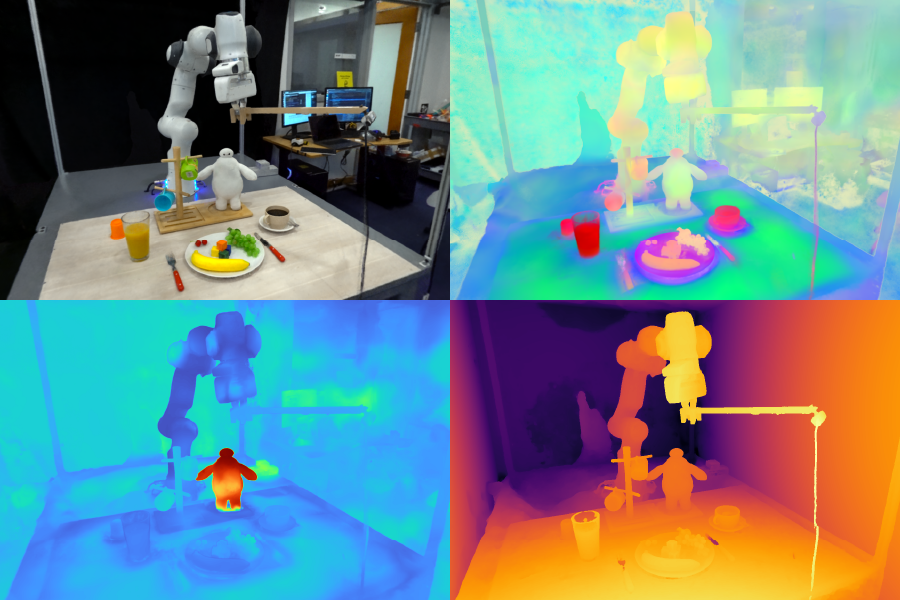
Function Fields for Robotic Manipulation (F3RM) permits robots to interpret open-ended textual content prompts utilizing pure language, serving to the machines manipulate unfamiliar objects. The system’s 3D characteristic fields may very well be useful in environments that comprise hundreds of objects, akin to warehouses. Photos courtesy of the researchers.
By Alex Shipps | MIT CSAIL
Think about you’re visiting a good friend overseas, and also you look inside their fridge to see what would make for a terrific breakfast. Most of the gadgets initially seem overseas to you, with each encased in unfamiliar packaging and containers. Regardless of these visible distinctions, you start to know what each is used for and choose them up as wanted.
Impressed by people’ potential to deal with unfamiliar objects, a bunch from MIT’s Laptop Science and Synthetic Intelligence Laboratory (CSAIL) designed Function Fields for Robotic Manipulation (F3RM), a system that blends 2D photos with basis mannequin options into 3D scenes to assist robots determine and grasp close by gadgets. F3RM can interpret open-ended language prompts from people, making the strategy useful in real-world environments that comprise hundreds of objects, like warehouses and households.
F3RM gives robots the power to interpret open-ended textual content prompts utilizing pure language, serving to the machines manipulate objects. Because of this, the machines can perceive less-specific requests from people and nonetheless full the specified activity. For instance, if a consumer asks the robotic to “choose up a tall mug,” the robotic can find and seize the merchandise that most closely fits that description.
“Making robots that may truly generalize in the true world is extremely laborious,” says Ge Yang, postdoc on the Nationwide Science Basis AI Institute for Synthetic Intelligence and Elementary Interactions and MIT CSAIL. “We actually need to determine how to do this, so with this venture, we attempt to push for an aggressive degree of generalization, from simply three or 4 objects to something we discover in MIT’s Stata Middle. We wished to learn to make robots as versatile as ourselves, since we are able to grasp and place objects despite the fact that we’ve by no means seen them earlier than.”
Studying “what’s the place by wanting”
The strategy may help robots with selecting gadgets in giant success facilities with inevitable muddle and unpredictability. In these warehouses, robots are sometimes given an outline of the stock that they’re required to determine. The robots should match the textual content supplied to an object, no matter variations in packaging, in order that clients’ orders are shipped appropriately.
For instance, the success facilities of main on-line retailers can comprise hundreds of thousands of things, lots of which a robotic may have by no means encountered earlier than. To function at such a scale, robots want to know the geometry and semantics of various gadgets, with some being in tight areas. With F3RM’s superior spatial and semantic notion skills, a robotic may change into simpler at finding an object, putting it in a bin, after which sending it alongside for packaging. In the end, this may assist manufacturing facility staff ship clients’ orders extra effectively.
