The medical expertise trade is a widely known a part of the general healthcare sector. Based on Statista, roughly 1.3 billion folks used digital well being in 2024. This included utilizing health trackers and smartwatches, distant physician consultations and classes, or some other digital remedy and care use.
An enormous enlargement within the growth of medical applied sciences has come from the coalition with synthetic intelligence.
It’s extensively accepted in healthcare that using AI brings time financial savings for healthcare workers, relieves burnout, lowers stress ranges and dealing additional time, and frees time spent on direct affected person care.
However one of the vital promising methods AI growth is being utilized in healthcare is in medical coding — a course of that hyperlinks medical documentation with billing, insurance coverage, and compliance programs.
What Is AI-Powered Medical Coding Software program?
AI-based medical coding software program is a program that helps medical caregivers routinely convert medical information (resembling physician’s notes or medical historical past extracts) into appropriate codes used for billing, insurance coverage claims, and monitoring affected person care.
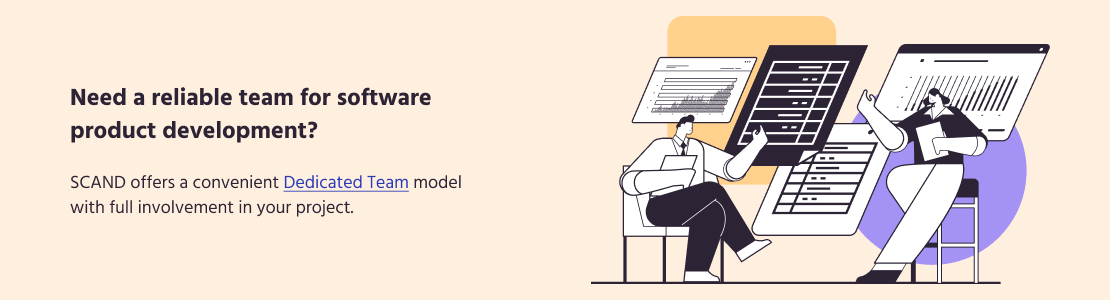
What are these codes:
- ICD-10 (Worldwide Classification of Illnesses): codes for diagnoses. For instance, J45 is bronchial asthma.
- CPT (Present Procedural Terminology): codes for procedures and providers. For instance, 99213 is a mid-term physician’s appointment.
- HCPCS (Healthcare Frequent Process Coding System): an extension of CPT, overlaying, for instance, medical merchandise and gear (e.g., wheelchairs).
- SNOMED CT is a medical terminology used to explain medical info in an expanded method.
As a result of codes are standardized and their use is obligatory in healthcare, beforehand, medical coders needed to learn physician’s reviews and enter codes manually.
Now, AI applies pure language processing (NLP) and machine studying algorithms (ML) and will get the job carried out manner quicker and with extra accuracy.
| Conventional Coding |
AI-Powered Coding |
The way it’s carried out |
By folks studying and coding manually |
By software program that understands medical textual content |
Pace |
Slower, particularly with a number of information |
A lot quicker, typically in actual time |
Accuracy |
Depends upon the coder’s expertise |
Constant and will get higher over time |
Scalability |
Restricted by workers |
Simply handles massive volumes of information |
Conventional vs. AI-Primarily based Coding
Easy Instance
Let’s say a doctor writes in an digital medical report: “The affected person got here with complaints of shortness of breath and wheezing. An inhaler was prescribed. Analysis: bronchial asthma.”
What the AI system does:
- Extracts medical information — reads textual content from the EHR (digital medical report).
- Analyzes utilizing NLP — acknowledges that the analysis “bronchial asthma”, the symptom “shortness of breath”, and the process “inhaler prescription” are talked about.
- Applies guidelines and deep studying — the system determines which codes precisely correspond to the desired info. For instance:
- Bronchial asthma analysis → ICD-10 code J45.909
- The common size of doctor go to → CPT code 99213
- Inhaler prescription → could require HCPCS code for a selected system, resembling HCPCS A4614 (if a nebulizer or consumable is indicated)
- Checks for errors and compliance with guidelines — AI can detect if one thing doesn’t match (for instance, a drug is prescribed, however the analysis that justifies it’s not indicated).
- Generates coding and sends the coding information to the medical billing system, from the place it goes to the insurance coverage firm for reimbursement.
Advantages of AI Medical Coding Software program
Do you know that as much as 30% of claims created with guide coding are denied, and as much as 65% are by no means reworked, negatively affecting income cycles?
Total, medical coding software program with the help of AI reduces denials and ensures healthcare suppliers work quicker, extra precisely, and fewer harassed.
Once more, Statista reveals that round 4 out of ten medical doctors and 1 / 4 of nurses admit that AI brings optimistic and welcome progress to their work within the healthcare system.
