Let’s examine the right way to do it in phases: we begin with the next take a look at that
tries to compile the template. In Go we use the usual html/template
bundle.
Go
func Test_wellFormedHtml(t *testing.T) { templ := template.Should(template.ParseFiles("index.tmpl")) _ = templ }
In Java, we use jmustache
as a result of it is quite simple to make use of; Freemarker or
Velocity are different frequent selections.
Java
@Take a look at void indexIsSoundHtml() { var template = Mustache.compiler().compile( new InputStreamReader( getClass().getResourceAsStream("/index.tmpl"))); }
If we run this take a look at, it can fail, as a result of the index.tmpl
file does
not exist. So we create it, with the above damaged HTML. Now the take a look at ought to move.
Then we create a mannequin for the template to make use of. The applying manages a todo-list, and
we are able to create a minimal mannequin for demonstration functions.
Go
func Test_wellFormedHtml(t *testing.T) {
templ := template.Should(template.ParseFiles("index.tmpl"))
mannequin := todo.NewList()
_ = templ
_ = mannequin
}
Java
@Take a look at
void indexIsSoundHtml() {
var template = Mustache.compiler().compile(
new InputStreamReader(
getClass().getResourceAsStream("/index.tmpl")));
var mannequin = new TodoList();
}
Now we render the template, saving the leads to a bytes buffer (Go) or as a String
(Java).
Go
func Test_wellFormedHtml(t *testing.T) {
templ := template.Should(template.ParseFiles("index.tmpl"))
mannequin := todo.NewList()
var buf bytes.Buffer
err := templ.Execute(&buf, mannequin)
if err != nil {
panic(err)
}
}
Java
@Take a look at
void indexIsSoundHtml() {
var template = Mustache.compiler().compile(
new InputStreamReader(
getClass().getResourceAsStream("/index.tmpl")));
var mannequin = new TodoList();
var html = template.execute(mannequin);
}
At this level, we wish to parse the HTML and we anticipate to see an
error, as a result of in our damaged HTML there’s a div
ingredient that
is closed by a p
ingredient. There’s an HTML parser within the Go
normal library, however it’s too lenient: if we run it on our damaged HTML, we do not get an
error. Fortunately, the Go normal library additionally has an XML parser that may be
configured to parse HTML (due to this Stack Overflow reply)
Go
func Test_wellFormedHtml(t *testing.T) {
templ := template.Should(template.ParseFiles("index.tmpl"))
mannequin := todo.NewList()
// render the template right into a buffer
var buf bytes.Buffer
err := templ.Execute(&buf, mannequin)
if err != nil {
panic(err)
}
// examine that the template will be parsed as (lenient) XML
decoder := xml.NewDecoder(bytes.NewReader(buf.Bytes()))
decoder.Strict = false
decoder.AutoClose = xml.HTMLAutoClose
decoder.Entity = xml.HTMLEntity
for {
_, err := decoder.Token()
swap err {
case io.EOF:
return // We're performed, it is legitimate!
case nil:
// do nothing
default:
t.Fatalf("Error parsing html: %s", err)
}
}
}
This code configures the HTML parser to have the best stage of leniency
for HTML, after which parses the HTML token by token. Certainly, we see the error
message we wished:
--- FAIL: Test_wellFormedHtml (0.00s) index_template_test.go:61: Error parsing html: XML syntax error on line 4: sudden finish ingredient
In Java, a flexible library to make use of is jsoup:
Java
@Take a look at
void indexIsSoundHtml() {
var template = Mustache.compiler().compile(
new InputStreamReader(
getClass().getResourceAsStream("/index.tmpl")));
var mannequin = new TodoList();
var html = template.execute(mannequin);
var parser = Parser.htmlParser().setTrackErrors(10);
Jsoup.parse(html, "", parser);
assertThat(parser.getErrors()).isEmpty();
}
And we see it fail:
java.lang.AssertionError: Anticipating empty however was:<[<1:13>: Unexpected EndTag token [] when in state [InBody],
Success! Now if we copy over the contents of the TodoMVC
template to our index.tmpl
file, the take a look at passes.
The take a look at, nonetheless, is simply too verbose: we extract two helper capabilities, in
order to make the intention of the take a look at clearer, and we get
Go
func Test_wellFormedHtml(t *testing.T) { mannequin := todo.NewList() buf := renderTemplate("index.tmpl", mannequin) assertWellFormedHtml(t, buf) }
Java
@Take a look at void indexIsSoundHtml() { var mannequin = new TodoList(); var html = renderTemplate("/index.tmpl", mannequin); assertSoundHtml(html); }
Stage 2: testing HTML construction
What else ought to we take a look at?
