Amazon DataZone has introduced a set of latest knowledge governance capabilities—area models and authorization insurance policies—that allow you to create enterprise unit-level or team-level group and handle insurance policies in accordance with your corporation wants. With the addition of area models, customers can set up, create, search, and discover knowledge property and tasks related to enterprise models or groups. With authorization insurance policies, these area unit customers can set entry insurance policies for creating tasks and glossaries, and utilizing compute assets inside Amazon DataZone.
As an Amazon DataZone administrator, now you can create area models (similar to Gross sales or Advertising and marketing) underneath the top-level area and assign area unit homeowners to additional handle the information workforce’s construction. Amazon DataZone customers can log in to the portal to browse and search the catalog by area models, and subscribe to knowledge produced by particular enterprise models. Moreover, authorization insurance policies will be configured for a site unit allowing actions similar to who can create tasks, metadata types, and glossaries inside their area models. Approved portal customers can then log in to the Amazon DataZone portal and create entities similar to tasks and create metadata types utilizing the approved tasks.
Amazon DataZone allows you to uncover, entry, share, and govern knowledge at scale throughout organizational boundaries, decreasing the undifferentiated heavy lifting of constructing knowledge and analytics instruments accessible to everybody within the group. With Amazon DataZone, knowledge customers like knowledge engineers, knowledge scientists, and knowledge analysts can share and entry knowledge throughout AWS accounts utilizing a unified knowledge portal, permitting them to find, use, and collaborate on this knowledge throughout their groups and organizations. Moreover, knowledge homeowners and knowledge stewards could make knowledge discovery easier by including enterprise context to knowledge whereas balancing entry governance to the information within the UI.
On this put up, we focus on frequent approaches to structuring area models, use circumstances that prospects within the healthcare and life sciences (HCLS) trade encounter, and methods to get began with the brand new area models and authorization insurance policies options from Amazon DataZone.
Approaches to structuring area models
Domains are top-level entities that embody a number of area models as sub-entities, every with particular insurance policies. Organizations can undertake totally different approaches when defining and structuring domains and area models. Some methods align these models with knowledge domains, whereas others observe organizational buildings or strains of enterprise. On this part, we discover a couple of examples of domains, area models, and methods to set up knowledge property and merchandise inside these constructs.
Domains aligned with the group
Area models will be constructed utilizing the organizational construction, strains of companies, or use circumstances. For instance, HCLS organizations sometimes have a spread of domains that embody numerous elements of their operations and providers. Clients are utilizing domains and area models to enhance searchability and findability of knowledge property inside an organized tree-like construction, and allow particular person organizational models to manage their very own authorization insurance policies.
One of many core advantages of organizing entities as area models is to allow search and self-service entry throughout numerous area models. The next are some frequent area models inside the HCLS sector:
- Commercials – Industrial elements of services or products associated to the life sciences and actions similar to market evaluation, product positioning, pricing, distribution, and buyer engagement. There may very well be a number of baby area models, similar to like Contract Analysis Group.
- Analysis and improvement – Pharmaceutical and medical system improvement. Some examples of kid area models embrace drug discovery and scientific trials administration.
- Scientific providers – Hospital and clinic administration. Examples of kid area models embrace doctor and nursing providers.
- Income cycle administration – Affected person billing and claims processing. Examples of kid area models embrace insurance coverage and payer relations.
The next are frequent domains and area models that apply throughout industries:
- Provide chain and logistics – Procurement and stock administration.
- Regulatory compliance and high quality assurance – Compliance with trade particular rules, high quality administration methods, and accreditation.
- Advertising and marketing – Methods, strategies, and practices aimed toward selling merchandise, providers, or concepts to potential prospects. Some examples of kid area models are campaigns and occasions.
- Gross sales – Gross sales course of, key efficiency indicators (KPIs), and metrics.
For instance, certainly one of our prospects, HEMA, makes use of Amazon DataZone as a single resolution for cataloging, discovery, sharing, and governance of their enterprise knowledge throughout enterprise domains.
“The launch of Area Models function is one other step in the direction of the emergence of Amazon DataZone because the main Knowledge Governance resolution for Knowledge Mesh-driven organizations. We are actually in a position to set up Knowledge Belongings underneath particular enterprise models, permitting producers to autonomously handle the lifecycle of their knowledge property, whereas offering end-users an environment friendly and arranged approach to uncover knowledge. Love that every enterprise unit can now have insurance policies that adhere to their very own wants as properly.”
– Tommaso Paracciani, Head of Knowledge & Cloud Platforms at HEMA.
One other buyer, AWS Knowledge Platform, makes use of Amazon DataZone to supply safe, trusted, handy, and quick entry to AWS enterprise knowledge.
