Scams
Your humble cellphone quantity is extra invaluable than you might assume. Right here’s the way it may fall into the mistaken fingers – and how one can assist hold it out of the attain of fraudsters.
15 Jul 2024
•
,
7 min. learn
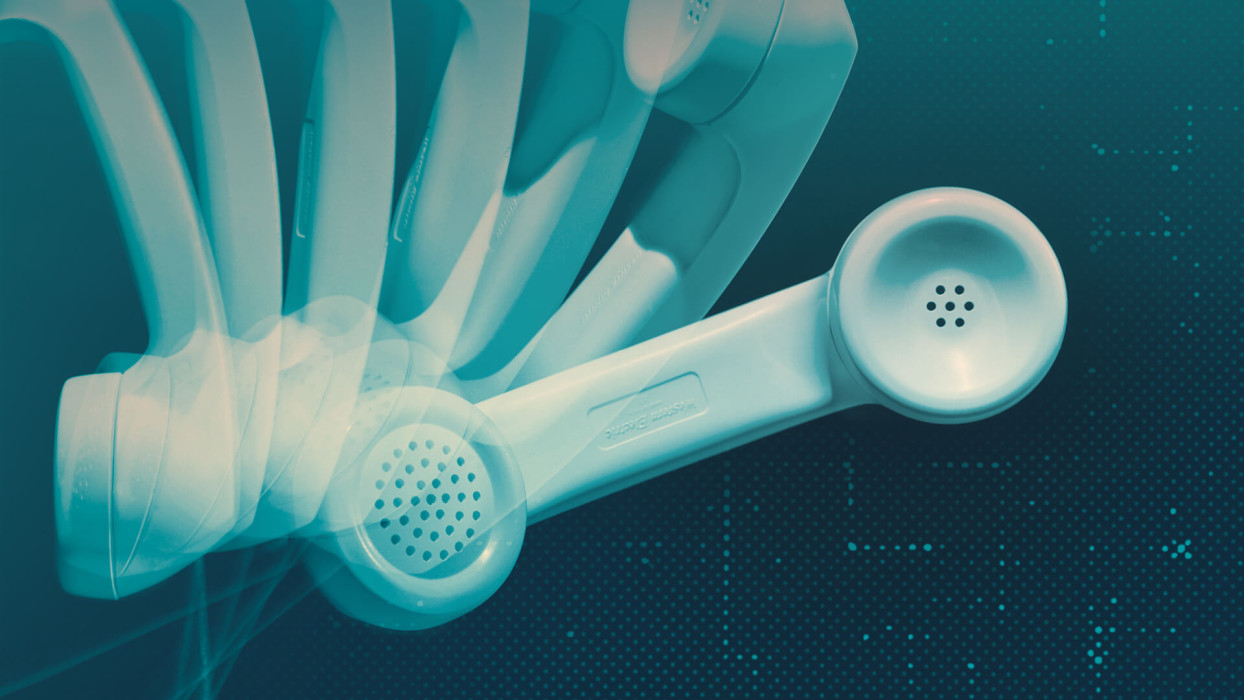
What is perhaps one of many best methods to rip-off somebody out of their cash – anonymously, in fact?
Would it not contain stealing their bank card information, maybe utilizing digital skimming or after hacking right into a database of delicate private info? Whereas efficient, these strategies could also be resource-intensive and require some technical prowess.
What about stealing cost data through pretend web sites? This will likely certainly match the invoice, however spoofing official web sites (and e mail addresses to “unfold the phrase”) might not be for everyone, both. The percentages are additionally excessive that such ploys can be noticed in time by the security-savvy amongst us or thwarted by safety controls.
As a substitute, dangerous actors are turning to extremely scalable operations that depend on refined social engineering techniques and prices little to function. Utilizing voice phishing (additionally known as vishing) and message scams (smishing), these operations have been developed right into a rip-off call-center trade value billions of {dollars}.
For starters, these ploys could not require a lot in the way in which of specialised or technical abilities. Additionally, a single particular person (usually a sufferer of human trafficking) can, at a time, ensnare a number of unwitting victims in numerous flavors of fraud. These usually contain pig butchering, cryptocurrency schemes, romance scams, and tech assist fraud, every of which spins a compelling yarn and preys on a few of what truly makes us human.
