Someplace, proper now, a expertise government tells their administrators: “we
want a approach to measure the productiveness of our engineering groups.” A working
group assembles to discover potential options, and weeks later, proposes
implementing the metrics: lead time, deployment frequency, and variety of
pull requests created per engineer.
Quickly after, senior engineering leaders meet to evaluation their newly created
dashboards. Instantly, questions and doubts are raised. One chief says:
“Our lead time is 2 days which is ‘low performing’ in keeping with these
benchmarks – however is there really an issue?”. One other chief says: “it’s
unsurprising to see that a few of our groups are deploying much less usually than
others. However I’m undecided if this spells a chance for enchancment.”
If this story arc is acquainted to you, don’t fear – it is acquainted to
most, together with among the greatest tech firms on this planet. It’s not unusual
for measurement applications to fall quick when metrics like DORA fail to supply
the insights leaders had hoped for.
There may be, nevertheless, a greater method. An method that focuses on
capturing insights from builders themselves, relatively than solely counting on
primary measures of velocity and output. We’ve helped many organizations make the
leap to this human-centered method. And we’ve seen firsthand the
dramatically improved understanding of developer productiveness that it
supplies.
What we’re referring to right here is qualitative measurement. On this
article, we offer a primer on this method derived from our expertise
serving to many organizations on this journey. We start with a definition of
qualitative metrics and advocate for them. We comply with with sensible
steering on seize, monitor, and make the most of this knowledge.
Right now, developer productiveness is a vital concern for companies amid
the backdrop of fiscal tightening and transformational applied sciences corresponding to
AI. As well as, developer expertise and platform engineering are garnering
elevated consideration as enterprises look past Agile and DevOps
transformation. What all these issues share is a reliance on measurement
to assist information choices and monitor progress. And for this, qualitative
measurement is vital.
Word: after we say “developer productiveness”, we imply the diploma to which
builders’ can do their work in a frictionless method – not the person
efficiency of builders. Some organizations discover “developer productiveness”
to be a problematic time period due to the way in which it may be misinterpreted by
builders. We advocate that organizations use the time period “developer
expertise,” which has extra optimistic connotations for builders.
What’s a qualitative metric?
We outline a qualitative metric as a measurement comprised of knowledge
offered by people. This can be a sensible definition – we haven’t discovered a
singular definition throughout the social sciences, and the choice
definitions we’ve seen have flaws that we focus on later on this
part.
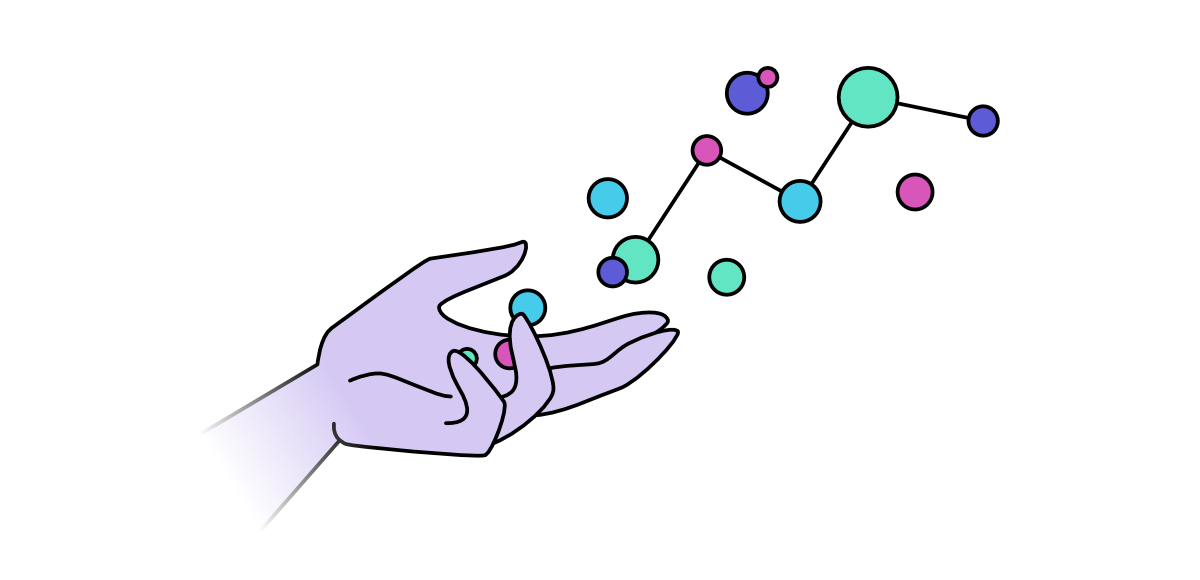
Determine 1: Qualitative metrics are measurements derived from people
The definition of the phrase “metric” is unambiguous. The time period
“qualitative,” nevertheless, has no authoritative definition as famous within the
2019 journal paper What’s Qualitative in
Qualitative Analysis:
There are various definitions of qualitative analysis, but when we search for
a definition that addresses its distinctive function of being
“qualitative,” the literature throughout the broad discipline of social science is
meager. The primary cause behind this text lies within the paradox, which, to
put it bluntly, is that researchers act as in the event that they know what it’s, however
they can not formulate a coherent definition.
