In immediately’s data-driven world, seamless integration and transformation of information throughout numerous sources into actionable insights is paramount. AWS Glue is a serverless knowledge integration service that helps analytics customers to find, put together, transfer, and combine knowledge from a number of sources for analytics, machine studying (ML), and software growth. With AWS Glue, you possibly can uncover and hook up with a whole bunch of numerous knowledge sources and handle your knowledge in a centralized knowledge catalog. It allows you to visually create, run, and monitor extract, remodel, and cargo (ETL) pipelines to load knowledge into your knowledge lakes.
On this weblog put up, we discover the right way to use the SFTP Connector for AWS Glue from the AWS Market to effectively course of knowledge from Safe File Switch Protocol (SFTP) servers into Amazon Easy Storage Service (Amazon S3), additional empowering your knowledge analytics and insights.
Introducing the SFTP connector for AWS Glue
The SFTP connector for AWS Glue simplifies the method of connecting AWS Glue jobs to extract knowledge from SFTP storage and to load knowledge into SFTP storage. This connector offers complete entry to SFTP storage, facilitating cloud ETL processes for operational reporting, backup and catastrophe restoration, knowledge governance, and extra.
Answer overview
On this instance, you employ AWS Glue Studio to connect with an SFTP server, then enrich that knowledge and add it to Amazon S3. The SFTP connector is used to handle the connection to the SFTP server. You’ll load the occasion knowledge from the SFTP website, be a part of it to the venue knowledge saved on Amazon S3, apply transformations, and retailer the info in Amazon S3. The occasion and venue recordsdata are from the TICKIT dataset.
The TICKIT dataset tracks gross sales exercise for the fictional TICKIT web site, the place customers purchase and promote tickets on-line for sporting occasions, exhibits, and live shows. On this dataset, analysts can determine ticket motion over time, success charges for sellers, and best-selling occasions, venues, and seasons.
For this instance, you employ AWS Glue Studio to develop a visible ETL pipeline. This pipeline will learn knowledge from an SFTP server, carry out transformations, after which load the reworked knowledge into Amazon S3. The next diagram illustrates this structure.
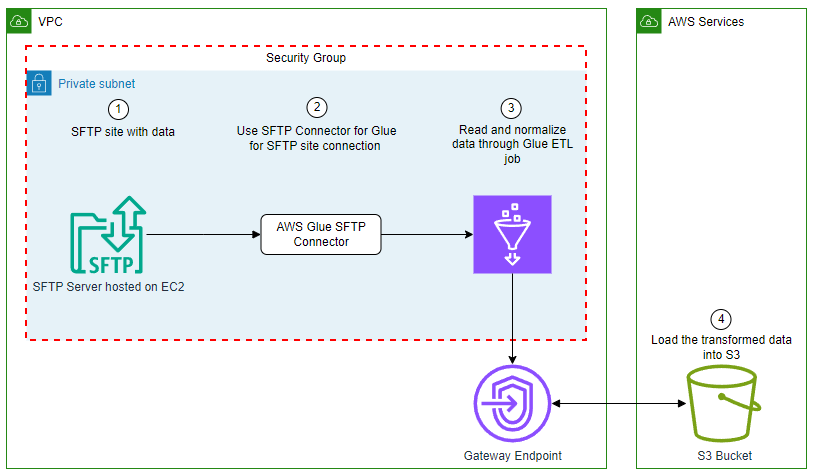
By the top of this put up, your visible ETL job will resemble the next screenshot.
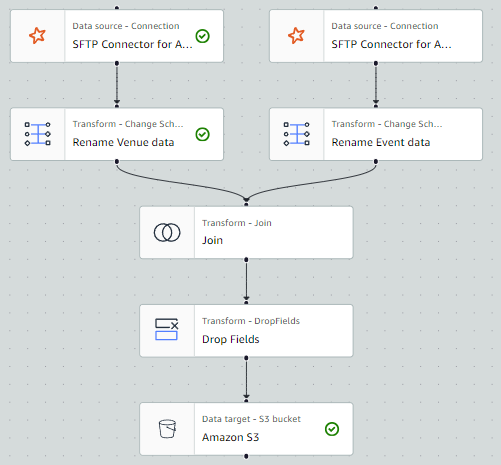
Conditions
For this resolution, you want the next:
- Subscribe to the SFTP Connector for AWS Glue within the AWS Market.
