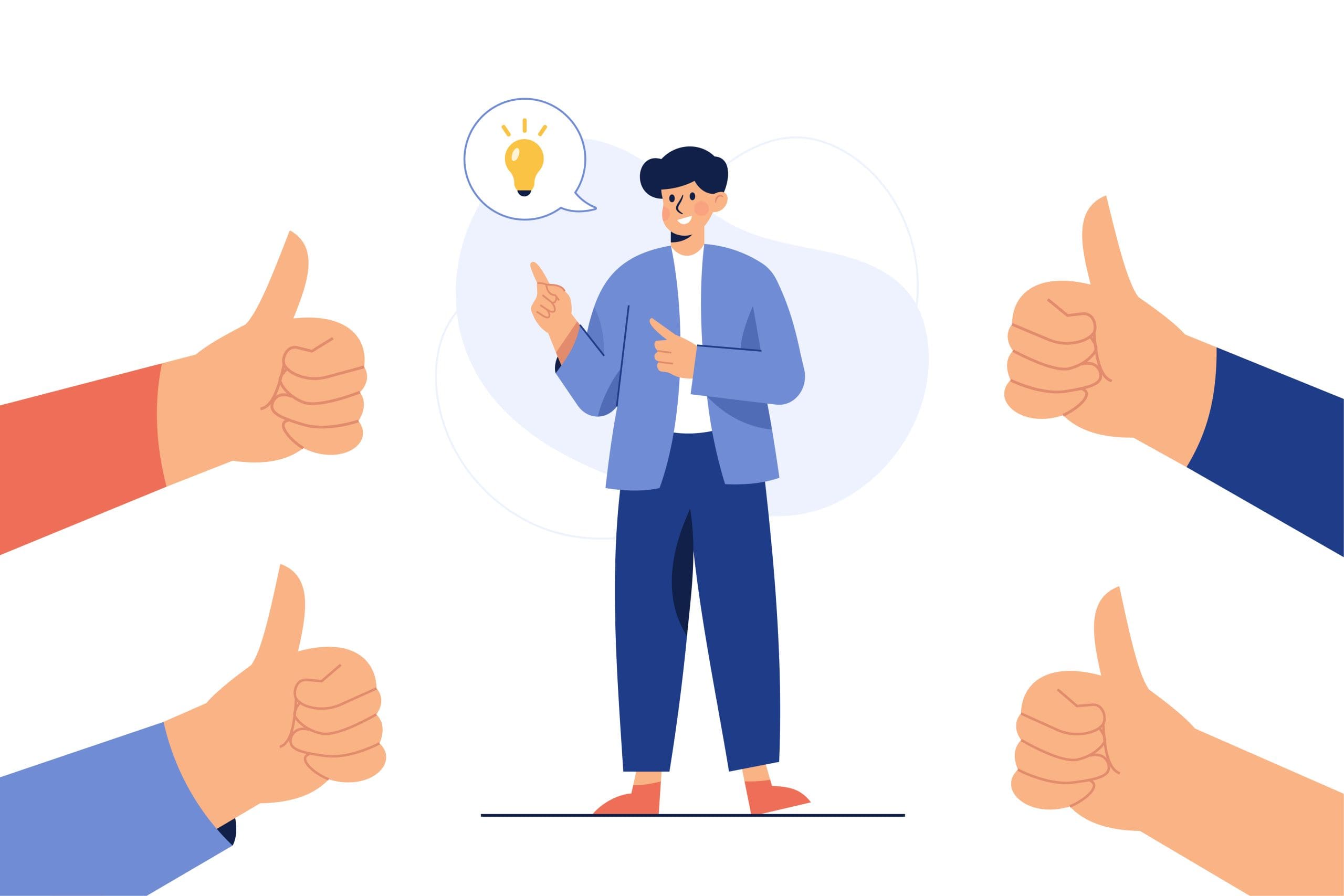
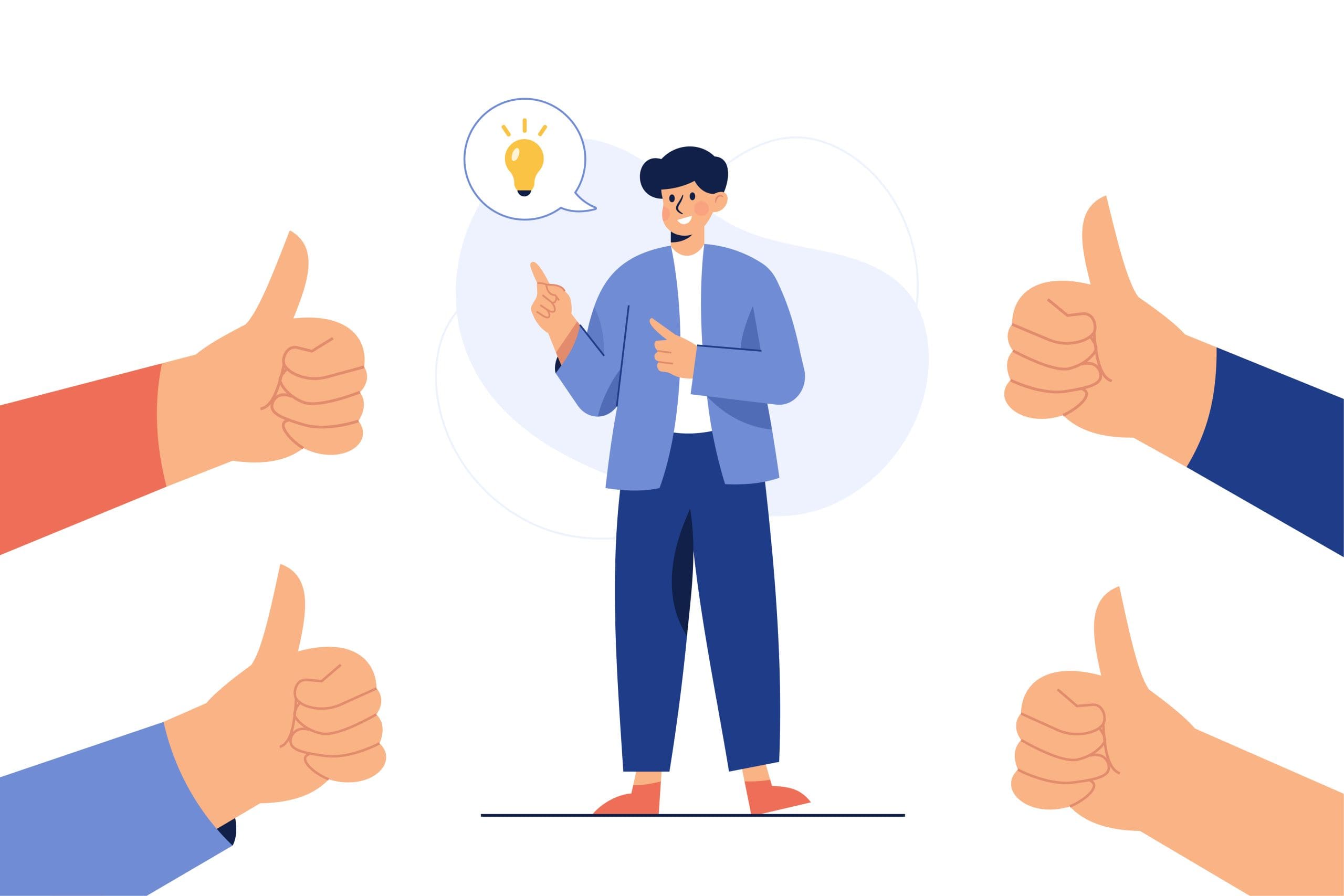
Picture by jcomp on Freepik
We have now relied on software program in our telephones and computer systems within the fashionable period. Many purposes, similar to e-commerce, film streaming, sport platforms, and others, have modified how we dwell, as these purposes make issues simpler. To make issues even higher, the enterprise usually offers options that permit suggestions from the info.
The idea of advice techniques is to foretell what the consumer may focused on primarily based on the enter. The system would supply the closest gadgets primarily based on both the similarity between the gadgets (content-based filtering) or the conduct (collaborative filtering).
With many approaches to the advice system structure, we will use the Hugging Face Transformers bundle. When you didn’t know, Hugging Face Transformers is an open-source Python bundle that permits APIs to simply entry all of the pre-trained NLP fashions that help duties similar to textual content processing, era, and lots of others.
This text will use the Hugging Face Transformers bundle to develop a easy suggestion system primarily based on embedding similarity. Let’s get began.
Develop a Advice System with Hugging Face Transformers
Earlier than we begin the tutorial, we have to set up the required packages. To do this, you should utilize the next code:
pip set up transformers torch pandas scikit-learn
You may choose the appropriate model on your atmosphere by way of their web site for the Torch set up.
As for the dataset instance, we’d use the Anime suggestion dataset instance from Kaggle.
As soon as the atmosphere and the dataset are prepared, we’ll begin the tutorial. First, we have to learn the dataset and put together them.
import pandas as pd
df = pd.read_csv('anime.csv')
df = df.dropna()
df['description'] = df['name'] +' '+ df['genre'] + ' ' +df['type']+' episodes: '+ df['episodes']
Within the code above, we learn the dataset with Pandas and dropped all of the lacking information. Then, we create a function referred to as “description” that incorporates all the data from the out there information, similar to identify, style, sort, and episode quantity. The brand new column would develop into our foundation for the advice system. It might be higher to have extra full data, such because the anime plot and abstract, however let’s be content material with this one for now.