“At AWS, our imaginative and prescient is to supply prospects with dependable, safe, and self-service entry to exabyte-scale knowledge whereas guaranteeing knowledge governance and compliance. With Amazon DataZone area models, we’re in a position to set up an enormous and rising variety of datasets to align with the organizational construction of the purchasers my groups serve internally. This simplifies knowledge discovery and helps us set up enterprise models’ knowledge in a hierarchical method for data-driven decision-making at AWS. Amazon DataZone authorization insurance policies coupled with area models allow a robust but versatile means of decentralizing knowledge governance and helps tailor entry insurance policies to particular person enterprise models. With these options, we’re in a position to scale back the undifferentiated heavy carry whereas constructing and managing knowledge merchandise.”
– Arnaud Mauvais, Director of Software program Improvement at AWS.
Domains aligned with knowledge possession
The time period knowledge area is essential inside the realm of knowledge governance. It signifies a definite area or classification of knowledge that a corporation oversees and regulates. Knowledge domains type a foundational pillar in knowledge governance frameworks. The idea of knowledge domains performs a pivotal function in knowledge governance, empowering organizations to systematically construction, administer, and harness their knowledge property. This strategic strategy aligns knowledge assets with enterprise objectives, fostering knowledgeable decision-making processes.
You’ll be able to both outline every knowledge area as a top-level area or outline a top-level knowledge area (for instance, Group) with a number of baby area models, similar to:
- Buyer knowledge – This area unit consists of all knowledge associated to prospects, similar to buyer profiles. A number of different baby area models with insurance policies will be constructed inside buyer area models, similar to buyer interactions and profiles.
- Monetary knowledge – This area unit encompasses knowledge associated to monetary data.
- Human assets knowledge – This area unit consists of employee-related knowledge.
- Product knowledge – This area unit covers knowledge associated to services or products supplied by the group.
Authorization insurance policies for domains and area models
Amazon DataZone area models offer you a strong and versatile knowledge governance resolution tailor-made to your organizational construction. These area models empower particular person enterprise strains or groups to determine their very own authorization insurance policies, enabling self-service governance over crucial actions similar to publishing knowledge property and using compute assets inside Amazon DataZone. The authorization insurance policies enabled by area models assist you to grant granular entry rights to customers and teams, empowering them to handle area models, mission memberships, and creation of content material similar to tasks, metadata types, glossaries and customized asset sorts.
Area governance authorization insurance policies assist organizations keep knowledge privateness, confidentiality, and integrity by controlling and limiting entry to delicate or crucial knowledge. In addition they help data-driven decision-making by ensuring approved customers have acceptable entry to the data they should carry out their duties. Equally, authorization insurance policies will help organizations govern the administration of organizational domains, collaboration, and metadata. These insurance policies will help outline roles like knowledge governance proprietor, knowledge product homeowners, and knowledge stewards.
Moreover, these insurance policies facilitate metadata administration, glossary administration, and area possession, so knowledge governance practices are aligned with the precise wants and necessities of every enterprise line or workforce. By utilizing area models and their related authorization insurance policies, organizations can decentralize knowledge governance tasks whereas sustaining a constant and managed strategy to knowledge asset and metadata administration. This distributed governance mannequin promotes possession and accountability inside particular person enterprise strains, fostering a tradition of knowledge stewardship and enabling extra agile and responsive knowledge administration practices.
Use circumstances for area models
Amazon DataZone area models assist prospects in numerous industries securely and effectively govern their knowledge, collaborate on necessary knowledge administration initiatives, and assist in complying with related rules. These capabilities are notably helpful for patrons in industries with strict knowledge privateness and safety necessities, similar to HCLS, monetary providers, and the general public sector. Amazon DataZone area models allow you to keep up management over your knowledge whereas facilitating seamless collaboration and serving to you adhere to rules like Well being Insurance coverage Portability and Accountability Act (HIPAA), Basic Knowledge Safety Regulation (GDPR), and others particular to your trade.
The next are key advantages of Amazon DataZone area models for HCLS prospects:
- Safe and compliant knowledge sharing – Amazon DataZone area models assist present a safe mechanism so that you can share delicate knowledge, similar to protected well being data (PHI) and personally identifiable data (PII). This helps organizations with regulatory necessities keep the privateness and safety of their knowledge.
- Scalable and versatile knowledge administration – Amazon DataZone area models provide a scalable and versatile knowledge administration resolution that allows you to handle and curate your knowledge, whereas additionally enabling environment friendly knowledge discovery and entry.