- Entry to an SFTP server with permissions to add and obtain knowledge.
- If the SFTP server is hosted on Amazon Elastic Compute Cloud (Amazon EC2), we advocate that the community communication between the SFTP server and the AWS Glue job occurs inside the digital personal cloud (VPC) as pictured within the previous structure diagram. Working your Glue job inside a VPC and safety group might be mentioned additional within the steps to create the AWS Glue job.
- If the SFTP server is hosted inside your on-premises community, we advocate that the community communication between the SFTP server and the Glue job occurs by means of VPN or AWS DirectConnect.
- Entry to an S3 bucket or the permissions to create an S3 bucket. We advocate that you simply hook up with that bucket utilizing a gateway endpoint. This may permit you to hook up with your S3 bucket instantly out of your VPC. If you’ll want to create an S3 bucket to retailer the outcomes, full the next steps:
- On the Amazon S3 console, select Buckets within the navigation pane.
- Select Create bucket.
- For Identify, enter a globally distinctive identify in your bucket; for instance,
tickit-use1-
.
- Select Create bucket.
- For this demonstration, create a folder with the identify tickit in your S3 bucket.
- Create the gateway endpoint.
- Create an AWS Id and Entry Administration (IAM) function for the AWS Glue ETL job. You could specify an IAM function for the job to make use of. The function should grant entry to all sources utilized by the job, together with Amazon S3 (for any sources, targets, scripts, and non permanent directories) and AWS Secrets and techniques Supervisor. For directions, see Configure an IAM function in your ETL job.
Load dataset to SFTP website
Load the allevents_pipe.txt file and venue_pipe.txt file from the TICKIT dataset to your SFTP server.
Retailer SFTP server sign-in credentials
An AWS Glue connection is a Knowledge Catalog object that shops connection data, reminiscent of URI strings and placement to credentials which can be saved in a Secrets and techniques Supervisor secret.
To retailer the SFTP server username and password in Secrets and techniques Supervisor, full the next steps:
- On the Secrets and techniques Supervisor console, select Secrets and techniques within the navigation pane.
- Select Retailer a brand new secret.
- Choose Different sort of secret.
- Enter host as Secret key and your SFTP server’s IP deal with (for instance,
153.47.122
) because the Secret worth, then select Add row.
- Enter the username as Secret key and your SFTP username as Secret worth, then select Add row.
- Enter password as Secret key and your SFTP password as Secret worth, then select Add row.
- Enter keyS3Uri as Secret Key and the Amazon S3 location of your SFTP secret key file as Secret worth
Notice: Secret Worth is the complete S3 path the place the SFTP server key file is saved. For instance:s3://sftp-bucket-johndoe123/id_rsa
.
- For Secret identify, enter a descriptive identify, then select Subsequent.
- Select Subsequent to maneuver to the overview step, then select Retailer.
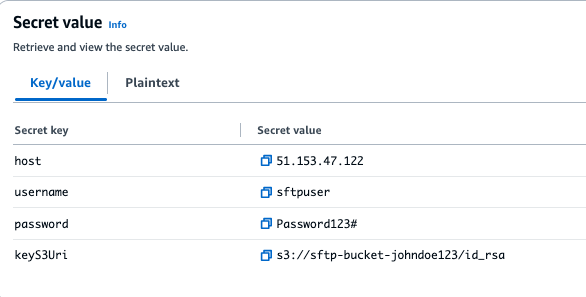
Create a connection to the SFTP server in AWS Glue
Full the next steps to create your connection to the SFTP server.
- On the AWS Glue console, below Knowledge Catalog within the navigation pane, select Connections.
