On this submit, we discover the efficiency advantages of utilizing the Amazon EMR runtime for Apache Spark and Apache Iceberg in comparison with operating the identical workloads with open supply Spark 3.5.1 on Iceberg tables. Iceberg is a well-liked open supply high-performance format for big analytic tables. Our benchmarks show that Amazon EMR can run TPC-DS 3 TB workloads 2.7 instances sooner, lowering the runtime from 1.548 hours to 0.564 hours. Moreover, the fee effectivity improves by 2.2 instances, with the full value reducing from $16.09 to $7.23 when utilizing Amazon Elastic Compute Cloud (Amazon EC2) On-Demand r5d.4xlarge cases, offering observable good points for information processing duties.
The Amazon EMR runtime for Apache Spark provides a high-performance runtime atmosphere whereas sustaining 100% API compatibility with open supply Spark and Iceberg desk format. In Run Apache Spark 3.5.1 workloads 4.5 instances sooner with Amazon EMR runtime for Apache Spark, we detailed among the optimizations, exhibiting a runtime enchancment of 4.5 instances sooner and a couple of.8 instances higher price-performance in comparison with open supply Spark 3.5.1 on the TPC-DS 3 TB benchmark. Nonetheless, most of the optimizations are geared in the direction of DataSource V1, whereas Iceberg makes use of Spark DataSource V2. Recognizing this, we’ve centered on migrating among the present optimizations within the EMR runtime for Spark to DataSource V2 and introducing Iceberg-specific enhancements. These enhancements are constructed on prime of the Spark runtime enhancements on question planning, bodily plan operator enhancements, and optimizations with Amazon Easy Storage Service (Amazon S3) and the Java runtime. We’ve added eight new optimizations incrementally for the reason that Amazon EMR 6.15 launch in 2023, that are current in Amazon EMR 7.1 and turned on by default. A few of the enhancements embody the next:
- Optimizing DataSource V2 in Spark:
- Dynamic filtering on non-partitioned columns
- Eradicating redundant broadcast hash joins
- Partial hash combination pushdowns
- Bloom filter-based joins
- Iceberg-specific enhancements:
- Knowledge prefetch
- Help for file size-based estimations
Amazon EMR on EC2, Amazon EMR Serverless, Amazon EMR on Amazon EKS, and Amazon EMR on AWS Outposts all use the optimized runtimes. Consult with Working with Apache Iceberg in Amazon EMR and Finest practices for optimizing Apache Iceberg workloads for extra particulars.
Benchmark outcomes for Amazon EMR 7.1 vs. open supply Spark 3.5.1 and Iceberg 1.5.2
To evaluate the Spark engine’s efficiency with the Iceberg desk format, we carried out benchmark exams utilizing the 3 TB TPC-DS dataset, model 2.13 (our outcomes derived from the TPC-DS dataset will not be immediately similar to the official TPC-DS outcomes on account of setup variations). Benchmark exams for the EMR runtime for Spark and Iceberg have been performed on Amazon EMR 7.1 clusters with Spark 3.5.0 and Iceberg 1.4.3-amzn-0 variations, and open supply Spark 3.5.1 and Iceberg 1.5.2 was deployed on EC2 clusters designated for open supply runs.
The setup directions and technical particulars can be found in our GitHub repository. To reduce the affect of exterior catalogs like AWS Glue and Hive, we used the Hadoop catalog for the Iceberg tables. This makes use of the underlying file system, particularly Amazon S3, because the catalog. We will outline this setup by configuring the property spark.sql.catalog..kind
. The actual fact tables used the default partitioning by the date column, which have plenty of partitions various from 200–2,100. No precalculated statistics have been used for these tables.
We ran a complete of 104 SparkSQL queries in three sequential rounds, and the typical runtime of every question throughout these rounds was taken for comparability. The typical runtime for the three rounds on Amazon EMR 7.1 with Iceberg enabled was 0.56 hours, demonstrating a 2.7-fold velocity improve in comparison with open supply Spark 3.5.1 and Iceberg 1.5.2. The next determine presents the full runtimes in seconds.