- Streamlined collaboration and governance – The platform supplies a centralized and managed surroundings for groups to collaborate on data-driven tasks. It permits efficient knowledge governance, permitting you to outline and implement insurance policies, present readability on who has entry to knowledge, and keep management over delicate data.
- Granular authorization insurance policies – Amazon DataZone area models assist you to outline and implement fine-grained authorization insurance policies, keep tight management over your knowledge, and streamline data-driven collaboration and governance throughout your groups.
Resolution overview
On the AWS Administration Console, the administrator (AWS account person) creates the Amazon DataZone area. Because the creator of the area, they will select so as to add different single sign-on (SSO) and AWS Identification and Entry Administration (IAM) customers as homeowners to handle the area. Below the area, area models (similar to Gross sales, Advertising and marketing, and Finance) will be created to replicate a hierarchy that aligns with the group’s knowledge ecosystem. Possession of those area models will be assigned to enterprise leaders, who might broaden a hierarchy representing their knowledge groups and later set insurance policies that allow customers and tasks to carry out particular actions. With the area construction in place, you possibly can set up your property underneath acceptable area models. The group of property to area models begins with tasks being assigned to a site unit at time of creation and property then being cataloged inside the mission. Catalog customers then browse the area hierarchy to seek out property associated to particular enterprise features. They’ll additionally seek for property utilizing a site unit as a search aspect.
Area models set the muse for a way authorization insurance policies allow customers to carry out actions in Amazon DataZone, similar to who can create and be part of tasks. Amazon DataZone creates a set of managed authorization insurance policies for each area unit, and area unit homeowners create grants inside a coverage to customers and tasks.
There are two Amazon DataZone entities which have insurance policies created on them. The primary is a area unit the place the homeowners can determine who might carry out actions similar to creating domains, tasks, becoming a member of tasks, creating metadata types, and so forth. The insurance policies have an choice to cascade the grant down by baby area models. These insurance policies are managed by the Amazon DataZone portal, and their grants will be utilized to 2 principal sorts:
- Person-based insurance policies – These insurance policies grant customers (IAM, SSO, and SSO teams) permission to carry out an motion (similar to create area models and tasks, be part of tasks, and take possession of area models and tasks)
- Undertaking-based insurance policies – These insurance policies grant a mission permission to carry out an motion (similar to create metadata types, glossaries, or customized asset sorts)
The second Amazon DataZone entity is a blueprint (defines the instruments and providers for Amazon DataZone environments), the place a knowledge platform person (AWS account person) who owns the Amazon DataZone blueprint can determine which tasks use their assets by surroundings profile creation on the Amazon DataZone portal. There are two approaches to specify which tasks can use the blueprint to create an surroundings profile:
- Account customers can use area models as a delegation mechanism to move the belief of utilizing the blueprint to a enterprise chief (area unit proprietor) on the Amazon DataZone portal
- Account customers can instantly grant a selected mission permission to make use of the blueprint
These insurance policies will be managed by the console and Amazon DataZone portal.
The next determine is an instance area construction for the ABC Corp area. Area models are created underneath the ABC Corp area with area unit homeowners assigned. Authorization insurance policies are utilized for every area unit and dictate the actions customers and tasks can carry out.
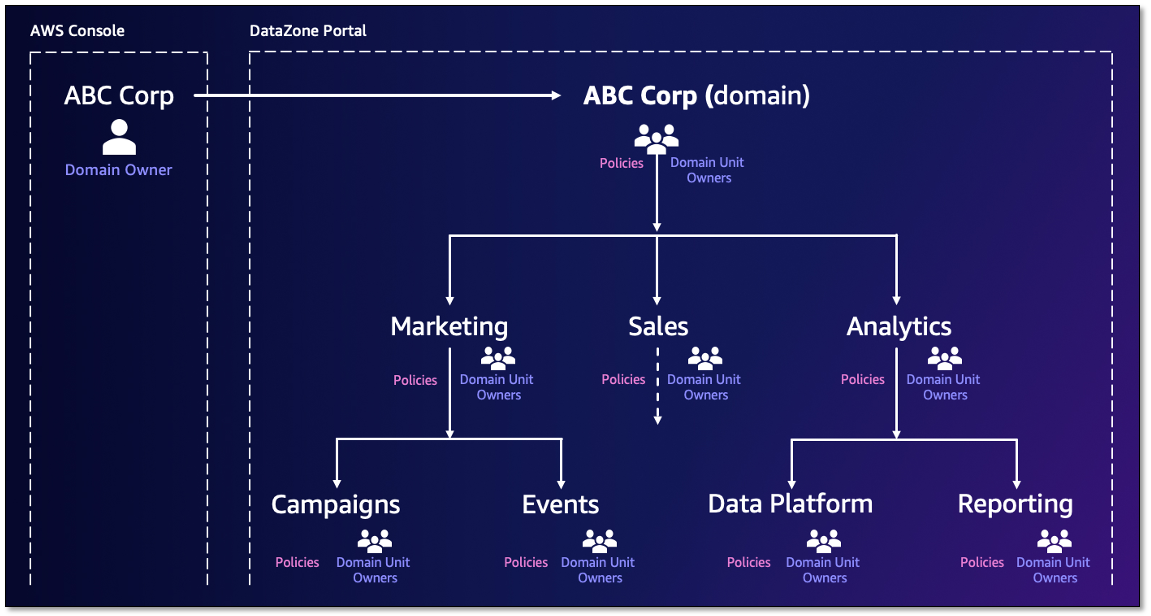
For extra details about Amazon DataZone elements, check with Amazon DataZone terminology and ideas.