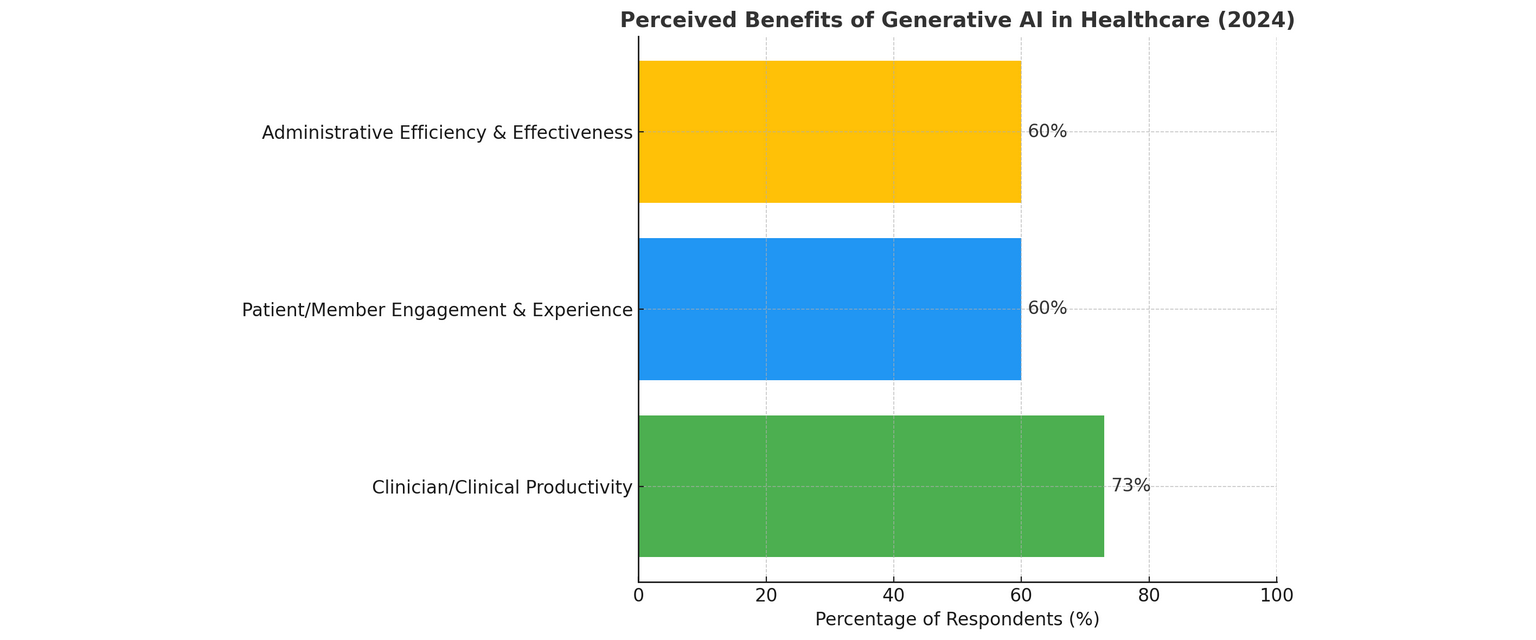
One of many biggest benefits is that it eliminates (or no less than reduces to zero) human errors and raises coding accuracy. This system can comprehend and perceive medical notes, after which present computerized ideas for the proper billing codes.
In consequence, there are rarer errors, fewer rejected claims, and fewer time to resolve instances.
As well as, AI medical coding software program is time-wise. Poor productiveness, which is anticipated to worsen as a result of an growing old inhabitants and a scarcity of important employees, is mirrored in excessive charges of doctor burnout.
Nevertheless, what might take hours for one particular person to code can now be carried out in minutes utilizing AI. This implies faster billing and serving to healthcare professionals receives a commission earlier.
An extra significant profit is the decrease expense. Since a variety of the drudge work is completed by software program, healthcare organizations don’t want as many individuals coding manually. Apart from, fewer errors imply fewer delays and decrease administrative funds.
Additionally, AI software program can simply address complicated instances if skilled correctly. It might probably perceive field-specific medical jargon and resolve the best codes even in problematic or uncommon diagnoses and procedures.
Virtually as essential, the software program aids in audit readiness and compliance. It follows accepted billing and coding tips and saves correct information of the way in which every code was chosen. So, if there’s an audit, it’s simpler to clarify and assist correct coding choices.
High Prepared-to-Apply Medical Coding Options
At this time, the trendy software program market already affords many AI-based options for medical coding. Regardless of some shortcomings (we are going to focus on them beneath), such options will be nice for familiarization functions with such software program or as a reference for future growth.