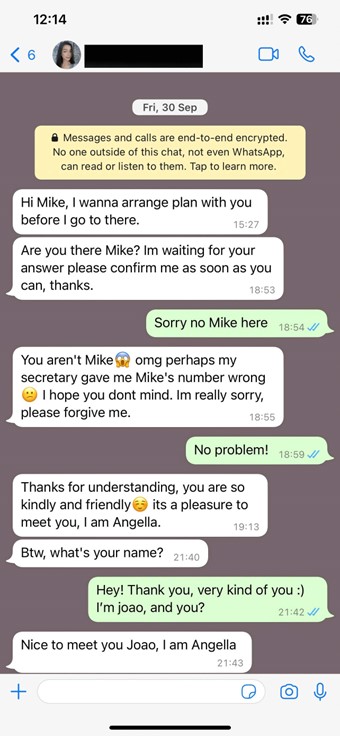
Howdy? Is that this factor on?
Think about receiving a name out of your financial institution to tell you that your account has been breached and with the intention to hold your cash protected, you have to share your delicate particulars with them. The urgency within the voice of the financial institution’s “worker” could certainly be sufficient to immediate you to share your delicate info. The issue is, this particular person won’t be out of your financial institution – or they might not even exist in any respect. It might be only a fabricated voice, however nonetheless sound utterly pure.
This isn’t in any respect unusual and cautionary tales from current years abound. Again in 2019, a CEO was scammed out of virtually US$250,000 by a convincing voice deepfake of their mother or father firm’s chief. Equally, a finance employee was tricked through a deepfake video name in 2024, costing their agency US$25 million.
AI, the enabler
With trendy AI voice cloning and translation capabilities, vishing and smishing have develop into simpler than ever. Certainly, ESET World Cybersecurity Advisor Jake Moore demonstrated the benefit with which anyone can create a convincing deepfake model of another person – together with somebody properly. Seeing and listening to are now not believing.
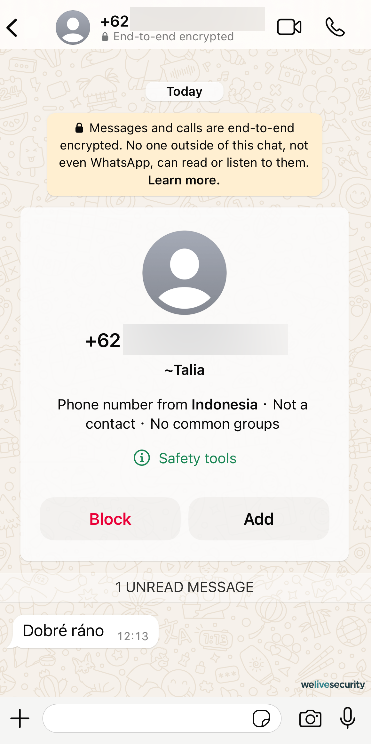
AI is reducing the barrier of entry for brand spanking new adversaries, serving as a multipronged instrument to collect information, automate tedious duties, and globalize their attain. Consequently, phishing utilizing AI-generated voices and textual content will most likely develop into extra commonplace.
On this observe, a current report by Enea famous a 1,265% rise in phishing scams for the reason that launch of ChatGPT in November 2022 and spotlighted the potential of enormous language fashions to assist gas such malicious operations.
What’s your identify, what’s your quantity?
As evidenced by Shopper Stories analysis from 2022, persons are turning into extra privacy-conscious than earlier than. Some 75% of the survey’s respondents have been no less than considerably involved in regards to the privateness of their information collected on-line, which can embrace cellphone numbers, as they’re a invaluable useful resource for each identification and promoting.
However now that we’re properly previous the age of the Yellow Pages, how does this connection between cellphone numbers and promoting work?
Contemplate this illustrative instance: a baseball fan positioned tickets in a devoted app’s checkout however did not full the acquisition. And but, shortly after closing the app, he obtained a cellphone name providing a reduction on the tickets. Naturally, he was baffled since he didn’t keep in mind offering his cellphone quantity to the app. How did it get his quantity, then?
The reply is – through monitoring. Some trackers can acquire particular info from a webpage, so after you’ve crammed of their cellphone quantity in a type, a tracker may detect and retailer it to create what is usually known as customized content material and expertise. There may be a complete enterprise mannequin often called “information brokering”, and the dangerous information is that it doesn’t take a breach for the information to develop into public.
Monitoring, information brokers, and leaks
Information brokers vacuum up your private info from publicly out there sources (authorities licenses/registrations), business sources (enterprise companions like bank card suppliers or shops) in addition to by monitoring your on-line actions (actions on social media, advert clicks, and many others.), earlier than promoting your info to others.
Nonetheless, the query in your lips could also be: how can scammers receive different individuals’s cellphone numbers?