Within the following sections, we stroll by the steps to get began with the information administration governance capabilities in Amazon DataZone.
Create an Amazon DataZone area
With Amazon DataZone, directors log in to the console and create an Amazon DataZone area. Extra area unit homeowners will be added to assist handle the area. For extra data, check with Managing Amazon DataZone domains and person entry.
Create area models to characterize your corporation models
To create a site unit, full the next steps:
- Log in to the DataZone knowledge portal and select Area in toolbar to view your area models.
- Because the area unit proprietor, select Create Area Unit.
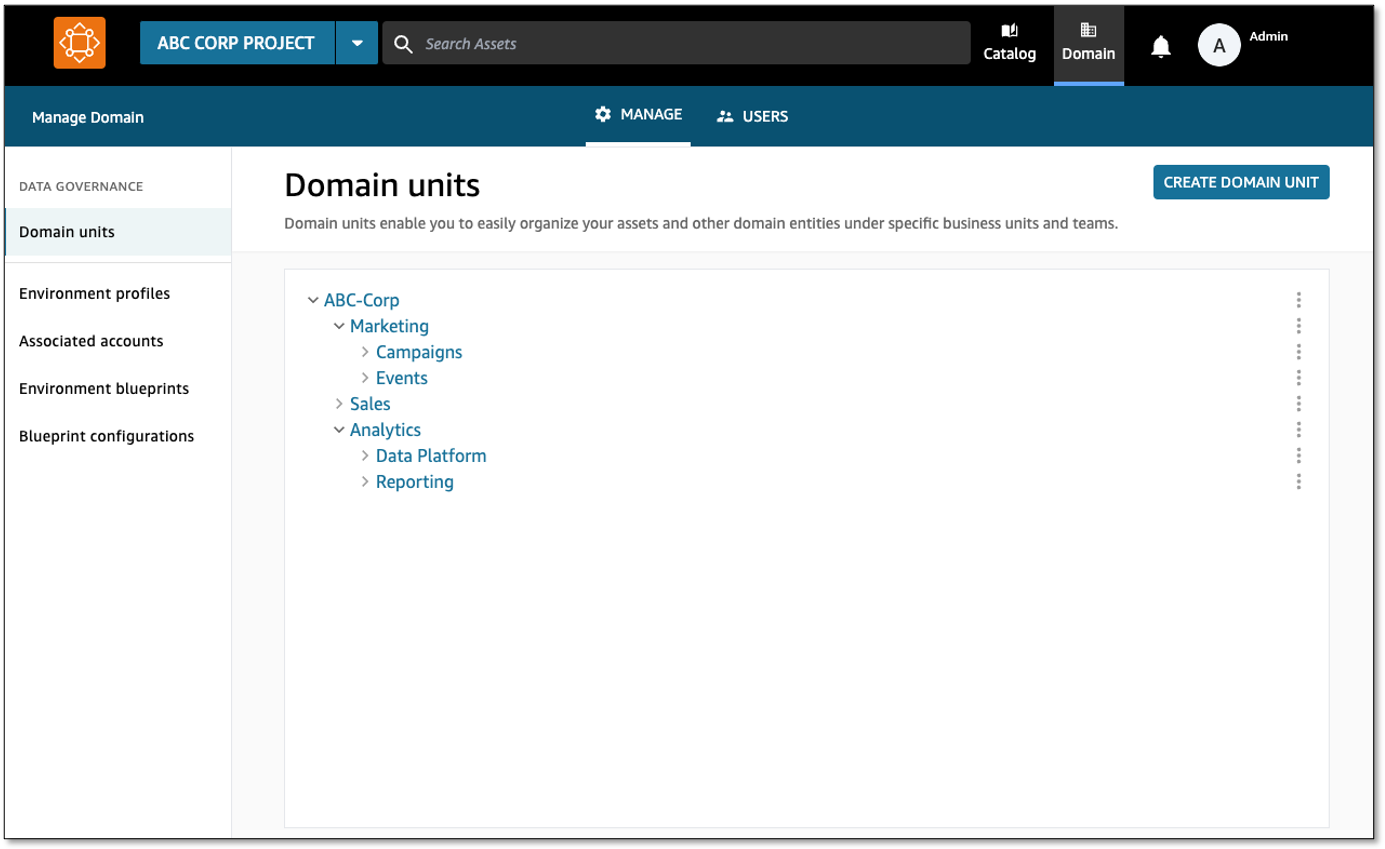
- Present your area unit particulars (representing totally different strains of enterprise).