An alternate definition we’ve heard is that qualitative metrics measure
high quality, whereas quantitative metrics measure amount. We’ve discovered this
definition problematic for 2 causes: first, the time period “qualitative
metric” consists of the time period metric, which suggests that the output is a
amount (i.e., a measurement). Second, high quality is often measured
by way of ordinal scales which can be translated into numerical values and
scores – which once more, contradicts the definition.
One other argument we’ve heard is that the output of sentiment evaluation
is quantitative as a result of the evaluation leads to numbers. Whereas we agree
that the info ensuing from sentiment evaluation is quantitative, primarily based on
our unique definition that is nonetheless a qualitative metric (i.e., a amount
produced qualitatively) until one had been to take the place that
“qualitative metric” is altogether an oxymoron.
Other than the issue of defining what a qualitative metric is, we’ve
additionally encountered problematic colloquialisms. One instance is the time period “delicate
metric”. We warning towards this phrase as a result of it harmfully and
incorrectly implies that knowledge collected from people is weaker than “arduous
metrics” collected from methods. We additionally discourage the time period “subjective
metrics” as a result of it misconstrues the truth that knowledge collected from people
might be both goal or subjective – as we focus on within the subsequent
part.
Kind | Definition | Instance |
---|---|---|
Attitudinal metrics | Subjective emotions, opinions, or attitudes towards a particular topic. | How glad are you together with your IDE, on a scale of 1–10? |
Behavioral metrics | Goal info or occasions pertaining to a person’s work expertise. | How lengthy does it take so that you can deploy a change to manufacturing? |
Later on this article we offer steering on gather and use
these measurements, however first we’ll present a real-world instance of this
method put to observe
Peloton is an American expertise firm
whose developer productiveness measurement technique facilities round
qualitative metrics. To gather qualitative metrics, their group
runs a semi-annual developer expertise survey led by their Tech
Enablement & Developer Expertise group, which is a part of their Product
Operations group.
Thansha Sadacharam, head of tech studying and insights, explains: “I
very strongly imagine, and I feel quite a lot of our engineers additionally actually
admire this, that engineers aren’t robots, they’re people. And simply
taking a look at primary numbers would not drive the entire story. So for us, having
a very complete survey that helped us perceive that whole
developer expertise was actually essential.”
Every survey is distributed to
a random pattern of roughly half of their builders. With this method,
particular person builders solely have to take part in a single survey per 12 months,
minimizing the general time spent on filling out surveys whereas nonetheless
offering a statistically important consultant set of knowledge outcomes.
The Tech Enablement & Developer Expertise group can also be liable for
analyzing and sharing the findings from their surveys with leaders throughout
the group.
For extra on Peloton’s developer expertise survey, hearken to this
interview
with Thansha Sadacharam.
Advocating for qualitative metrics
Executives are sometimes skeptical in regards to the reliability or usefulness of
qualitative metrics. Even extremely scientific organizations like Google have
needed to overcome these biases. Engineering leaders are inclined towards
system metrics since they’re accustomed to working with telemetry knowledge
for inspecting methods. Nonetheless, we can not depend on this similar method for
measuring individuals.
Keep away from pitting qualitative and quantitative metrics towards one another.
We’ve seen some organizations get into an inside “battle of the
metrics” which isn’t a very good use of time or power. Our recommendation for
champions is to keep away from pitting qualitative and quantitative metrics towards
one another as an both/or. It’s higher to make the argument that they’re
complementary instruments – as we cowl on the finish of this text.
We’ve discovered that the underlying reason for opposition to qualitative knowledge
are misconceptions which we deal with under. Later on this article, we
define the distinct advantages of self-reported knowledge corresponding to its capacity to
measure intangibles and floor vital context.
