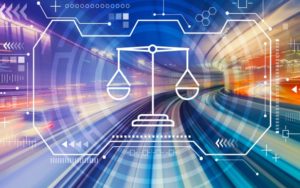
(TierneyMJ/ Shutterstock)
The standard, amount, and variety of coaching information have an amazing influence on generative AI (GenAI) mannequin efficiency. Components akin to mannequin structure, coaching strategies, and the complexity of the issues being solved additionally play essential roles. Nonetheless, the main mannequin builders are all zeroing in on information high quality, depth, and selection as the most important components figuring out AI mannequin efficiency and the most important alternative driving the subsequent rounds of enchancment.
Microsoft researchers defined the speedy enchancment within the efficiency of the most recent Phi language fashions by saying, “The innovation lies fully in our dataset for coaching.” The corporate’s Phi-3 mannequin coaching included extra information than with earlier fashions. We noticed an identical improvement with Meta’s Llama 3 fashions utilizing 15T token datasets. Nonetheless, Microsoft additionally careworn the good thing about “closely filtered net information.” When inaccuracies and biases are embedded in coaching information, AI-powered options usually tend to produce outputs inconsistent with actuality and introduce the next threat of exacerbating undesirable biases. Information high quality and curation matter.
Going Past a Guidelines
To mitigate the danger of inaccurate or biased outputs, organizations ought to leverage high-quality and various datasets which can be filtered and curated in alignment with their wants, company values, and governance frameworks. This includes utilizing people for what they do finest, producing and classifying long-tail info, and machines for his or her strengths in information filtering and curation at scale. People are significantly essential for growing and classifying coaching datasets which can be correct and consultant of the populations and situations the AI will serve, whereas machines are glorious at generalization. This mixture varieties the muse of high-performing giant language fashions (LLMs). This can be much more crucial as multimodal fashions develop into commonplace.
However builders can’t cease there. A number of different finest practices embody fine-tuning and steady monitoring of efficiency metrics, person suggestions and system logs. These steps are additionally crucial for detecting and mitigating the prevalence of hallucinations and biases. That is significantly essential as AI methods proceed evolving by making use of person information to enhance efficiency and alignment.
The answer to many of those challenges goes past a guidelines. Enterprises ought to undertake a system of checks and balances inside their AI expertise stack supported by a strong governance framework. That is additional enhanced by elevating worker consciousness and adoption throughout the enterprise to make sure they facilitate interactions which can be free from bias and dangerous content material and are dependable and correct.
Make use of Bias Detection and Mitigation Practices
At its core, in case your coaching datasets are too small or of low high quality, your LLM will perpetuate and amplify biases and inaccuracies. This will doubtlessly trigger vital hurt to people. Significantly in danger are underrepresented and marginalized communities akin to ethnic and racial minorities, LGBTQ+ people, folks with disabilities, and immigrants, amongst many others. This phenomenon could be most detrimental within the areas of regulation, training, employment, finance, and healthcare. As such, it’s essential that organizations make use of humans-in-the-loop (HITL) when evaluating GenAI software efficiency, conducting supervised fine-tuning (SFT), and interesting in immediate engineering to correctly information AI mannequin actions.
A key approach in AI mannequin coaching is reinforcement studying from human suggestions (RLHF). Since AI fashions lack a nuanced understanding of language and context, RLHF incorporates the real-world data of people into the coaching course of. For instance, RLHF can prepare GenAI to information mannequin responses to align with model preferences or social and cultural norms. That is particularly essential for firms working in a number of international markets the place understanding (and following) cultural nuances can outline success or failure.
Nevertheless it’s not nearly together with HITL. Success can be dependent upon partaking correctly certified, uniquely skilled, and various people to create, accumulate, annotate, and validate the info for high quality management. This method gives the dual advantages of upper high quality and threat mitigation.