- You’ll be able to create extra area models in a nested style.
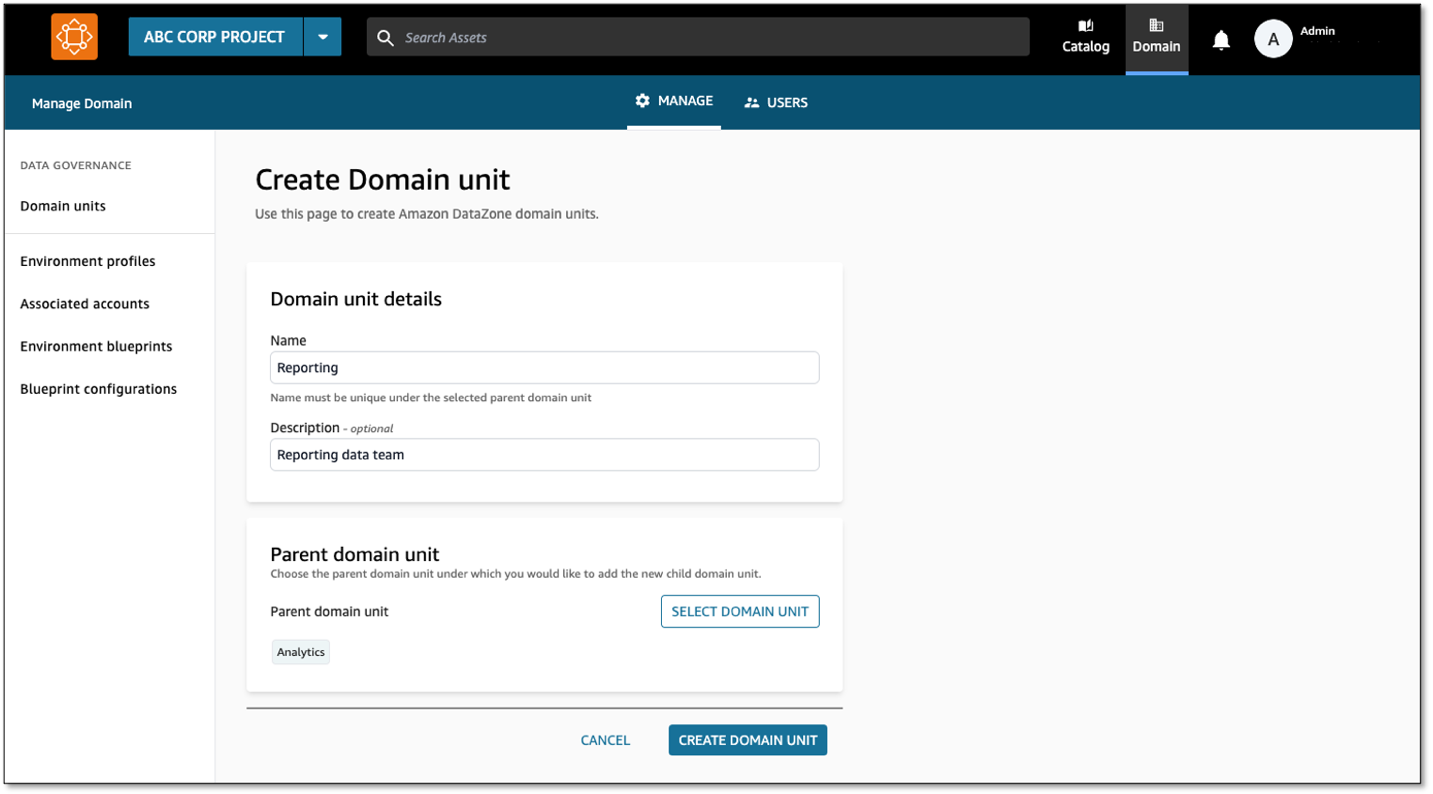
- For every area unit, assign homeowners to handle the area unit and its authorization insurance policies.