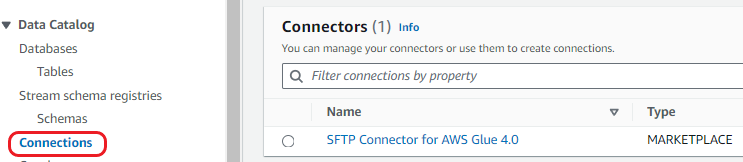
- Choose the SFTP connector for AWS Glue 4.0. Then select Create connection.
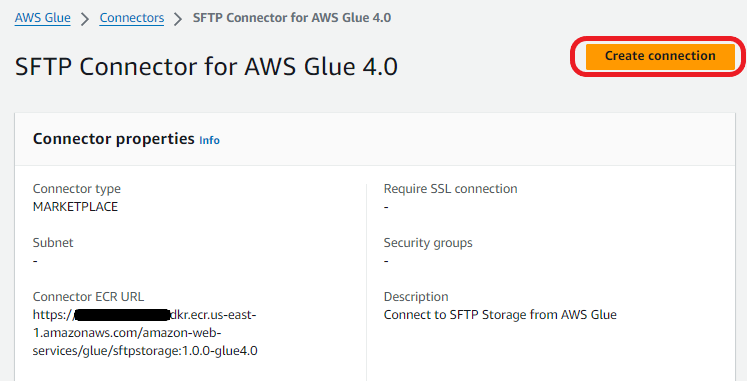
- Enter a reputation for the connection after which, below Connection entry, select the Secrets and techniques Supervisor secret you created for you SFTP server credentials.
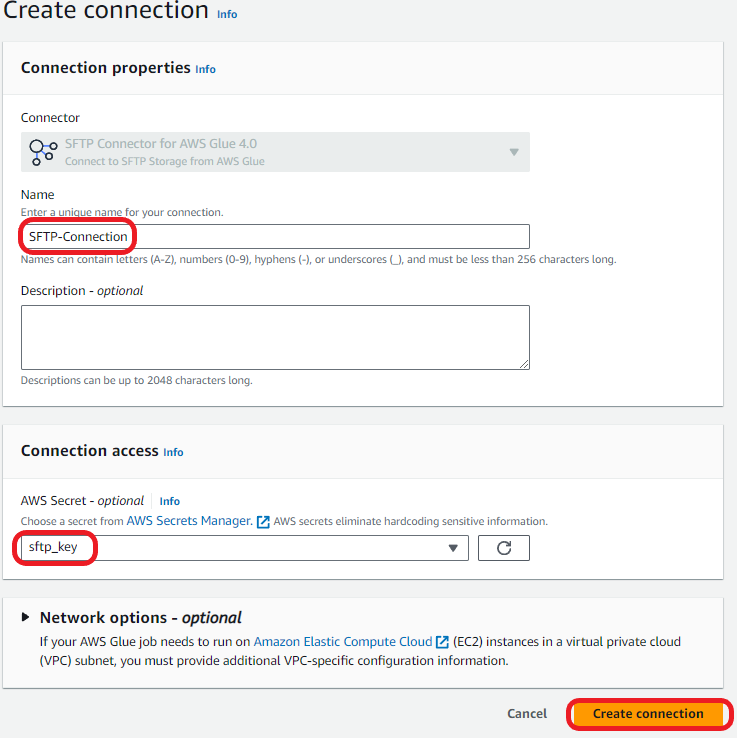
Create a connection to the VPC in AWS Glue
An information connection is used to ascertain community connectivity between the VPC and the AWS Glue job. To create the VPC connection, full the next steps.
- On the AWS Glue console web page, click on on Knowledge Connections location on the left aspect menu.
- Click on the Create connection button within the Connections panel.

- Choose Community
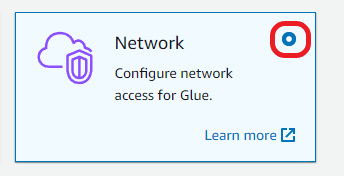
- Choose the VPC, Subnet, and Safety Group that your SFTP server resides in. Click on Subsequent.