False impression: Qualitative knowledge is simply subjective
Conventional office surveys usually concentrate on the subjective
opinions and emotions of their staff. Thus many engineering leaders
intuitively imagine that surveys can solely gather subjective knowledge from
builders.
As we describe within the following part, surveys may also seize
goal details about info or occasions. Google’s DevOps Analysis and
Evaluation (DORA) program is a wonderful concrete
instance.
Some examples of goal survey questions:
- How lengthy does it take to go from code dedicated to code efficiently
working in manufacturing? - How usually does your group deploy code to manufacturing or
launch it to finish customers?
False impression: Qualitative knowledge is unreliable
One problem of surveys is that individuals with all method of backgrounds
write survey questions with no particular coaching. In consequence, many
office surveys don’t meet the minimal requirements wanted to provide
dependable or legitimate measures. Nicely designed surveys, nevertheless, produce
correct and dependable knowledge (we offer steering on how to do that later in
the article).
Some organizations have issues that individuals might lie in surveys. Which
can occur in conditions the place there’s worry round how the info shall be
used. In our expertise, when surveys are deployed as a instrument to assist
perceive and enhance bottlenecks affecting builders, there isn’t any
incentive for respondents to lie or recreation the system.
Whereas it’s true that survey knowledge isn’t all the time 100% correct, we regularly
remind leaders that system metrics are sometimes imperfect too. For instance,
many organizations try to measure CI construct occasions utilizing knowledge aggregated
from their pipelines, solely to seek out that it requires important effort to
clear the info (e.g. excluding background jobs, accounting for parallel
jobs) to provide an correct consequence
The 2 varieties of qualitative metrics
There are two key varieties of qualitative metrics:
- Attitudinal metrics seize subjective emotions, opinions, or
attitudes towards a particular topic. An instance of an attitudinal measure would
be the numeric worth captured in response to the query: “How glad are
you together with your IDE, on a scale of 1-10?”. - Behavioral metrics seize goal info or occasions pertaining to an
people’ work experiences. An instance of a behavioral measure could be the
amount captured in response to the query: “How lengthy does it take so that you can
deploy a change to manufacturing?”
We’ve discovered that the majority tech practitioners overlook behavioral measures
when desirous about qualitative metrics. This happens regardless of the
prevalence of qualitative behavioral measures in software program analysis, such
because the Google’s DORA program talked about earlier.
DORA publishes annual benchmarks for metrics corresponding to lead time for
adjustments, deployment frequency, and alter fail fee. Unbeknownst to many,
DORA’s benchmarks are captured utilizing qualitative strategies with the survey
objects proven under:
Lead time
For the first software or service you’re employed on,
what’s your lead time for adjustments (that’s, how lengthy does it take to go
from code dedicated to code efficiently working in manufacturing)?
Greater than six months
One to 6 months
One week to 1 month
Someday to 1 week
Lower than at some point
Lower than one hour
Deploy frequency
For the first software or service you
work on, how usually does your group deploy code to manufacturing or
launch it to finish customers?
Fewer than as soon as per six months
Between as soon as per 30 days and as soon as each six months
Between as soon as per week and as soon as per 30 days
Between as soon as per day and as soon as per week
Between as soon as per hour and as soon as per day
On demand (a number of deploys per day)
Change fail proportion
For the first software or service you’re employed on, what
proportion of adjustments to manufacturing or releases to customers lead to
degraded service (for instance, result in service impairment or service
outage) and subsequently require remediation (for instance, require a
hotfix, rollback, repair ahead, patch)?
0–15%
16–30%
31–45%
46–60%
61–75%
76–100%
Time to revive
For the first software or service you’re employed on, how lengthy
does it usually take to revive service when a service incident or a
defect that impacts customers happens (for instance, unplanned outage, service
impairment)?
Greater than six months
One to 6 months
One week to 1 month
Someday to 1 week
Lower than at some point
Lower than one hour
We’ve discovered that the flexibility to gather attitudinal and behavioral knowledge
on the similar time is a strong advantage of qualitative measurement.
For instance, behavioral knowledge would possibly present you that your launch course of
is quick and environment friendly. However solely attitudinal knowledge may inform you whether or not it
is clean and painless, which has essential implications for developer
burnout and retention.