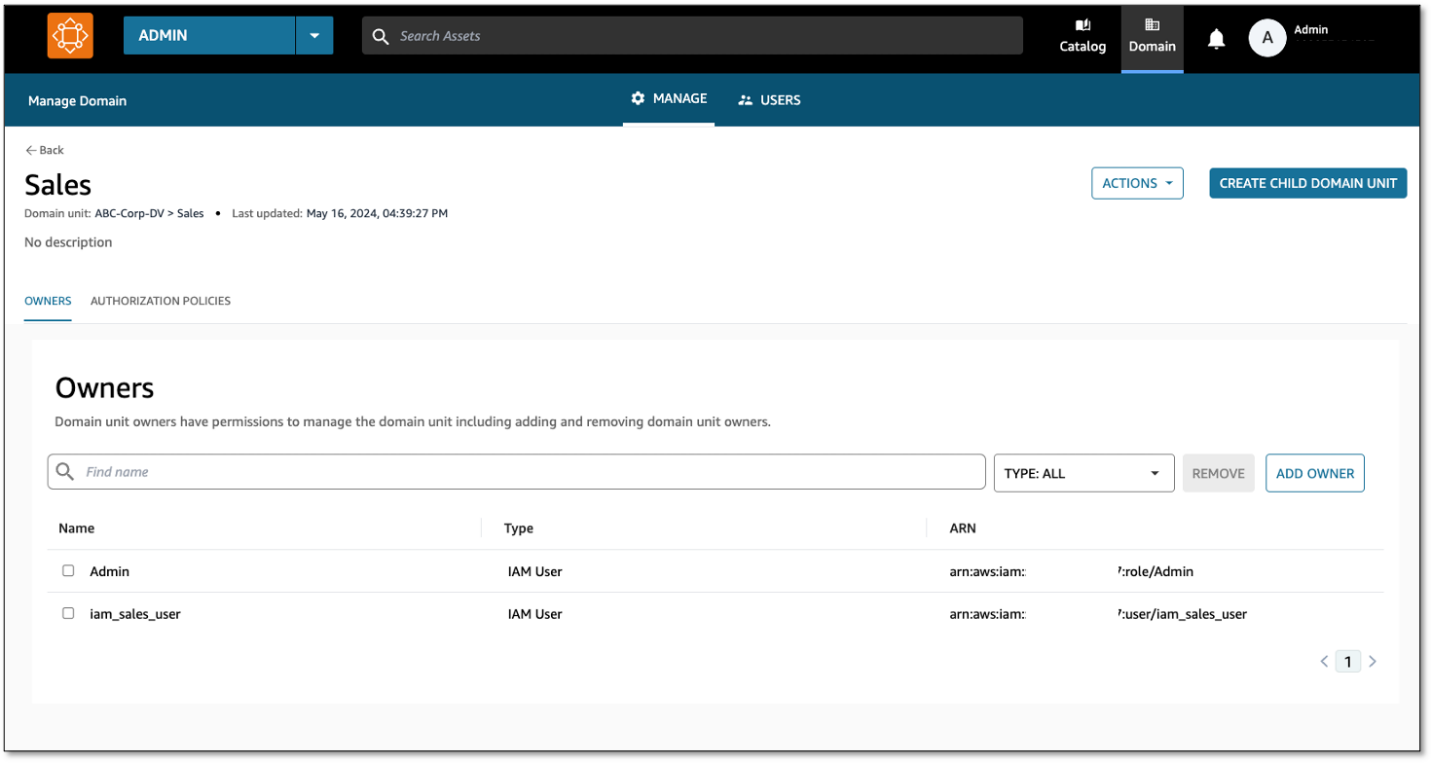
Apply authorization insurance policies so area models can self-govern
Amazon DataZone managed authorization insurance policies can be found for each area unit, and area unit homeowners can grant entry by that coverage to customers and tasks. Insurance policies are both user-based (granted to customers) or project-based (granted to tasks).
- On the Authorization Insurance policies tab of a site unit, grant authorization insurance policies to customers or tasks letting them carry out sure actions. For this instance, we select Undertaking creation coverage for the Gross sales area.