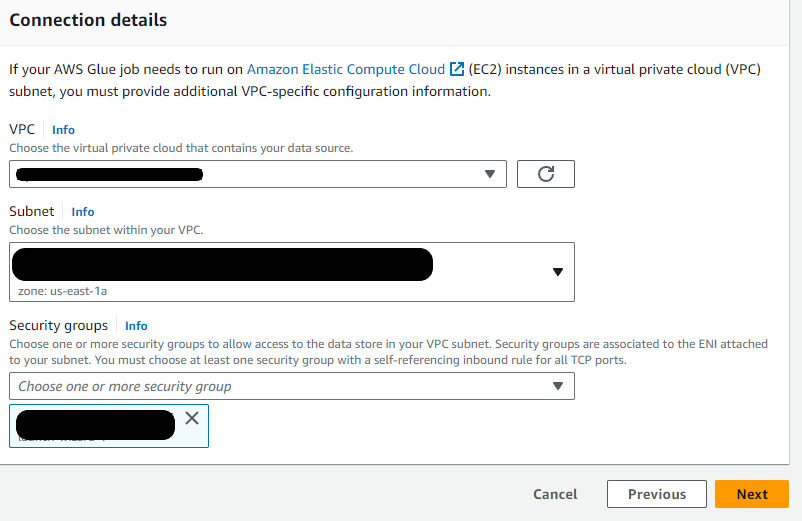
- Identify the connection SFTP VPC Join after which click on
Deploy the answer
Now that we accomplished the stipulations, we’re going to setup the AWS Glue Studio job for this resolution. We’ll create a glue studio job, add occasions and venue knowledge from the SFTP server, perform knowledge transformations and cargo reworked knowledge to s3.
Create your AWS Glue Studio job:
- On the AWS Glue console, below ETL Jobs within the navigation pane, select Visible ETL.
- Choose Visible ETL within the central pane.
- Select the pencil icon to enter a reputation in your job.
- Select the Job particulars tab.
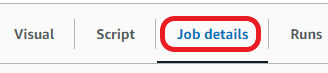
- Scroll right down to and choose Superior properties and develop.
- Scroll to Connections and choose SFTP VPC Join.
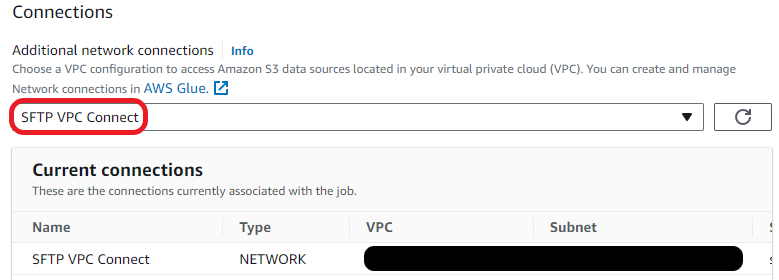
- Select Visible to return to the workflow editor web page.
Add the occasions knowledge from the SFTP server as your first knowledge set:
- Select Add nodes and choose SFTP Connector for AWS Glue 4.0 on the Sources
- Enter the next for Knowledge supply properties for:
- Connection: Choose the connection to the SFTP server that you simply created in Create the connection to the SFTP server in AWS Glue.
- Enter the next key-value pairs:
Key |
Worth |
header |
false |
path |
/recordsdata (this ought to be the trail to the occasion file in your SFTP server) |
fileFormat |
csv |
delimiter |
| |
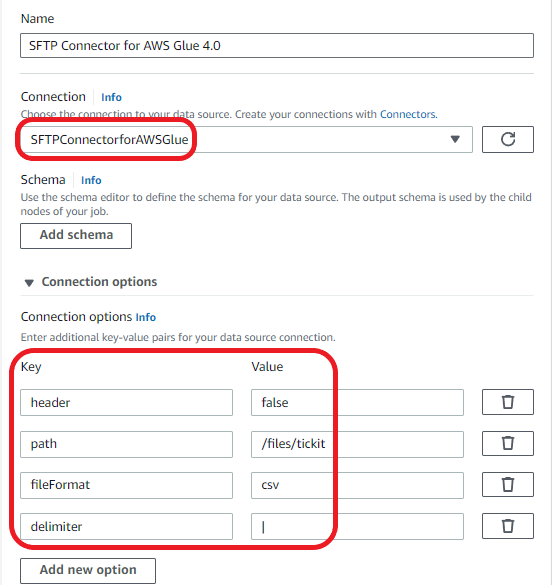
Rename the columns of the Occasion dataset:
- Select Add nodes and select Change Schema on the Transforms
- Enter the next remodel properties:
- For Identify, enter Rename Occasion knowledge.