To make use of a non-tech analogy: think about you’re feeling sick and go to a
physician. The physician takes your blood stress, your temperature, your coronary heart
fee, they usually say “Nicely, it appears such as you’re all good. There’s nothing
flawed with you.” You’ll be greatly surprised! You’d say, “Wait, I’m telling
you that one thing feels flawed.”
The advantages of qualitative metrics
One argument for qualitative metrics is that they keep away from subjecting
builders to the sensation of “being measured” by administration. Whereas we’ve
discovered this to be true – particularly when in comparison with metrics derived from
builders’ Git or Jira knowledge – it doesn’t deal with the primary goal
advantages that qualitative approaches can present.
There are three fundamental advantages of qualitative metrics relating to
measuring developer productiveness:
Qualitative metrics will let you measure issues which can be in any other case
unmeasurable
System metrics like lead time and deployment quantity seize what’s
occurring in our pipelines or ticketing methods. However there are various extra
elements of builders’ work that must be understood to be able to enhance
productiveness: for instance, whether or not builders are in a position to keep within the move
or work or simply navigate their codebases. Qualitative metrics allow you to
measure these intangibles which can be in any other case tough or unimaginable to
measure.
An attention-grabbing instance of that is technical debt. At Google, a examine to
determine metrics for technical debt included an evaluation of 117 metrics
that had been proposed as potential indicators. To the frustration of
Google researchers, no single metric or mixture of metrics had been discovered
to be legitimate indicators (for extra on how Google measures technical debt,
hearken to this interview).
Whereas there might exist an undiscovered goal metric for technical
debt, one can suppose that this can be unimaginable attributable to the truth that
evaluation of technical debt depends on the comparability between the present
state of a system or codebase versus its imagined excellent state. In different
phrases, human judgment is important.
Qualitative metrics present lacking visibility throughout groups and
methods
Metrics from ticketing methods and pipelines give us visibility into
among the work that builders do. However this knowledge alone can not give us
the total story. Builders do quite a lot of work that’s not captured in tickets
or builds: for instance, designing key options, shaping the route of a
mission, or serving to a teammate get onboarded.
It’s unimaginable to realize visibility into all these actions by way of
knowledge from our methods alone. And even when we may theoretically gather
all the info by way of methods, there are further challenges to capturing
metrics by way of instrumentation.
One instance is the problem of normalizing metrics throughout totally different
group workflows. For instance, for those who’re making an attempt to measure how lengthy it takes
for duties to go from begin to completion, you would possibly attempt to get this knowledge
out of your ticketing instrument. However particular person groups usually have totally different
workflows that make it tough to provide an correct metric. In
distinction, merely asking builders how lengthy duties usually take might be
a lot easier.
One other frequent problem is cross-system visibility. For instance, a
small startup can measure TTR (time to revive) utilizing simply a problem
tracker corresponding to Jira. A big group, nevertheless, will possible have to
consolidate and cross-attribute knowledge throughout planning methods and deployment
pipelines to be able to acquire end-to-end system visibility. This generally is a
yearlong effort, whereas capturing this knowledge from builders can present a
baseline rapidly.
Qualitative metrics present context for quantitative knowledge
As technologists, it’s straightforward to focus closely on quantitative measures.
They appear clear and clear, afterall. There’s a threat, nevertheless, that the
full story isn’t being informed with out richer knowledge and that this will lead us
into specializing in the flawed factor.
One instance of that is code evaluation: a typical optimization is to attempt to
velocity up the code evaluation. This appears logical as ready for a code evaluation
could cause wasted time or undesirable context switching. We may measure the
time it takes for opinions to be accomplished and incentivize groups to enhance
it. However this method might encourage unfavourable conduct: reviewers speeding
by way of opinions or builders not discovering the correct consultants to carry out
opinions.
Code opinions exist for an essential function: to make sure top quality
software program is delivered. If we do a extra holistic evaluation – specializing in the
outcomes of the method relatively than simply velocity – we discover that optimization
of code evaluation should guarantee good code high quality, mitigation of safety
dangers, constructing shared data throughout group members, in addition to making certain
that our coworkers aren’t caught ready. Qualitative measures may help us
assess whether or not these outcomes are being met.
One other instance is developer onboarding processes. Software program improvement
is a group exercise. Thus if we solely measure particular person output metrics such
as the speed new builders are committing or time to first commit, we miss
essential outcomes e.g. whether or not we’re absolutely using the concepts the
builders are bringing, whether or not they really feel protected to ask questions and if
they’re collaborating with cross-functional friends.