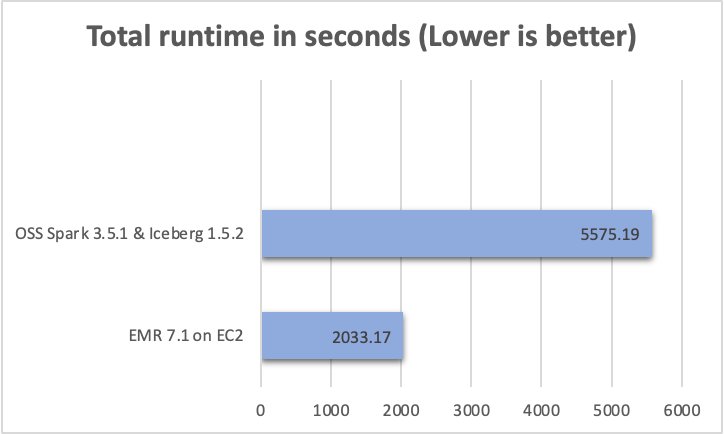
The next desk summarizes the metrics.
Metric |
Amazon EMR 7.1 on EC2 |
Open Supply Spark 3.5.1 and Iceberg 1.5.2 |
Common runtime in seconds |
2033.17 |
5575.19 |
Geometric imply over queries in seconds |
10.13153 |
20.34651 |
Value* |
$7.23 |
$16.09 |
*Detailed value estimates are mentioned later on this submit.
The next chart demonstrates the per-query efficiency enchancment of Amazon EMR 7.1 relative to open supply Spark 3.5.1 and Iceberg 1.5.2. The extent of the speedup varies from one question to a different, starting from 9.6 instances sooner for q93 to 1.04 instances sooner for q34, with Amazon EMR outperforming the open supply Spark with Iceberg tables. The horizontal axis arranges the TPC-DS 3 TB benchmark queries in descending order primarily based on the efficiency enchancment seen with Amazon EMR, and the vertical axis depicts the magnitude of this speedup in seconds.
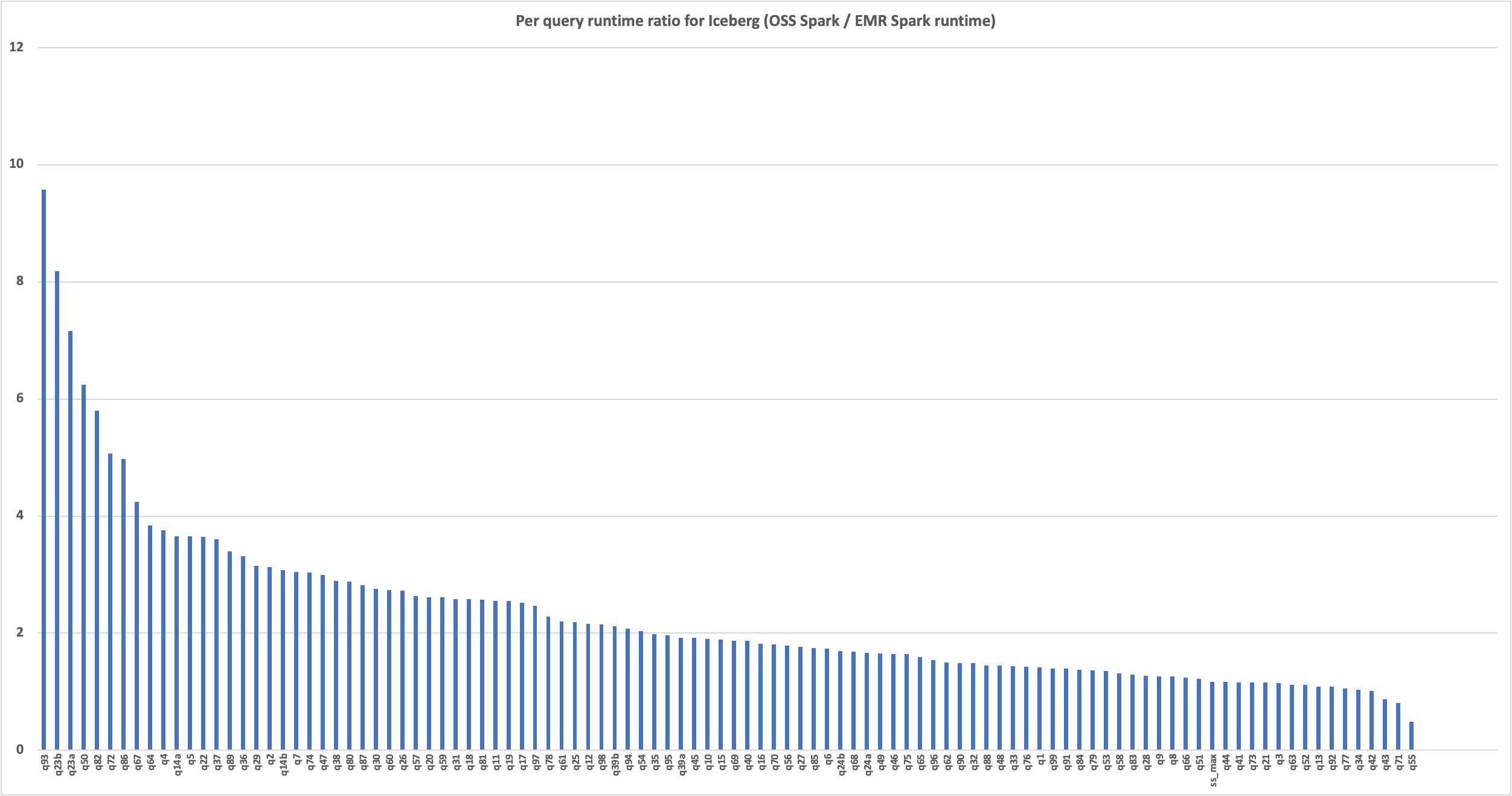
Value comparability
Our benchmark gives the full runtime and geometric imply information to evaluate the efficiency of Spark and Iceberg in a fancy, real-world choice assist state of affairs. For extra insights, we additionally study the fee facet. We calculate value estimates utilizing formulation that account for EC2 On-Demand cases, Amazon Elastic Block Retailer (Amazon EBS), and Amazon EMR bills.
- Amazon EC2 value (consists of SSD value) = variety of cases * r5d.4xlarge hourly charge * job runtime in hours
- 4xlarge hourly charge = $1.152 per hour
- Root Amazon EBS value = variety of cases * Amazon EBS per GB-hourly charge * root EBS quantity measurement * job runtime in hours
- Amazon EMR value = variety of cases * r5d.4xlarge Amazon EMR value * job runtime in hours
- 4xlarge Amazon EMR value = $0.27 per hour
- Whole value = Amazon EC2 value + root Amazon EBS value + Amazon EMR value