We all know that the seems to be of a web page can solely be examined, in the end, by a
human how it’s rendered in a browser. Nevertheless, there may be typically
logic in templates, and we wish to have the ability to take a look at that logic.
One may be tempted to check the rendered HTML with string equality,
however this method fails in observe, as a result of templates comprise quite a lot of
particulars that make string equality assertions impractical. The assertions
grow to be very verbose, and when studying the assertion, it turns into troublesome
to grasp what it’s that we’re making an attempt to show.
What we’d like
is a method to claim that some elements of the rendered HTML
correspond to what we anticipate, and to ignore all the small print we do not
care about. A technique to do that is by working queries with the CSS selector language:
it’s a highly effective language that enables us to pick out the
parts that we care about from the entire HTML doc. As soon as we now have
chosen these parts, we (1) depend that the variety of ingredient returned
is what we anticipate, and (2) that they comprise the textual content or different content material
that we anticipate.
The UI that we’re imagined to generate seems to be like this:
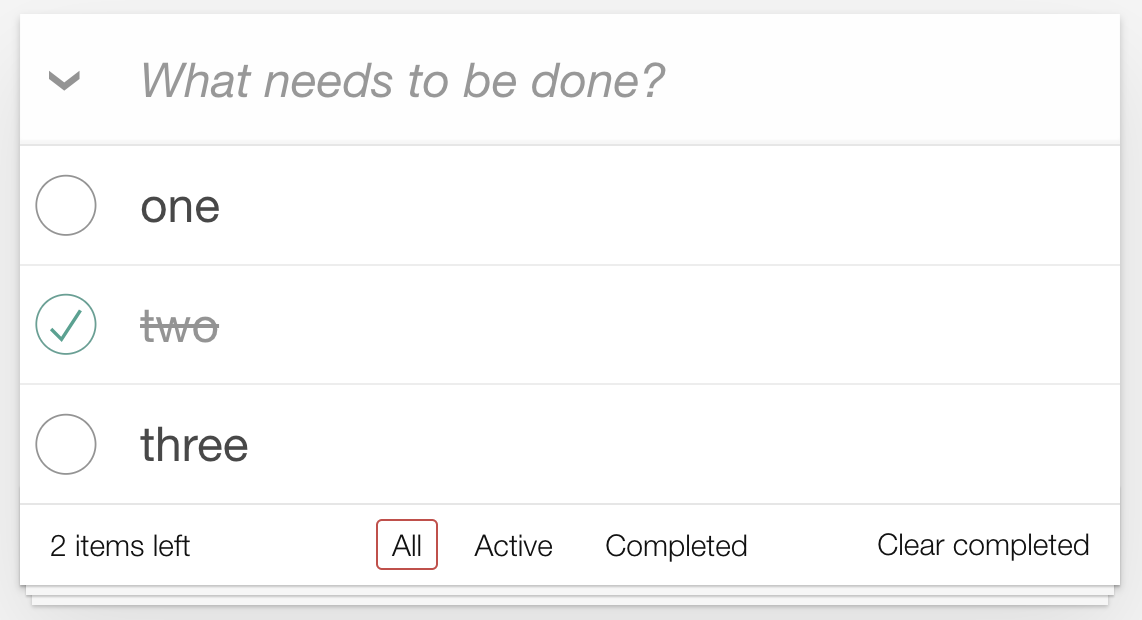
There are a number of particulars which can be rendered dynamically:
- The variety of gadgets and their textual content content material change, clearly
- The fashion of the todo-item adjustments when it is accomplished (e.g., the
second) - The “2 gadgets left” textual content will change with the variety of non-completed
gadgets - One of many three buttons “All”, “Energetic”, “Accomplished” shall be
highlighted, relying on the present url; as an example if we determine that the
url that exhibits solely the “Energetic” gadgets is/lively
, then when the present url
is/lively
, the “Energetic” button must be surrounded by a skinny crimson
rectangle - The “Clear accomplished” button ought to solely be seen if any merchandise is
accomplished
Every of this issues will be examined with the assistance of CSS selectors.
This can be a snippet from the TodoMVC template (barely simplified). I
haven’t but added the dynamic bits, so what we see right here is static
content material, supplied for instance:
index.tmpl