Take into account an instance from healthcare. LLMs can be utilized to rapidly analyze textual content and picture information akin to digital well being information, radiology studies, medical literature, and affected person info to extract insights, make predictions, and help in scientific decision-making. Nonetheless, if the coaching information used was not appropriately various or there was an inadequate amount, sure biases would emerge. The state of affairs could be exacerbated if medical specialists usually are not included within the information and software output evaluation course of. Herein lies the danger. Failure to precisely establish ailments and account for variations amongst affected person populations can result in misdiagnosis and inappropriate remedies.
Implementing System Strategies
Generative AI options are proliferating. Meaning the necessity for correct and consultant information is extra essential than ever throughout all industries. The truth is, a survey by TELUS Worldwide, discovered that 40% of respondents imagine extra work by firms is required to guard customers from bias and false info, and 77% need manufacturers to audit their algorithms to mitigate bias and prejudice earlier than integrating GenAI expertise.
To forestall biases from getting into the earliest levels of LLM improvement, manufacturers can implement a multi-faceted method all through the event lifecycle. Along with various information assortment, implementing bias detection instruments, HITL evaluations and steady monitoring and iteration, manufacturers can incorporate countermeasures like adversarial examples in coaching to additional improve a platform’s skill to detect anomalies and reply appropriately.
For instance, a latest method that we’ve got taken includes integrating adversarial examples into coaching a Twin-LLM Security System for a retrieval augmented era (RAG) platform. This technique makes use of a secondary LLM, or Supervisor LLM, to categorize outputs in response to custom-made person expertise tips, introducing an extra layer of checks and balances to make sure accuracy and mitigate biases from the outset.
Constructing Layers to Mitigating Bias in GenAI Programs
Along with the abovementioned methods and practices, manufacturers can make use of strategies akin to information anonymization and augmentation to assist additional establish potential biases or inaccuracies and scale back their influence on GenAI methods’ outputs.
Information anonymization includes obscuring or eradicating personally identifiable info (PII) from datasets to guard people’ privateness. By anonymizing information, biases associated to demographic traits akin to race, gender, or age could be decreased because the system doesn’t have entry to express details about people’ identities. This, in flip, reduces the danger of biased selections or predictions primarily based on such attributes.
Past this, tooling akin to guardrails and supervisor LLMs can supply the flexibility to proactively establish issues as they come up. These instruments can allow firms to redact or rewrite problematic responses and log them to be used in subsequent mannequin coaching.
Information augmentation includes increasing the coaching dataset by creating new artificial examples to diversify the coaching dataset and improve the illustration of underrepresented teams and views. For instance, this might embody paraphrasing sentences or changing synonyms in textual content datasets or scaling, cropping and rotating pictures for picture information. By means of these strategies, the system learns from a broader vary of information to develop into extra strong, mitigating biases that will come up resulting from skewed or restricted datasets. Integrating these strategies into the info pre-processing pipeline may help construct extra inclusive and equitable GenAI methods.
Preserving Humanity within the Loop
Though no GenAI mannequin right now could be utterly free from hallucinations or bias, enterprise leaders should embed moral AI practices throughout their organizations and put money into bias-mitigation initiatives because the expertise continues to evolve. It’s an ongoing course of, but it surely’s crucial to defending their enterprise and the top customers and to responsibly advancing GenAI adoption.
In regards to the creator: Tobias Dengel is the President of TELUS Digital Options and founder and President of WillowTree, a TELUS Worldwide Firm. In his present position, Tobias is targeted on propelling the continued and profitable evolution of TELUS Worldwide to the subsequent frontier of expertise in CX. With over 20 years of expertise, he joined the corporate in January 2023 when WillowTree was acquired by TELUS Worldwide. Previous to his present position, Tobias held quite a lot of management roles together with Basic Supervisor of AOL Native and VP of AOL Worldwide, primarily based in London. He was the co-founder of Leads.com, a pioneering search company that was acquired by Internet.com in 2005.
Associated Gadgets:
Why Preserving People within the Loop Is Crucial for Reliable AI
Hallucinations, Plagiarism, and ChatGPT
Organizations Wrestle with AI Bias