The calculations reveal that the Amazon EMR 7.1 benchmark yields a 2.2-fold value effectivity enchancment over open supply Spark 3.5.1 and Iceberg 1.5.2 in operating the benchmark job.
Metric |
Amazon EMR 7.1 |
Open Supply Spark 3.5.1 and Iceberg 1.5.2 |
Runtime in hours |
0.564 |
1.548 |
Variety of EC2 cases |
9 |
9 |
Amazon EBS Measurement |
20gb |
20gb |
Amazon EC2 value |
$5.85 |
$16.05 |
Amazon EBS value |
$0.01 |
$0.04 |
Amazon EMR value |
$1.37 |
$0 |
Whole value |
$7.23 |
$16.09 |
Value financial savings |
Amazon EMR 7.1 is 2.2 instances higher |
Baseline |
Along with the time-based metrics mentioned to date, information from Spark occasion logs reveals that Amazon EMR 7.1 scanned roughly 3.4 instances much less information from Amazon S3 and 4.1 instances fewer data than the open supply model within the TPC-DS 3 TB benchmark. This discount in Amazon S3 information scanning contributes on to value financial savings for Amazon EMR workloads.
Run open supply Spark benchmarks on Iceberg tables
We used separate EC2 clusters, every outfitted with 9 r5d.4xlarge cases, for testing each open supply Spark 3.5.1 and Iceberg 1.5.2 and Amazon EMR 7.1. The first node was outfitted with 16 vCPU and 128 GB of reminiscence, and the eight employee nodes collectively had 128 vCPU and 1024 GB of reminiscence. We performed exams utilizing the Amazon EMR default settings to showcase the standard consumer expertise and minimally adjusted the settings of Spark and Iceberg to keep up a balanced comparability.
The next desk summarizes the Amazon EC2 configurations for the first node and eight employee nodes of kind r5d.4xlarge.
EC2 Occasion |
vCPU |
Reminiscence (GiB) |
Occasion Storage (GB) |
EBS Root Quantity (GB) |
r5d.4xlarge |
16 |
128 |
2 x 300 NVMe SSD |
20 GB |
Stipulations
The next stipulations are required to run the benchmarking:
- Utilizing the directions within the emr-spark-benchmark GitHub repo, arrange the TPC-DS supply information in your S3 bucket and in your native pc.