How one can seize qualitative metrics
Many tech practitioners don’t understand how tough it’s to write down good
survey questions and design good survey devices. The truth is, there are
complete fields of examine associated to this, corresponding to psychometrics and
industrial psychology. It is very important convey or construct experience right here
when potential.
Beneath are few good guidelines for writing surveys to keep away from the most typical
errors we see organizations make:
- Survey objects must be rigorously worded and each query ought to solely ask
one factor. - If you wish to evaluate outcomes between surveys, watch out about altering
the wording of questions such that you simply’re measuring one thing totally different. - Should you change any wording, you have to do rigorous statistical exams.
In survey parlance, ”good surveys” means “legitimate and dependable” or
“demonstrating good psychometric properties.” Validity is the diploma to
which a survey merchandise really measures the assemble you need to measure.
Reliability is the diploma to which a survey merchandise produces constant
outcomes out of your inhabitants and over time.
One mind-set about survey design that we’ve discovered useful to
tech practitioners: consider the survey response course of as an algorithm
that takes place within the human thoughts.
When a person is introduced a survey query, a collection of psychological
steps happen to be able to arrive at a response. The mannequin under is from
the seminal 2012 e-book, The Psychology of Survey
Response:
Part | Particular Processes |
---|---|
Comprehension |
Attend to questions and directions Signify logical type of query Determine query focus (info sought) Hyperlink key phrases to related ideas |
Retrieval |
Generate retrieval technique and cues Retrieve particular, generic recollections Fill in lacking particulars |
Judgment |
Assess completeness and relevance of recollections Draw inferences primarily based on accessibility Combine materials retrieved Make estimate primarily based on partial retrieval |
Response |
Map Judgement onto response class Edit response |
Decomposing the survey response course of and inspecting every step
may help us refine our inputs to provide extra correct survey outcomes.
Growing good survey objects requires rigorous design, testing, and
evaluation – identical to the method of designing software program!
However good survey design is only one side of working profitable surveys.
Extra challenges embrace participation charges, knowledge evaluation, and figuring out
act on knowledge. Beneath are among the finest practices we’ve
discovered.
Phase outcomes by group and persona
A typical mistake made by organizational leaders is to concentrate on companywide
outcomes as a substitute of knowledge damaged down by group and persona (e.g., function, tenure,
seniority). As beforehand described, developer expertise is extremely contextual
and might differ radically throughout groups or roles. Focusing solely on mixture
outcomes can result in overlooking issues that have an effect on small however essential
populations throughout the firm, corresponding to cell builders.
Evaluate outcomes towards benchmarks
Comparative evaluation may help contextualize knowledge and assist drive motion. For
instance, developer sentiment towards code high quality generally skews unfavourable, making
it tough to determine true issues or gauge their magnitude. The extra
actionable knowledge level is: “are our builders extra annoyed about code
high quality than different groups or organizations?” Groups with decrease sentiment scores
than their friends and organizations with decrease scores than their business friends
can floor notable alternatives for enchancment.
Use transactional surveys the place acceptable
Transactional surveys seize suggestions throughout particular touchpoints or
interactions within the developer workflow. For instance, platform groups can use
transactional surveys to immediate builders for suggestions whereas they’re within the midst of
creating a brand new service in an inside developer portal. Transactional surveys can
additionally increase knowledge from periodic surveys by producing higher-frequency suggestions and
extra granular insights.
Keep away from survey fatigue
Many organizations battle to maintain excessive participation charges in surveys
over time. Lack of follow-up could cause builders to really feel that
repeatedly responding to surveys isn’t worthwhile. It’s subsequently
vital that leaders and groups comply with up and take significant motion after surveys.
Whereas a quarterly or
semi-annual survey cadence is perfect for many organizations, we’ve seen some
organizations achieve success with extra frequent surveys which can be built-in into
common group rituals corresponding to retrospectives.
Survey Template
Beneath are a easy set of survey questions for getting began. Load the questions
under into your most well-liked survey instrument, or get began rapidly by making a duplicate of our ready-to-go
Google Varieties template.
The template is deliberately easy, however surveys usually turn into fairly sizable as your measurement
technique matures. For instance, Shopify’s developer survey is 20-minutes
lengthy and Google’s is over 30-minutes lengthy.
After you have collected responses, rating the a number of alternative questions
utilizing both imply or high field scoring. Imply scores are calculated by
assigning every choice a worth between 1 and 5 and taking the typical.
Prime field scores are calculated by the odds of responses that
select one of many high two most favorable choices.