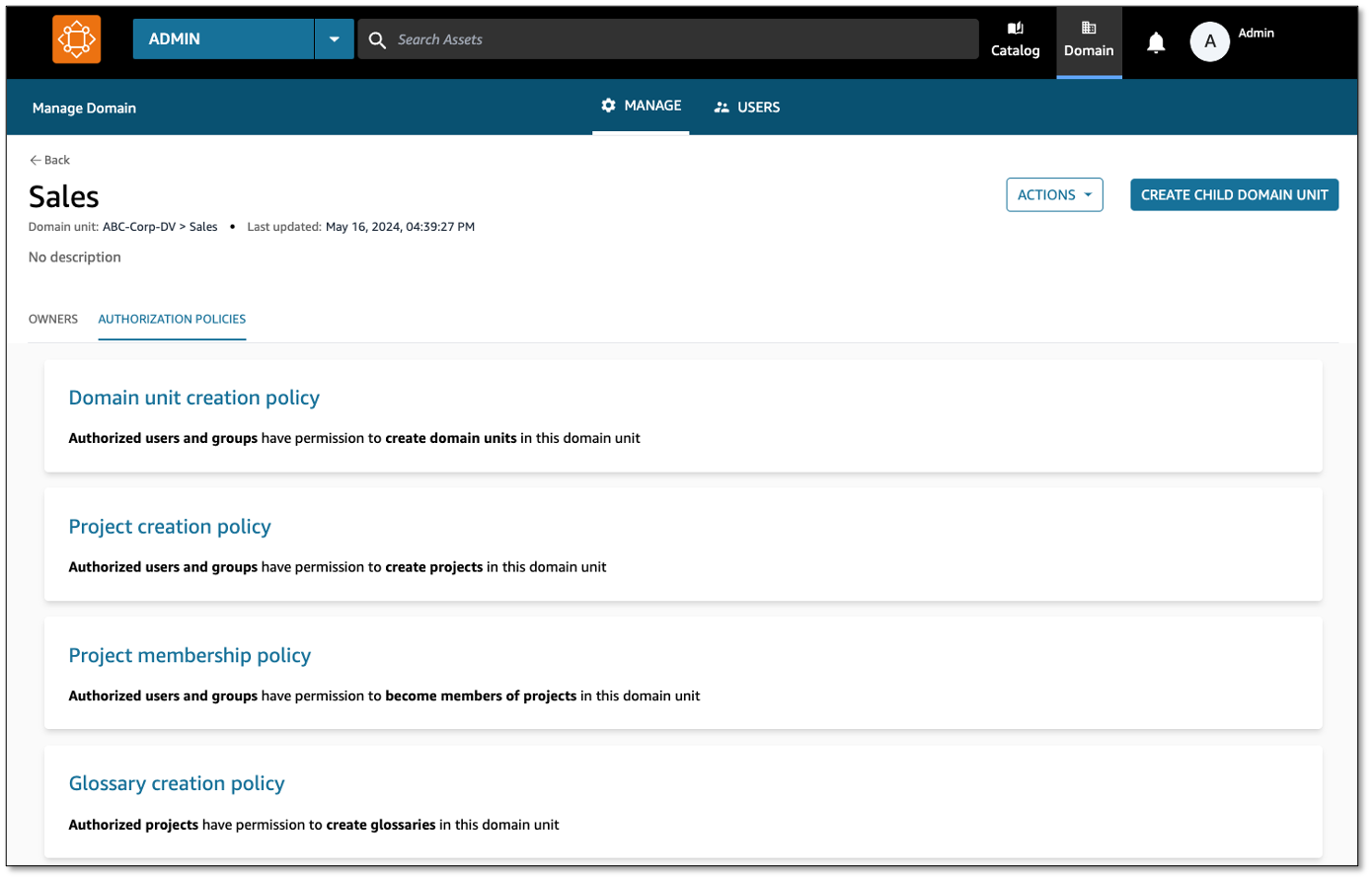
- Select Add Coverage Grant so as to add both choose customers and teams, all customers, or all teams.
With this, a Gross sales workforce member can log in to the information portal and create tasks underneath the Gross sales area.