- Construct the benchmark software following the steps supplied in Steps to construct spark-benchmark-assembly software and replica the benchmark software to your S3 bucket. Alternatively, copy spark-benchmark-assembly-3.5.1.jar to your S3 bucket.
- Create Iceberg tables from the TPC-DS supply information. Observe the directions on GitHub to create Iceberg tables utilizing the Hadoop catalog. For instance, the next code makes use of an EMR 7.1 cluster with Iceberg enabled to create the tables:
aws emr add-steps --cluster-id --steps Sort=Spark,Identify="Create Iceberg Tables",
Args=[--class,com.amazonaws.eks.tpcds.CreateIcebergTables,
--conf,spark.sql.extensions=org.apache.iceberg.spark.extensions.IcebergSparkSessionExtensions,
--conf,spark.sql.catalog.hadoop_catalog=org.apache.iceberg.spark.SparkCatalog,
--conf,spark.sql.catalog.hadoop_catalog.type=hadoop,
--conf,spark.sql.catalog.hadoop_catalog.warehouse=s3:////,
--conf,spark.sql.catalog.hadoop_catalog.io-impl=org.apache.iceberg.aws.s3.S3FileIO,
s3:////spark-benchmark-assembly-3.5.1.jar,
s3://blogpost-sparkoneks-us-east-1/blog/BLOG_TPCDS-TEST-3T-partitioned/,
/home/hadoop/tpcds-kit/tools,parquet,3000,true,,true,true],ActionOnFailure=CONTINUE
--region
Be aware the Hadoop catalog warehouse location and database identify from the previous step. We use the identical tables to run benchmarks with Amazon EMR 7.1 and open supply Spark and Iceberg.
This benchmark software is constructed from the department tpcds-v2.13_iceberg. For those who’re constructing a brand new benchmark software, swap to the proper department after downloading the supply code from the GitHub repo.
Create and configure a YARN cluster on Amazon EC2
To match Iceberg efficiency between Amazon EMR on Amazon EC2 and open supply Spark on Amazon EC2, observe the directions within the emr-spark-benchmark GitHub repo to create an open supply Spark cluster on Amazon EC2 utilizing Flintrock with eight employee nodes.
Primarily based on the cluster choice for this check, the next configurations are used:
Run the TPC-DS benchmark with Apache Spark 3.5.1 and Iceberg 1.5.2
Full the next steps to run the TPC-DS benchmark:
- Log in to the open supply cluster main utilizing
flintrock login $CLUSTER_NAME
.
- Submit your Spark job:
- Select the proper Iceberg catalog warehouse location and database that has the created Iceberg tables.
- The outcomes are created in
s3:///benchmark_run
.
- You possibly can monitor progress in
/media/ephemeral0/spark_run.log
.
spark-submit
--master yarn
--deploy-mode shopper
--class com.amazonaws.eks.tpcds.BenchmarkSQL
--conf spark.driver.cores=4
--conf spark.driver.reminiscence=10g
--conf spark.executor.cores=16
--conf spark.executor.reminiscence=100g
--conf spark.executor.cases=8
--conf spark.community.timeout=2000
--conf spark.executor.heartbeatInterval=300s
--conf spark.dynamicAllocation.enabled=false
--conf spark.shuffle.service.enabled=false
--conf spark.hadoop.fs.s3a.aws.credentials.supplier=com.amazonaws.auth.InstanceProfileCredentialsProvider
--conf spark.hadoop.fs.s3.impl=org.apache.hadoop.fs.s3a.S3AFileSystem
--conf spark.jars.packages=org.apache.hadoop:hadoop-aws:3.3.4,org.apache.iceberg:iceberg-spark-runtime-3.5_2.12:1.5.2,org.apache.iceberg:iceberg-aws-bundle:1.5.2
--conf spark.sql.extensions=org.apache.iceberg.spark.extensions.IcebergSparkSessionExtensions
--conf spark.sql.catalog.native=org.apache.iceberg.spark.SparkCatalog
--conf spark.sql.catalog.native.kind=hadoop
--conf spark.sql.catalog.native.warehouse=s3a:////