- For Node dad and mom, choose SFTP Connector for AWS Glue 4.0.
- Within the Change Schema part, map the supply keys to the goal keys:
- col0:
eventid
- col1:
e_venueid
- col2:
catid
- col3:
dateid
- col4:
eventname
- col5:
starttime
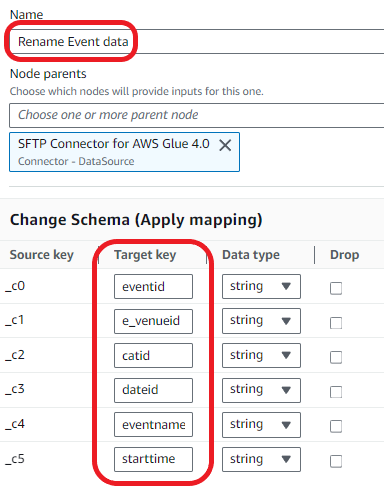
Add the venue_pipe.txt file from the SFTP website:
- Select Add nodes and select SFTP Connector for AWS Glue 4.0 on the Sources
- Enter the next for Knowledge supply properties for:
- Connection: Choose the connection to the SFTP server that you simply created in Create the connection to the SFTP server in AWS Glue.
- Enter the next key-value pairs:
Key |
Worth |
header |
false |
path |
/recordsdata (this ought to be the trail to the venue file in your SFTP website) |
fileFormat |
csv |
delimiter |
| |
Rename the columns of the venue dataset:
- Select Add nodes and select Change Schema on the Transforms
- Enter the next remodel properties:
- For Identify, enter Rename Venue knowledge.
- For Node dad and mom, choose Venue.
- Within the Change Schema part, map the supply keys to the goal keys:
- col0:
venueid
- col1:
venuename
- col2:
venuecity
- col3:
venuestate
- col4:
venueseats
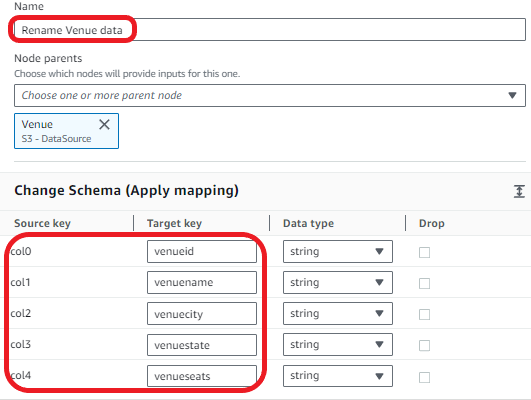
Be part of the venue and occasion datasets.
- Select Add nodes and select Be part of on the Transforms
- Enter the next remodel properties:
- For Identify, enter Be part of.
- For Node dad and mom, choose Rename Venue knowledge and Rename Occasion knowledge.
- For Be part of sort¸ choose Internal be a part of.
- For Be part of situations, choose venueid for Rename Venue knowledge and e_venueid for Rename Occasion knowledge.
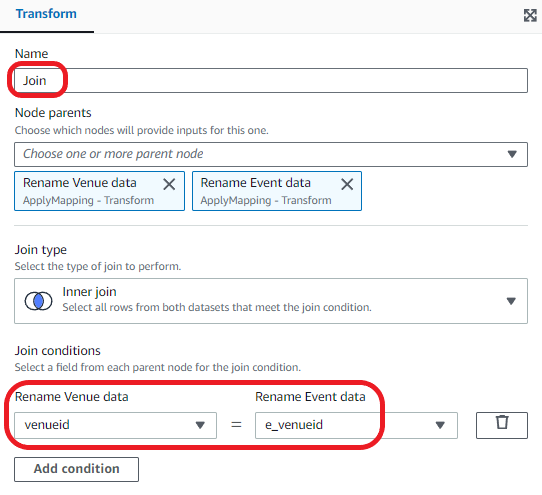
Drop the duplicate area:
- Select Add nodes and select Drop Fields on the Transforms
- Enter the next remodel properties:
- For Identify, enter Drop Fields.