Remember to evaluation open textual content responses which may comprise nice
info. Should you’ve collected numerous feedback, LLM instruments
corresponding to ChatGPT might be helpful for extracting core themes and
solutions. Whenever you’ve completed analyzing outcomes, be sure you share
your findings with respondents so their time filling out the survey
feels worthwhile.
How straightforward or tough is it so that you can do work as a
developer or technical contributor at [INSERT ORGANIATION NAME]?
Very tough
Considerably tough
Neither straightforward nor tough
Considerably straightforward
Very straightforward
For the first software or service you’re employed on, what
is your lead time for adjustments (that’s, how lengthy does it take to go
from code dedicated to code efficiently working in
manufacturing)?
A couple of month
One week to 1 month
Someday to 1 week
Lower than at some point
Lower than one hour
How usually do you’re feeling extremely productive in your
work?
By no means
A little bit of the time
A number of the time
More often than not
The entire time
Please fee your settlement or disagreement with the next
statements:
My group follows improvement finest practices | □ | □ | □ | □ | □ |
I’ve sufficient time for deep work. | □ | □ | □ | □ | □ |
I’m glad with the quantity of automated take a look at protection in my mission. |
□ | □ | □ | □ | □ |
It is simple for me to deploy to manufacturing. | □ | □ | □ | □ | □ |
I am glad with the standard of our CI/CD tooling. | □ | □ | □ | □ | □ |
My group’s codebase is simple for me to contribute to. | □ | □ | □ | □ | □ |
The quantity of technical debt on my group is acceptable primarily based on our targets. | □ | □ | □ | □ | □ |
Specs are constantly revisited and reprioritized in keeping with person alerts. | □ | □ | □ | □ | □ |
Please share any further suggestions on how your developer expertise might be improved
[open textarea]
Utilizing qualitative and quantitative metrics collectively
Qualitative metrics and quantitative metrics are complementary approaches
to measuring developer productiveness. Qualitative metrics, derived from
surveys, present a holistic view of productiveness that features each subjective
and goal measurements. Quantitative metrics, however, present
distinct benefits as properly:
- Precision. People can inform you whether or not their CI/CD builds are usually
quick or gradual (i.e., whether or not durations are nearer to a minute or an hour), however
they can not report on construct occasions right down to millisecond precision. Quantitative
metrics are wanted when a excessive diploma of precision is required in our
measurements. - Continuity. Usually, the frequency at which a corporation can survey
their builders is at most a few times per quarter. With a purpose to gather extra
frequent or steady metrics, organizations should collect knowledge
systematically.
Finally, it’s by way of the mixture of qualitative and quantitative metrics – a mixed-methods method –
that organizations can acquire most visibility into the productiveness and
expertise of builders. So how do you utilize qualitative and quantitative
metrics collectively?
We’ve seen organizations discover success after they begin with qualitative
metrics to determine baselines and decide the place to focus. Then, comply with with
quantitative metrics to assist drill in deeper into particular areas.
Engineering leaders discover this method to be efficient as a result of qualitative
metrics present a holistic view and context, offering broad understanding of
potential alternatives. Quantitative metrics, however, are
usually solely accessible for a narrower set of the software program supply
course of.
Google equally advises its engineering leaders to go to survey knowledge first
earlier than taking a look at logs knowledge because of this. Google engineering researcher
Ciera Jaspan explains: “We encourage leaders to go to the survey knowledge first,
as a result of for those who solely take a look at logs knowledge it would not actually inform you whether or not
one thing is nice or dangerous. For instance, we’ve a metric that tracks the time
to make a change, however that quantity is ineffective by itself. You do not know, is
this a very good factor? Is it a foul factor? Do we’ve an issue?”.
A combined strategies method permits us to benefit from the advantages of
each qualitative and quantitative metrics whereas getting a full perceive of
developer productiveness:
- Begin with qualitative knowledge to determine your high alternatives
- As soon as what you need to enhance, use quantitative metrics to
drill-in additional - Monitor your progress utilizing each qualitative and quantitative metrics
It is just by combining as a lot knowledge as potential – each qualitative and
quantitative – that organizations can start to construct a full understanding of
developer productiveness.
Ultimately, nevertheless, it’s essential to recollect: organizations spend so much
on extremely certified people that may observe and detect issues that log-based
metrics can’t. By tapping into the minds and voices of builders,
organizations can unlock insights beforehand seen as unimaginable.