--conf spark.sql.defaultCatalog=native
--conf spark.sql.catalog.native.io-impl=org.apache.iceberg.aws.s3.S3FileIO
spark-benchmark-assembly-3.5.1.jar
s3:///benchmark_run 3000 1 false
q1-v2.13,q10-v2.13,q11-v2.13,q12-v2.13,q13-v2.13,q14a-v2.13,q14b-v2.13,q15-v2.13,q16-v2.13,
q17-v2.13,q18-v2.13,q19-v2.13,q2-v2.13,q20-v2.13,q21-v2.13,q22-v2.13,q23a-v2.13,q23b-v2.13,
q24a-v2.13,q24b-v2.13,q25-v2.13,q26-v2.13,q27-v2.13,q28-v2.13,q29-v2.13,q3-v2.13,q30-v2.13,
q31-v2.13,q32-v2.13,q33-v2.13,q34-v2.13,q35-v2.13,q36-v2.13,q37-v2.13,q38-v2.13,q39a-v2.13,
q39b-v2.13,q4-v2.13,q40-v2.13,q41-v2.13,q42-v2.13,q43-v2.13,q44-v2.13,q45-v2.13,q46-v2.13,
q47-v2.13,q48-v2.13,q49-v2.13,q5-v2.13,q50-v2.13,q51-v2.13,q52-v2.13,q53-v2.13,q54-v2.13,
q55-v2.13,q56-v2.13,q57-v2.13,q58-v2.13,q59-v2.13,q6-v2.13,q60-v2.13,q61-v2.13,q62-v2.13,
q63-v2.13,q64-v2.13,q65-v2.13,q66-v2.13,q67-v2.13,q68-v2.13,q69-v2.13,q7-v2.13,q70-v2.13,
q71-v2.13,q72-v2.13,q73-v2.13,q74-v2.13,q75-v2.13,q76-v2.13,q77-v2.13,q78-v2.13,q79-v2.13,
q8-v2.13,q80-v2.13,q81-v2.13,q82-v2.13,q83-v2.13,q84-v2.13,q85-v2.13,q86-v2.13,q87-v2.13,
q88-v2.13,q89-v2.13,q9-v2.13,q90-v2.13,q91-v2.13,q92-v2.13,q93-v2.13,q94-v2.13,q95-v2.13,
q96-v2.13,q97-v2.13,q98-v2.13,q99-v2.13,ss_max-v2.13
true > /media/ephemeral0/spark_run.log 2>&1 &!
Summarize the outcomes
After the Spark job finishes, retrieve the check end result file from the output S3 bucket at s3:///benchmark_run/timestamp=xxxx/abstract.csv/xxx.csv
. This may be performed both by means of the Amazon S3 console by navigating to the required bucket location or through the use of the Amazon Command Line Interface (AWS CLI). The Spark benchmark software organizes the information by making a timestamp folder and putting a abstract file inside a folder labeled abstract.csv
. The output CSV recordsdata include 4 columns with out headers:
- Question identify
- Median time
- Minimal time
- Most time
With the information from three separate check runs with one iteration every time, we will calculate the typical and geometric imply of the benchmark runtimes.
Run the TPC-DS benchmark with the EMR runtime for Spark
Many of the directions are much like Steps to run Spark Benchmarking with just a few Iceberg-specific particulars.
Stipulations
Full the next prerequisite steps:
- Run
aws configure
to configure the AWS CLI shell to level to the benchmarking AWS account. Consult with Configure the AWS CLI for directions.
- Add the benchmark software JAR file to Amazon S3.
Deploy the EMR cluster and run the benchmark job
Full the next steps to run the benchmark job:
- Use the AWS CLI command as proven in Deploy EMR on EC2 Cluster and run benchmark job to spin up an EMR on EC2 cluster. Make certain to allow Iceberg. See Create an Iceberg cluster for extra particulars. Select the proper Amazon EMR model, root quantity measurement, and similar useful resource configuration because the open supply Flintrock setup. Consult with create-cluster for an in depth description of the AWS CLI choices.
- Retailer the cluster ID from the response. We’d like this for the following step.
- Submit the benchmark job in Amazon EMR utilizing
add-steps
from the AWS CLI:
- Exchange with the cluster ID from Step 2.
- The benchmark software is at
s3:///spark-benchmark-assembly-3.5.1.jar
.
- Select the proper Iceberg catalog warehouse location and database that has the created Iceberg tables. This needs to be the identical because the one used for the open supply TPC-DS benchmark run.