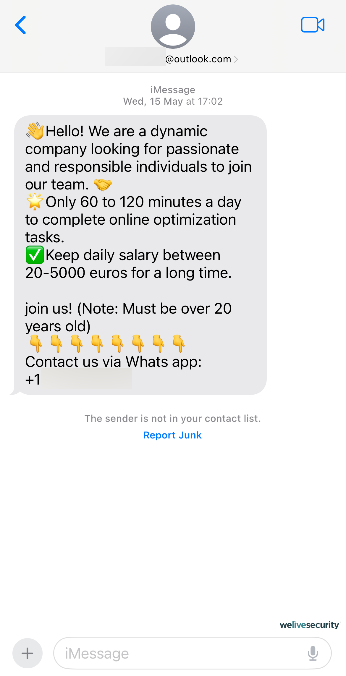
Naturally, the extra corporations, websites, and apps you share your private info with, the extra detailed your private “advertising and marketing profile” is. This additionally will increase your publicity to information leaks, since information brokers themselves can expertise safety incidents. A knowledge dealer may additionally promote your info to others, probably together with dangerous actors.
However information brokers, or breaches affecting them, aren’t the one supply of cellphone numbers for scammers. Listed below are another methods during which criminals can get ahold of your cellphone quantity:
- Public sources: Social media websites or on-line job markets would possibly present your cellphone quantity as a method to make a connection. In case your privateness settings should not dialed in accurately or you aren’t conscious of the implications of revealing your cellphone quantity in your social media profile, your quantity is perhaps out there to anybody, even an AI internet scraper.
- Stolen accounts: Varied on-line providers require your cellphone quantity, be it to verify your identification, to position an order, or to function an authentication issue. When your accounts get brute-forced on account of weak passwords or considered one of your on-line suppliers suffers an information breach your quantity may simply leak as properly.
- Autodialers: Autodialers name random numbers, and as quickly as you reply the decision, you might be focused by a rip-off. Generally these autodialers name simply to verify that the quantity is in use in order that it may be added to a listing of targets.
- Mail: Test any of your current deliveries – these often have your handle seen on the letter/field, however in some instances, they’ll even have your e mail or cellphone quantity printed on them. What if somebody stole considered one of your deliveries or rummaged by way of your recycling pile? Contemplating that information leaks often comprise the identical info, this may be very harmful and grounds for additional exploitation.
For example of a wide-scale breach affecting cellphone numbers, AT&T lately revealed that hundreds of thousands of shoppers’ name and textual content message information from mid-to-late 2022 have been uncovered in an enormous information leak. Almost all the firm’s prospects and other people utilizing the cell community have had their numbers, name durations, and variety of name interactions uncovered. Whereas name and textual content contents are allegedly not among the many breached information, buyer names and numbers can nonetheless be simply linked, as reported by CNN.
Reportedly, the blame may be placed on a third-party cloud platform, which a malicious actor had accessed. Coincidentally, the identical platform has had a number of instances of large leaks linked to it lately.
How you can shield your cellphone quantity
So, how will you shield your self and your quantity? Listed below are a couple of suggestions:
- Concentrate on phishing. By no means reply unsolicited messages/calls from overseas numbers, don’t click on on random hyperlinks in your emails/messages, and keep in mind to maintain cool and assume earlier than you react to a seemingly pressing state of affairs, as a result of that’s how they get you.
- Ask your service supplier about their SIM safety measures. They could have an possibility for card locks to guard towards SIM swapping, for instance, or further account safety layers to forestall scams like name forwarding.
- Shield your accounts with two-factor authentication, ideally utilizing devoted safety keys, apps, or biometrics as an alternative of SMS-based verification. The latter may be intercepted by dangerous actors with relative ease. Do the identical for service supplier accounts as properly.
- Suppose twice earlier than offering your cellphone quantity to a web site. Whereas having it as a further restoration possibility in your numerous apps is perhaps helpful, different strategies like secondary emails/authenticators may supply a safer various.
- For on-line purchases, think about using a pre-paid SIM card or a VoIP service as an alternative of your common cellphone quantity.
- Use a cellular safety resolution with name filtering, in addition to be sure that third-party cookies in your internet browser are blocked, and discover different privacy-enhancing instruments and applied sciences.
In a world that more and more depends on on-line report preserving, there’s a low likelihood that your quantity received’t be preserved by a 3rd celebration someplace. And because the AT&T incident suggests, relying by yourself service’s safety can be slightly problematic. This doesn’t imply that you must stay in a state of fixed paranoia, although.
Alternatively, it highlights the significance of committing to correct cyber hygiene and being conscious of your information on-line. Vigilance can be nonetheless key, particularly when contemplating the implications of this new, AI-powered (beneath)world.