The Chinese language firm in control of handing out domains ending in “.prime” has been given till mid-August 2024 to point out that it has put in place programs for managing phishing stories and suspending abusive domains, or else forfeit its license to promote domains. The warning comes amid the discharge of latest findings that .prime was the most typical suffix in phishing web sites over the previous yr, second solely to domains ending in “.com.”
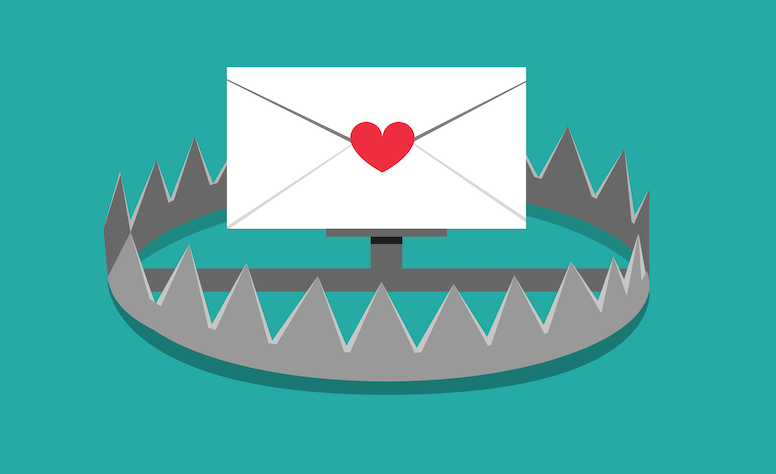
Picture: Shutterstock.
On July 16, the Web Company for Assigned Names and Numbers (ICANN) despatched a letter to the homeowners of the .prime area registry. ICANN has filed tons of of enforcement actions towards area registrars over time, however on this case ICANN singled out a site registry liable for sustaining a whole top-level area (TLD).
Amongst different causes, the missive chided the registry for failing to answer stories about phishing assaults involving .prime domains.
“Based mostly on the knowledge and information gathered by a number of weeks, it was decided that .TOP Registry doesn’t have a course of in place to promptly, comprehensively, and fairly examine and act on stories of DNS Abuse,” the ICANN letter reads (PDF).
ICANN’s warning redacted the identify of the recipient, however information present the .prime registry is operated by a Chinese language entity referred to as Jiangsu Bangning Science & Know-how Co. Ltd. Representatives for the corporate haven’t responded to requests for remark.
Domains ending in .prime had been represented prominently in a brand new phishing report launched immediately by the Interisle Consulting Group, which sources phishing knowledge from a number of locations, together with the Anti-Phishing Working Group (APWG), OpenPhish, PhishTank, and Spamhaus.
Interisle’s latest examine examined almost two million phishing assaults within the final yr, and located that phishing websites accounted for greater than 4 p.c of all new .prime domains between Might 2023 and April 2024. Interisle stated .prime has roughly 2.76 million domains in its secure, and that greater than 117,000 of these had been phishing websites previously yr.
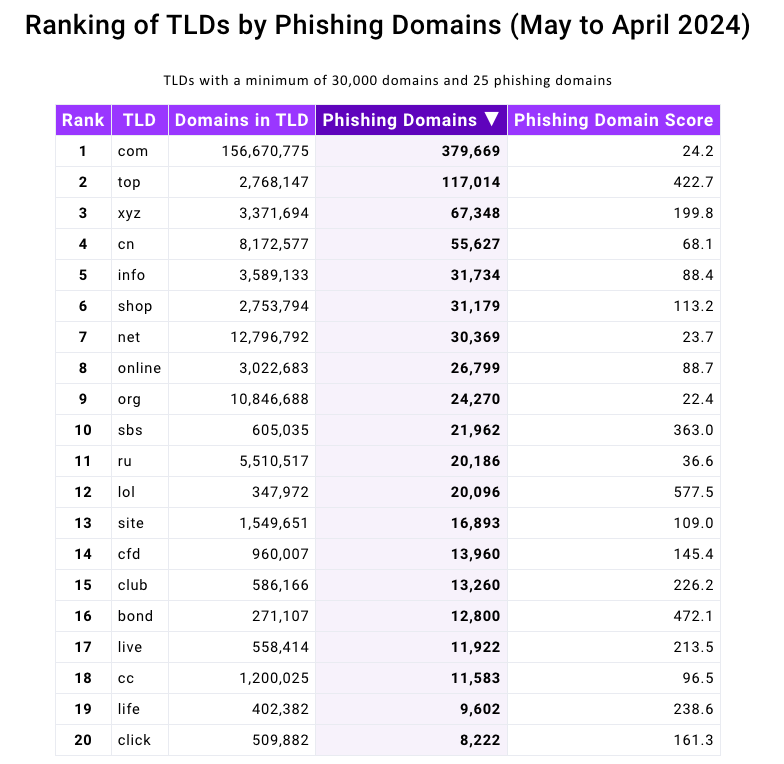
Supply: Interisle Consulting Group.
ICANN stated its overview was based mostly on info collected and studied about .prime domains over the previous few weeks. However the truth that excessive volumes of phishing websites are being registered by Jiangsu Bangning Science & Know-how Co Ltd. is hardly a brand new pattern.
For instance, greater than 10 years in the past the identical Chinese language registrar was the fourth most typical supply of phishing web sites, as tracked by the APWG. Keep in mind that the APWG report excerpted under was revealed greater than a yr earlier than Jiangsu Bangning acquired ICANN approval to introduce and administer the brand new .prime registry.