- The outcomes will probably be in
s3:///benchmark_run
.
aws emr add-steps --cluster-id
--steps Sort=Spark,Identify="SPARK Iceberg EMR TPCDS Benchmark Job",
Args=[--class,com.amazonaws.eks.tpcds.BenchmarkSQL,
--conf,spark.driver.cores=4,
--conf,spark.driver.memory=10g,
--conf,spark.executor.cores=16,
--conf,spark.executor.memory=100g,
--conf,spark.executor.instances=8,
--conf,spark.network.timeout=2000,
--conf,spark.executor.heartbeatInterval=300s,
--conf,spark.dynamicAllocation.enabled=false,
--conf,spark.shuffle.service.enabled=false,
--conf,spark.sql.iceberg.data-prefetch.enabled=true,
--conf,spark.sql.extensions=org.apache.iceberg.spark.extensions.IcebergSparkSessionExtensions,
--conf,spark.sql.catalog.local=org.apache.iceberg.spark.SparkCatalog,
--conf,spark.sql.catalog.local.type=hadoop,
--conf,spark.sql.catalog.local.warehouse=s3:///,
--conf,spark.sql.defaultCatalog=local,
--conf,spark.sql.catalog.local.io-impl=org.apache.iceberg.aws.s3.S3FileIO,
s3:///spark-benchmark-assembly-3.5.1.jar,
s3:///benchmark_run,3000,1,false,
'q1-v2.13,q10-v2.13,q11-v2.13,q12-v2.13,q13-v2.13,q14a-v2.13,
q14b-v2.13,q15-v2.13,q16-v2.13,q17-v2.13,q18-v2.13,q19-v2.13,
q2-v2.13,q20-v2.13,q21-v2.13,q22-v2.13,q23a-v2.13,q23b-v2.13,
q24a-v2.13,q24b-v2.13,q25-v2.13,q26-v2.13,q27-v2.13,q28-v2.13,
q29-v2.13,q3-v2.13,q30-v2.13,q31-v2.13,q32-v2.13,q33-v2.13,
q34-v2.13,q35-v2.13,q36-v2.13,q37-v2.13,q38-v2.13,q39a-v2.13,
q39b-v2.13,q4-v2.13,q40-v2.13,q41-v2.13,q42-v2.13,q43-v2.13,
q44-v2.13,q45-v2.13,q46-v2.13,q47-v2.13,q48-v2.13,q49-v2.13,
q5-v2.13,q50-v2.13,q51-v2.13,q52-v2.13,q53-v2.13,q54-v2.13,
q55-v2.13,q56-v2.13,q57-v2.13,q58-v2.13,q59-v2.13,q6-v2.13,
q60-v2.13,q61-v2.13,q62-v2.13,q63-v2.13,q64-v2.13,q65-v2.13,
q66-v2.13,q67-v2.13,q68-v2.13,q69-v2.13,q7-v2.13,q70-v2.13,
q71-v2.13,q72-v2.13,q73-v2.13,q74-v2.13,q75-v2.13,q76-v2.13,
q77-v2.13,q78-v2.13,q79-v2.13,q8-v2.13,q80-v2.13,q81-v2.13,
q82-v2.13,q83-v2.13,q84-v2.13,q85-v2.13,q86-v2.13,q87-v2.13,
q88-v2.13,q89-v2.13,q9-v2.13,q90-v2.13,q91-v2.13,q92-v2.13,
q93-v2.13,q94-v2.13,q95-v2.13,q96-v2.13,q97-v2.13,q98-v2.13,
q99-v2.13,ss_max-v2.13',true,],ActionOnFailure=CONTINUE
--region
Summarize the outcomes
After the step is full, you possibly can see the summarized benchmark end result at s3:///benchmark_run/timestamp=xxxx/abstract.csv/xxx.csv
in the identical approach because the earlier run and compute the typical and geometric imply of the question runtimes.
Clear up
To stop any future expenses, delete the sources you created by following the directions supplied within the Cleanup part of the GitHub repository.
Abstract
Amazon EMR is persistently enhancing the EMR runtime for Spark when used with Iceberg tables, attaining a efficiency that’s 2.7 instances sooner than open supply Spark 3.5.1 and Iceberg 1.5.2 on TPC-DS 3 TB, v2.13. We encourage you to maintain updated with the most recent Amazon EMR releases to completely profit from ongoing efficiency enhancements.
To remain knowledgeable, subscribe to the AWS Large Knowledge Weblog’s RSS feed, the place you’ll find updates on the EMR runtime for Spark and Iceberg, in addition to tips about configuration greatest practices and tuning suggestions.
Concerning the authors
Hari Kishore Chaparala is a software program growth engineer for Amazon EMR at Amazon Net Providers.
Udit Mehrotra is an Engineering Supervisor for EMR at Amazon Net Providers.