“One factor that always surprises folks with F3RM is that the identical system additionally works on a room and constructing scale, and can be utilized to construct simulation environments for robotic studying and huge maps,” says Yang. “However earlier than we scale up this work additional, we need to first make this method work actually quick. This fashion, we are able to use one of these illustration for extra dynamic robotic management duties, hopefully in real-time, in order that robots that deal with extra dynamic duties can use it for notion.”
The MIT crew notes that F3RM’s potential to know completely different scenes may make it helpful in city and family environments. For instance, the method may assist personalised robots determine and choose up particular gadgets. The system aids robots in greedy their environment — each bodily and perceptively.
“Visible notion was outlined by David Marr as the issue of realizing ‘what’s the place by wanting,’” says senior creator Phillip Isola, MIT affiliate professor {of electrical} engineering and laptop science and CSAIL principal investigator. “Latest basis fashions have gotten actually good at realizing what they’re taking a look at; they’ll acknowledge hundreds of object classes and supply detailed textual content descriptions of photos. On the identical time, radiance fields have gotten actually good at representing the place stuff is in a scene. The mixture of those two approaches can create a illustration of what’s the place in 3D, and what our work exhibits is that this mixture is very helpful for robotic duties, which require manipulating objects in 3D.”
Making a “digital twin”
F3RM begins to know its environment by taking photos on a selfie stick. The mounted digicam snaps 50 photos at completely different poses, enabling it to construct a neural radiance discipline (NeRF), a deep studying technique that takes 2D photos to assemble a 3D scene. This collage of RGB pictures creates a “digital twin” of its environment within the type of a 360-degree illustration of what’s close by.
Along with a extremely detailed neural radiance discipline, F3RM additionally builds a characteristic discipline to reinforce geometry with semantic info. The system makes use of CLIP, a imaginative and prescient basis mannequin skilled on tons of of hundreds of thousands of photos to effectively be taught visible ideas. By reconstructing the 2D CLIP options for the pictures taken by the selfie stick, F3RM successfully lifts the 2D options right into a 3D illustration.
Retaining issues open-ended
After receiving just a few demonstrations, the robotic applies what it is aware of about geometry and semantics to know objects it has by no means encountered earlier than. As soon as a consumer submits a textual content question, the robotic searches by the area of potential grasps to determine these most definitely to achieve selecting up the thing requested by the consumer. Every potential choice is scored primarily based on its relevance to the immediate, similarity to the demonstrations the robotic has been skilled on, and if it causes any collisions. The best-scored grasp is then chosen and executed.
To exhibit the system’s potential to interpret open-ended requests from people, the researchers prompted the robotic to select up Baymax, a personality from Disney’s “Huge Hero 6.” Whereas F3RM had by no means been immediately skilled to select up a toy of the cartoon superhero, the robotic used its spatial consciousness and vision-language options from the muse fashions to determine which object to know and the way to choose it up.
F3RM additionally permits customers to specify which object they need the robotic to deal with at completely different ranges of linguistic element. For instance, if there’s a steel mug and a glass mug, the consumer can ask the robotic for the “glass mug.” If the bot sees two glass mugs and one among them is full of espresso and the opposite with juice, the consumer can ask for the “glass mug with espresso.” The inspiration mannequin options embedded inside the characteristic discipline allow this degree of open-ended understanding.
“If I confirmed an individual the way to choose up a mug by the lip, they might simply switch that data to select up objects with related geometries akin to bowls, measuring beakers, and even rolls of tape. For robots, reaching this degree of adaptability has been fairly difficult,” says MIT PhD scholar, CSAIL affiliate, and co-lead creator William Shen. “F3RM combines geometric understanding with semantics from basis fashions skilled on internet-scale information to allow this degree of aggressive generalization from only a small variety of demonstrations.”
Shen and Yang wrote the paper underneath the supervision of Isola, with MIT professor and CSAIL principal investigator Leslie Pack Kaelbling and undergraduate college students Alan Yu and Jansen Wong as co-authors. The crew was supported, partly, by Amazon.com Providers, the Nationwide Science Basis, the Air Power Workplace of Scientific Analysis, the Workplace of Naval Analysis’s Multidisciplinary College Initiative, the Military Analysis Workplace, the MIT-IBM Watson Lab, and the MIT Quest for Intelligence. Their work shall be introduced on the 2023 Convention on Robotic Studying.
MIT Information