mode, administration of Kubernetes clusters is simplified, letting AWS handle cluster infrastructure, corresponding to compute autoscaling, pod and repair networking, software load balancing, cluster DNS, block storage, and GPU assist. Auto Mode additionally leverages EC2 managed situations, which allows EKS to tackle the shared accountability possession and safety of the cluster compute the place purposes have to run.
Speaking with the AWS crew at KubeCon it emerged that AWS is the host cloud for the Kubernetes challenge at CNCF, which it provides without charge to CNCF, so a pleasant contribution to open supply from Amazon.
Launched in 2019, LoftLabs is the seller that introduced digital clusters to Kubernetes, the corporate is now 60 robust. With digital clusters organizations can run fewer bodily clusters and inside a cluster using digital clusters creates higher administration of crew sources than namespaces. A current press launch on its buyer, Aussie Broadband, says that improvement groups might deploy clusters on-demand in below 45 secs. The shopper estimates it saved 2.4k hours of dev time per 12 months and £180k discount in provisioning prices per 12 months. At KubeCon it launched a brand new product, vNode, which gives a extra granular isolation of workloads operating inside vClusters. This method enhances multi-tenancy by way of improved useful resource allocation and isolation throughout the virtualized environments. Since a digital node is mapped to a non-privileged consumer, privileged workloads are remoted and may entry sources corresponding to storage which might be out there on the digital cluster.
Cloud Native Buildpacks provide improved safety
I spoke with the Cloud Foundry crew, which talked about that its CI/CD device, Concourse, has joined CNCF initiatives, and that Cloud Foundry is a distinguished adopter of Cloud Native Buildpacks, which it described because the hidden gem inside CNCF. Buildpacks rework software supply code into container photographs, together with all the required dependencies. An instance utilized by Kubernetes is kpack, and a bonus is that they cast off the necessity for Dockerfiles. Whereas Docker was transformational within the evolution of cloud native computing, it isn’t open supply, which creates an anomaly inside CNCF. Provide chain safety just isn’t handled in Dockerfiles, and there’s a rising demand for larger transparency and openness in order to cut back safety dangers. Buildpacks have been evolving to deal with these safety considerations, with for instance a software program invoice of supplies. Buildpacks had been first conceived by Heroku in 2011, adopted by Cloud Foundry and others, after which the open supply Cloud Native Buildpacks challenge joined CNCF in 2018, with graduate standing anticipated in 2026.
Observability firm Dash0 was based in 2023 by CEO Mirko Novakovic, to carry out tracing, logging, metrics and alerts, and whose earlier observability firm, Instana, was offered to IBM in 2002. Dash0 is constructed from the bottom up across the OpenTelemetry normal, this implies there isn’t a vendor lock-in of the telemetry knowledge, which stays in an open, standardized format. It makes use of OpenTelemetry’s semantic conventions so as to add context to knowledge, and it helps the OpenTelemetry’s collector, a central level for receiving, processing and forwarding telemetry knowledge. Designed to make the developer expertise with observability simple, it has value transparency, and a telemetry spam filter the place logs, traces and metrics that aren’t wanted are eliminated. Mirko’s method is that since you’re in search of a needle in a haystack, first make the haystack as small as potential, and that is the place AI is used.
The search house is decreased by not inspecting logs which have already been processed and present regular habits. Then Dash0 makes use of an LLM primarily based AI to reinforce the info by structuring it, after which it’ll acknowledge error codes and drill down additional to triage the error supply and determine its potential origins. Mirko doesn’t name this root-cause-analysis, as a result of this time period has been overused and resulted in lack of confidence because of false positives. As an alternative Dash0’s triage characteristic will give the most certainly explanation for the error as its first alternative, but additionally present possible alternate options, this implies the developer has materials to seek out and isolate the foundation trigger.
Dash0 finds basis LLMs may be correct with out requiring extra finetuning or Retrieval Augmented Technology and makes use of multiple LLM to cross examine outcomes and scale back hallucinations.
I spoke with Benjamin Brial, CEO and founding father of Cycloid, which gives a Kubernetes sustainable platform engineering answer to streamline DevOps, hybrid/multi-cloud adoption, and software program supply. It has established enterprise purchasers like Orange Enterprise Companies, Siemens, Valiantys, and Resort Spider, and contributes to open-source with instruments like TerraCognita and InfraMap. Digital sovereignty and sustainability are two key missions for the corporate, which operates within the EU and North America. It reduces prices by presenting to the developer solely the instruments/options they want. Cycloid emphasizes sustainability by way of FinOps and GreenOps. It provides a centralized view of cloud prices throughout suppliers in a single panel, and it tracks cloud carbon footprint to attenuate environmental impression, addressing cloud useful resource waste. With digital sovereignty turning into extra vital within the present geopolitical local weather, Cycloid with its base in Paris, leverages its European roots to deal with regional sovereignty considerations, partnering with native and world gamers like Orange Enterprise Companies and Arrow Electronics to ship options tailor-made to the European market.
Cycloid makes use of a plugin framework to combine any third-party device. It additionally embeds open supply instruments in its answer corresponding to TerraCognita (for importing infrastructure into IaC), TerraCost (for value estimation) and InfraMap (for visualizing infrastructure). These instruments allow organizations to reverse engineer and handle their infrastructure with out dependency on proprietary programs, a key side of digital sovereignty. Cycloid provides enterprises the liberty to pick out the precise instruments for every course of, keep self-hosted options, and embed any form of automation corresponding to Terraform, Ansible, and Helm to deploy IaaS, PaaS, or containers, which is vital for retaining management over knowledge and operations.