- For Node dad and mom, choose Be part of.
- Within the DropFields part, choose e_venueid.
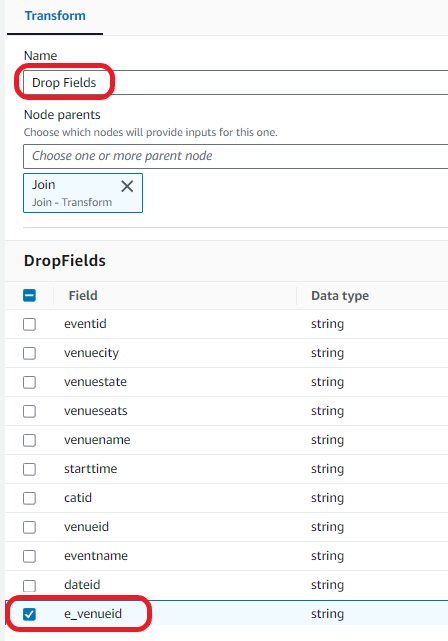
Load the info into your S3 bucket:
- Select Add nodes and select Amazon S3 from the Sources
- Enter the next remodel properties:
- For Node dad and mom, choose Drop Fields.
- For Format, choose CSV.
- For Compression Kind, choose None.
- For S3 Goal Location, select your S3 bucket and enter your required file identify adopted by a slash (/).
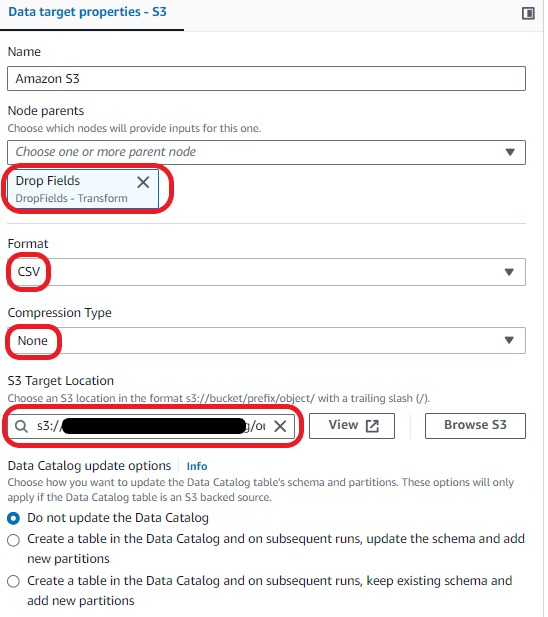
Now you can save and run your AWS Glue visible ETL Job. Run the job after which go to the Runs tab to watch its progress. After the job has accomplished, the Run standing will change to Succeeded. The information might be within the goal S3 bucket.
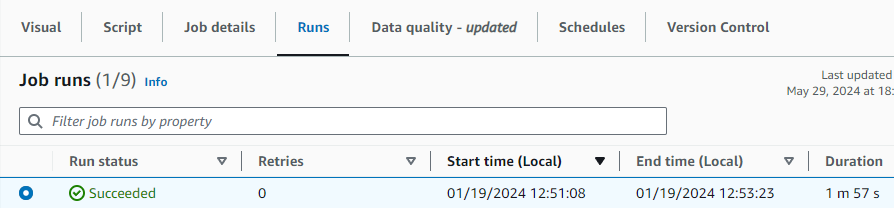
Clear up
To keep away from incurring extra fees brought on by sources created as a part of this put up, ensure you delete the gadgets created within the AWS Account for this put up:
- Delete the Secrets and techniques Supervisor key created for the SFTP connector . credentials.
- Delete the SFTP connector.