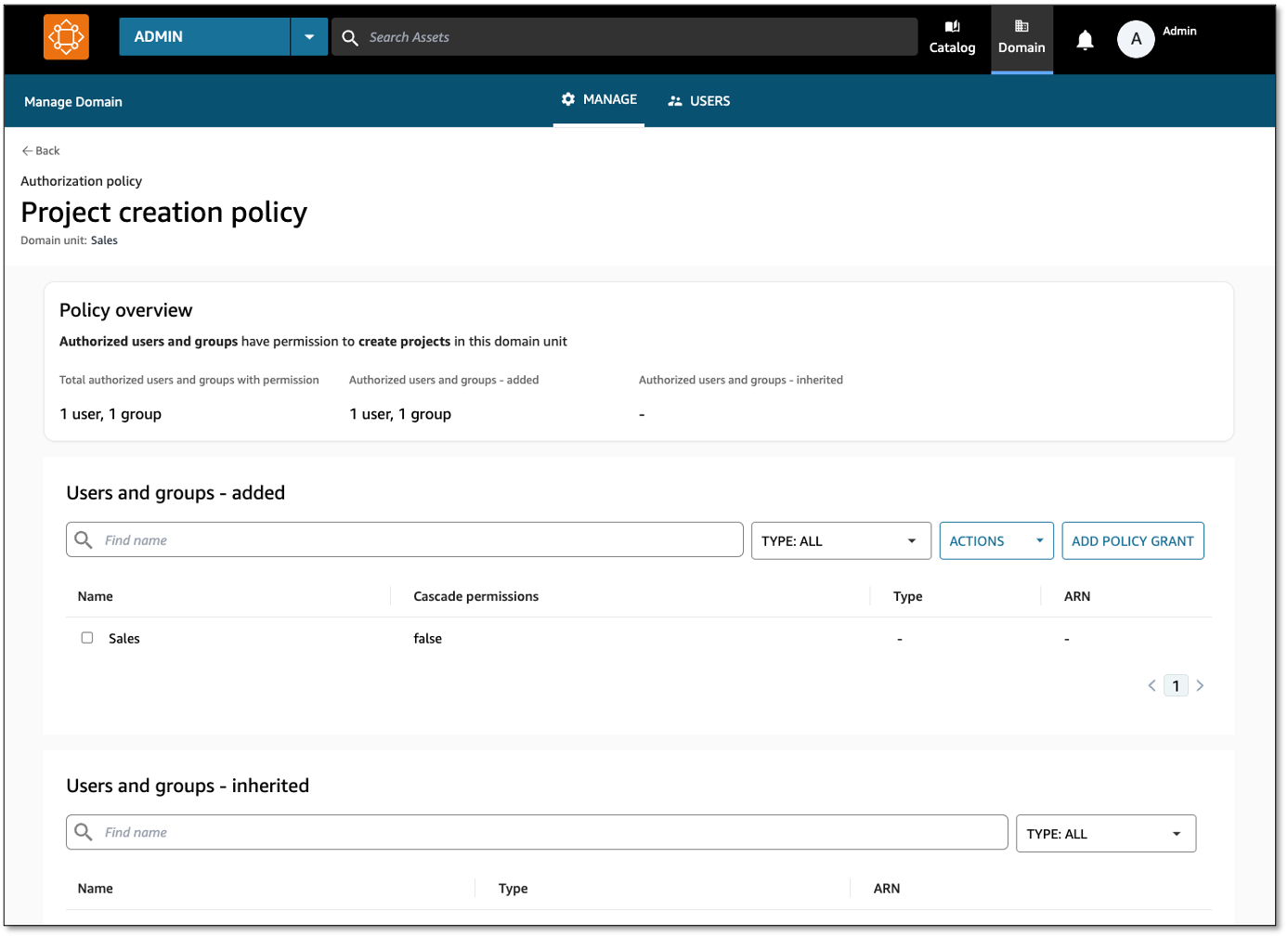
Conclusion
On this put up, we mentioned frequent approaches to structuring area models, use circumstances that prospects within the HCLS trade encounter, and methods to get began with the brand new area models and authorization insurance policies options from Amazon DataZone.
Area models present clear separation between knowledge areas, making the discoverability of knowledge environment friendly for customers. Authorization insurance policies, together with area models, present the governance layer controlling entry to the information and supply management over how the information is cataloged. Collectively, Amazon DataZone area models and authorization insurance policies make group and governance potential throughout your knowledge.
Amazon DataZone area models and authorization insurance policies can be found in all AWS Areas the place Amazon DataZone is on the market. To study extra, check with Working with area models.
In regards to the Authors
David Victoria is a Senior Technical Product Supervisor with Amazon DataZone at AWS. He focuses on enhancing administration and governance capabilities wanted for patrons to help their analytics methods. He’s obsessed with serving to prospects notice essentially the most worth from their knowledge in a safe, ruled method. Outdoors of labor, he enjoys climbing, touring, and making his new child child giggle.
Nora O Sullivan is a Senior Options Architect at AWS. She focuses on serving to HCLS prospects select the precise AWS providers for his or her knowledge and analytics wants to allow them to derive worth from their knowledge. Outdoors of labor, she enjoys {golfing} and discovering new wines and authors.
Navneet Srivastava, a Principal Specialist and Analytics Technique Chief, develops strategic plans for constructing an end-to-end analytical technique for big biopharma, healthcare, and life sciences organizations. Navneet is accountable for serving to life sciences organizations and healthcare firms deploy knowledge governance and analytical functions, digital medical information, units, and AI/ML-based functions whereas educating prospects about methods to construct safe, scalable, and cost-effective AWS options. His experience spans throughout knowledge analytics, knowledge governance, AI, ML, huge knowledge, and healthcare-related applied sciences.