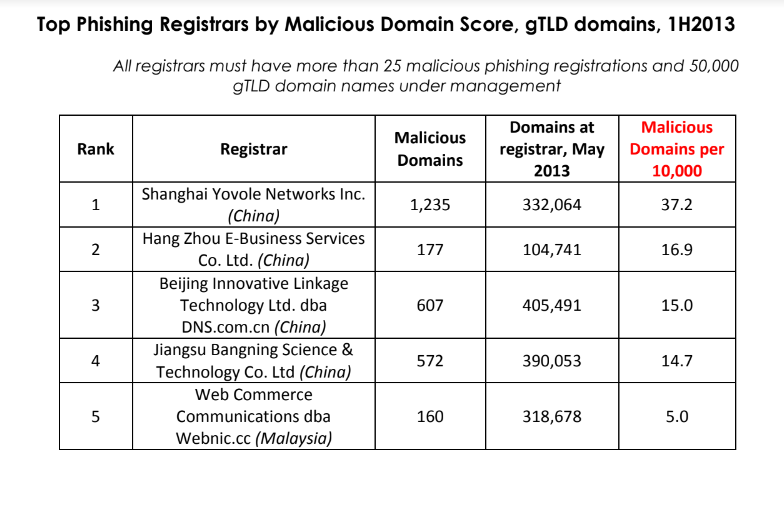
Supply: APWG phishing report from 2013, two years earlier than .prime got here into being.
An interesting new wrinkle within the phishing panorama is the expansion in rip-off pages hosted by way of the InterPlanetary File System (IPFS), a decentralized knowledge storage and supply community that’s based mostly on peer-to-peer networking. In accordance with Interisle, the usage of IPFS to host and launch phishing assaults — which might make phishing websites tougher to take down — elevated a staggering 1,300 p.c, to roughly 19,000 phishing websites reported within the final yr.
Final yr’s report from Interisle discovered that domains ending in “.us” — the top-level area for the US — had been among the many most prevalent in phishing scams. Whereas .us domains usually are not even on the Prime 20 record of this yr’s examine, “.com” maintained its perennial #1 spot as the biggest supply of phishing domains total.
A yr in the past, the phishiest area registrar by far was Freenom, a now-defunct registrar that handed out free domains in a number of country-code TLDs, together with .tk, .ml, .ga and .cf. Freenom went out of enterprise after being sued by Meta, which alleged Freenom ignored abuse complaints whereas monetizing visitors to abusive domains.
Following Freenom’s demise, phishers shortly migrated to different new low-cost TLDs and to providers that permit nameless, free area registrations — notably subdomain providers. For instance, Interisle discovered phishing assaults involving web sites created on Google’s blogspot.com skyrocketed final yr greater than 230 p.c. Different subdomain providers that noticed a considerable development in domains registered by phishers embrace weebly.com, github.io, wix.com, and ChangeIP, the report notes.
Interisle Consulting companion Dave Piscitello stated ICANN may simply ship related warning letters to no less than a half-dozen different top-level area registries, noting that spammers and phishers are likely to cycle by the identical TLDs periodically — together with .xyz, .information, .help and .lol, all of which noticed significantly extra enterprise from phishers after Freenom’s implosion.
Piscitello stated area registrars and registries may considerably scale back the variety of phishing websites registered by their providers simply by flagging prospects who attempt to register big volumes of domains without delay. Their examine discovered that no less than 27% of the domains used for phishing had been registered in bulk — i.e. the identical registrant paid for tons of or 1000’s of domains in fast succession.
The report features a case examine wherein a phisher this yr registered 17,562 domains over the course of an eight-hour interval — roughly 38 domains per minute — utilizing .lol domains that had been all composed of random letters.
ICANN tries to resolve contract disputes privately with the registry and registrar group, and consultants say the nonprofit group normally solely publishes enforcement letters when the recipient is ignoring its non-public notices. Certainly, ICANN’s letter notes Jiangsu Bangning didn’t even open its emailed notifications. It additionally cited the registry for falling behind in its ICANN membership charges.
With that in thoughts, a overview of ICANN’s public enforcement exercise suggests two developments: One is that there have been far fewer public compliance and enforcement actions in recent times — even because the variety of new TLDs has expanded dramatically.
The second is that in a majority of instances, the failure of a registry or registrar to pay its annual ICANN membership charges was cited as a motive for a warning letter. A overview of almost two dozen enforcement letters ICANN has despatched to area registrars since 2022 exhibits that failure to pay dues was cited as a motive (or the motive) for the violation no less than 75 p.c of the time.
Piscitello, a former vp of safety at ICANN, stated almost all breach notices despatched out whereas he was at ICANN had been as a result of the registrar owed cash.
“I feel the remaining is simply lipstick to counsel that ICANN’s on prime of DNS Abuse,” Piscitello stated.
KrebsOnSecurity has sought remark from ICANN and can replace this story in the event that they reply.
ICANN stated most of its investigations are resolved and closed by the preliminary casual decision stage, and that tons of of enforcement instances are initiated throughout this stage with the contracted events who’re required to exhibit compliance, grow to be compliant, and/or current and implement remediation plans to stop the recurrence of these enforcement points.
“It is very important have in mind that, previous to issuing any discover of breach to a registrar or registry operator, ICANN Compliance conducts an total contractual compliance ‘well being verify’ of the related contracted occasion,” ICANN stated in a written response to questions. “Throughout this verify, ICANN Compliance proactively critiques the contracted occasion’s compliance with obligations throughout the agreements and insurance policies. Any extra contractual violation discovered throughout these checks is added to the Discover of Breach. It isn’t unusual for events who did not adjust to contractual obligations (whether or not they’re associated to DNS Abuse, RDDS, or others) to even be in arrears with ICANN charges.”
Replace, 11:49 p.m. ET: Added assertion from ICANN. Clarified Piscitello’s former function at ICANN.