- Unsubscribe from the SFTP Connector in AWS Market.
- Delete the info loaded to the Amazon S3 bucket and the bucket.
- Delete the AWS Glue visible ETL job.
Conclusion
On this weblog put up, we demonstrated the right way to use the SFTP connector for AWS Glue to streamline the processing of information from SFTP servers into Amazon S3. This integration performs a pivotal function in enhancing your knowledge analytics capabilities by providing an environment friendly and simple technique to convey collectively disparate knowledge sources. Whether or not your aim is to research SFTP server knowledge for actionable insights, bolster your reporting mechanisms, or enrich your online business intelligence instruments, this connector ensures a extra streamlined and cost-effective method to attaining your knowledge aims.
You need to use SFTP connector for AWS Glue to simplify the method of connecting AWS Glue jobs to extract knowledge from distant SFTP storage or to load knowledge to distant SFTP storage, whereas performing knowledge cleaning and transformations in-memory as a part of your ETL pipelines. On this weblog put up, we discover this resolution in additional element. Alternatively, AWS Switch Household offers fully-managed and AWS native SFTP connectors to reliably copy giant quantity of recordsdata between distant SFTP sources and Amazon S3. You’ve gotten the choice to design an answer utilizing Switch Household’s fully-managed SFTP connector to repeat recordsdata between distant SFTP servers and their Amazon S3 places with none modifications, after which use Glue’s ETL service for cleaning and transformation of the file knowledge.
For additional particulars on the SFTP connector, see the SFTP Connector for Glue documentation.
In regards to the Authors
Sean Bjurstrom is a Technical Account Supervisor in ISV accounts at Amazon Internet Providers, the place he focuses on Analytics applied sciences and attracts on his background in consulting to help prospects on their analytics and cloud journeys. Sean is enthusiastic about serving to companies harness the facility of information to drive innovation and progress. Exterior of labor, he enjoys working and has participated in a number of marathons.
Seun Akinyosoye is a Sr. Technical Account Supervisor supporting public sector buyer at Amazon Internet Providers. Seun has a background in analytics, knowledge engineering which he makes use of to assist prospects obtain their outcomes and targets. Exterior of labor Seun enjoys spending time along with his household, studying, touring and supporting his favourite sports activities groups.
Vinod Jayendra is a Enterprise Assist Lead in ISV accounts at Amazon Internet Providers, the place he helps prospects in fixing their architectural, operational, and value optimization challenges. With a selected deal with Serverless applied sciences, he attracts from his intensive background in software growth to ship top-tier options. Past work, he finds pleasure in high quality household time, embarking on biking adventures, and training youth sports activities crew.
Kamen Sharlandjiev is a Sr. Massive Knowledge and ETL Options Architect, MWAA and AWS Glue ETL professional. He’s on a mission to make life simpler for purchasers who’re dealing with advanced knowledge integration and orchestration challenges. His secret weapon? Totally managed AWS providers that may get the job carried out with minimal effort. Comply with Kamen on LinkedIn to maintain updated with the most recent MWAA and AWS Glue options and information!
Chris Scull is a Options Architect dealing in orchestration instruments and trendy cloud applied sciences. With two years of expertise at AWS, Chris has developed an curiosity in Amazon Managed Workflows for Apache Airflow, which permits for environment friendly knowledge processing and workflow administration. Moreover, he’s enthusiastic about exploring the capabilities of GenAI with Bedrock, a platform for constructing generative AI purposes on AWS.
Shengjie Luo is a Massive knowledge architect of Amazon Cloud Know-how skilled service crew. Chargeable for options consulting, structure and supply of AWS based mostly knowledge warehouse and knowledge lake, and good at server-less computing, knowledge migration, cloud knowledge integration, knowledge warehouse planning, knowledge service structure design and implementation.
Qiushuang Feng is a Options Architect at AWS, answerable for Enterprise prospects’ technical structure design, consulting, and design optimization on AWS Cloud providers. Earlier than becoming a member of AWS, Qiushuang labored in IT corporations reminiscent of IBM and Oracle, and accrued wealthy sensible expertise in growth and analytics.