Subsequent, we’d use Hugging Face Transformers to load an embedding mannequin and remodel the textual content right into a numerical vector. Particularly, we’d use sentence embedding to remodel the entire sentence.
The advice system can be primarily based on the embedding from all of the anime “description” we’ll carry out quickly. We might use the cosine similarity technique, which measures the similarity of two vectors. By measuring the similarity between the anime “description” embedding and the consumer’s question enter embedding, we will get exact gadgets to suggest.
The embedding similarity strategy sounds easy, however it may be highly effective in comparison with the traditional suggestion system mannequin, as it may seize the semantic relationship between phrases and supply contextual which means for the advice course of.
We might use the embedding mannequin sentence transformers from the Hugging Face for this tutorial. To remodel the sentence into embedding, we’d use the next code.
from transformers import AutoTokenizer, AutoModel
import torch
import torch.nn.practical as F
def mean_pooling(model_output, attention_mask):
token_embeddings = model_output[0] #First factor of model_output incorporates all token embeddings
input_mask_expanded = attention_mask.unsqueeze(-1).increase(token_embeddings.measurement()).float()
return torch.sum(token_embeddings * input_mask_expanded, 1) / torch.clamp(input_mask_expanded.sum(1), min=1e-9)
tokenizer = AutoTokenizer.from_pretrained('sentence-transformers/all-MiniLM-L6-v2')
mannequin = AutoModel.from_pretrained('sentence-transformers/all-MiniLM-L6-v2')
def get_embeddings(sentences):
encoded_input = tokenizer(sentences, padding=True, truncation=True, return_tensors="pt")
with torch.no_grad():
model_output = mannequin(**encoded_input)
sentence_embeddings = mean_pooling(model_output, encoded_input['attention_mask'])
sentence_embeddings = F.normalize(sentence_embeddings, p=2, dim=1)
return sentence_embeddings
Strive the embedding course of and see the vector consequence with the next code. Nevertheless, I might not present the output because it’s fairly lengthy.
sentences = ['Some great movie', 'Another funny movie']
consequence = get_embeddings(sentences)
print("Sentence embeddings:")
print(consequence)
To make issues simpler, Hugging Face maintains a Python bundle for embedding sentence transformers, which might decrease the entire transformation course of in 3 strains of code. Set up the mandatory bundle utilizing the code beneath.
pip set up -U sentence-transformers
Then, we will remodel the entire anime “description” with the next code.
from sentence_transformers import SentenceTransformer
mannequin = SentenceTransformer('sentence-transformers/all-MiniLM-L6-v2')
anime_embeddings = mannequin.encode(df['description'].tolist())
With the embedding database is prepared, we’d create a operate to take consumer enter and carry out cosine similarity as a suggestion system.
from sklearn.metrics.pairwise import cosine_similarity
def get_recommendations(question, embeddings, df, top_n=5):
query_embedding = mannequin.encode([query])
similarities = cosine_similarity(query_embedding, embeddings)
top_indices = similarities[0].argsort()[-top_n:][::-1]
return df.iloc[top_indices]
Now that every little thing is prepared, we will strive the advice system. Right here is an instance of buying the highest 5 anime suggestions from the consumer enter question.
question = "Humorous anime I can watch with associates"
suggestions = get_recommendations(question, anime_embeddings, df)
print(suggestions[['name', 'genre']])
Output>>
identify
7363 Sentou Yousei Shoujo Tasukete! Mave-chan
8140 Anime TV de Hakken! Tamagotchi
4294 SKET Dance: SD Character Flash Anime
1061 Isshuukan Mates.
2850 Oshiete! Galko-chan
style
7363 Comedy, Parody, Sci-Fi, Shounen, Tremendous Energy
8140 Comedy, Fantasy, Children, Slice of Life
4294 Comedy, Faculty, Shounen
1061 Comedy, Faculty, Shounen, Slice of Life
2850 Comedy, Faculty, Slice of Life
The result’s all the comedy anime, as we would like the humorous anime. Most of them additionally embrace anime, which is appropriate to observe with associates from the style. In fact, the advice can be even higher if we had extra detailed data.
Conclusion
A Advice System is a device for predicting what customers is likely to be focused on primarily based on the enter. Utilizing Hugging Face Transformers, we will construct a suggestion system that makes use of the embedding and cosine similarity strategy. The embedding strategy is highly effective as it may account for the textual content’s semantic relationship and contextual which means.
Cornellius Yudha Wijaya is an information science assistant supervisor and information author. Whereas working full-time at Allianz Indonesia, he likes to share Python and information suggestions by way of social media and writing media. Cornellius writes on quite a lot of AI and machine studying subjects.