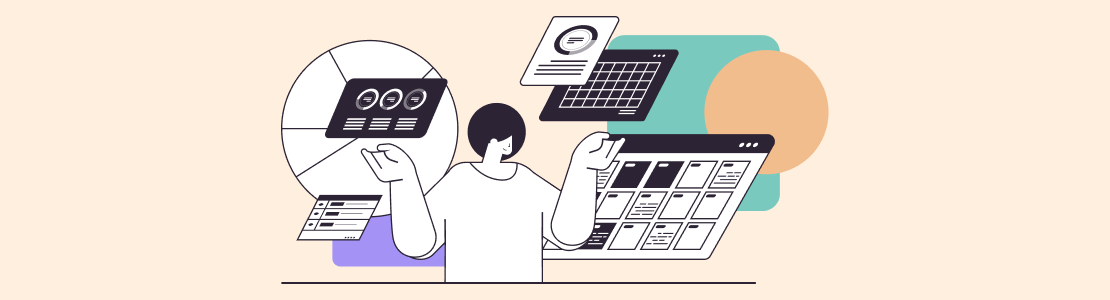
1. 3M™ CodeFinder™ and 3M™ 360 Embody™
3M is a widely known well being expertise agency. Their 360 Embody and CodeFinder options assist coders by suggesting related medical codes from medical doctors’ notes. The options can learn medical information and choose up on essential info like diagnoses and procedures.
They combine with many digital well being report programs and assist hospitals handle coding for various ranges of care. In addition they preserve detailed information to make audits simpler and assist enhance documentation high quality.
2. Optum™ Coding and Reimbursement Instruments
Optum AI coding options leverage sensible algorithms to learn affected person notes and advocate the best codes for billing. They’re appropriate for giant well being suppliers or insurance coverage firms as a result of they deal with a excessive quantity of information.
Optum’s resolution has good integration with totally different EHRs, reduces declare errors, and affords reviews to observe coding efficiency. It is usually scalable, i.e., it could possibly put up with extra sufferers if vital.
3. Cerner Code Help
Cerner’s Code Help device, in flip, is designed to work inside their very own EHR system, making it straightforward for physicians and coders to make use of with out switching between packages.
It goes via affected person information and highlights essential particulars as a way to ease the selection of appropriate codes in a well timed trend.
As a result of it’s constructed into Cerner’s system, it integrates flawlessly into each day workflow and helps to hurry up the billing course of.
4. Nuance CDE One
Nuance (now a part of Microsoft) additionally has a device that helps medical doctors write extra complete medical notes and have all the knowledge out there that’s wanted for environment friendly coding.
It affords dwell suggestions to enhance documentation, dwell medical documentation steerage, automated question era, and built-in doctor suggestions.
By and enormous, this can be a handy device for hospitals that need to improve the standard of their medical notes and on the similar time make coding extra correct. By the way in which, Nuance works with many widespread EHR programs.
5. Athenahealth Medical Coding Companies
Athenahealth gives a cloud-based coding service. It combines AI with assist from actual coding consultants, which makes it a superb different for small or medium-sized medical practices that have to outsource coding however lack the need to rent a considerable workers.
Athenahealth ensures fast and proper coding and this system interfaces with Athenahealth’s billing system and EHR.
Challenges in Off-the-Shelf AI Medical Coding
As we said earlier, pre-packaged AI medical coding software program is usually a nice technique of quickly getting began, nevertheless it additionally has some disadvantages.
One of many largest issues is that they often can’t be custom-made. Market options are usually made to accommodate everybody potential, so that you usually can’t modify how the system works to your processes, laws, or procedures.
In case your hospital or clinic does issues in a specific manner, the software program received’t give you the results you want.
One other matter is restricted integration. Pre-made instruments often join effectively with widespread EHR programs, however should you’re utilizing a much less frequent system (or a mixture of totally different instruments) they may not work with out further setup or assist from builders.
Apart from, some instruments are wonderful for small clinics however could not have the ability to course of a lot information, many customers, or multiple location. This might gradual issues down or enhance your value as you develop.
Additionally, should you use off-the-shelf software program, you must await the seller to improve it. If you need a brand new function or want to satisfy a brand new regulation, you may’t improve the software program your self, you must wait till the agency makes it out there.
Lastly, the instruments won’t be correctly skilled to take care of specialty medication. When you work in a specialty space, then the AI won’t perceive your instances correctly, and there could be coding bugs that you’d have to rectify manually.
How Customized Software program Growth Can Assist
If off-the-shelf AI medical coding software program will not be your case by a number of standards, you’d higher attempt customized healthcare software program growth particularly supposed to your calls for.
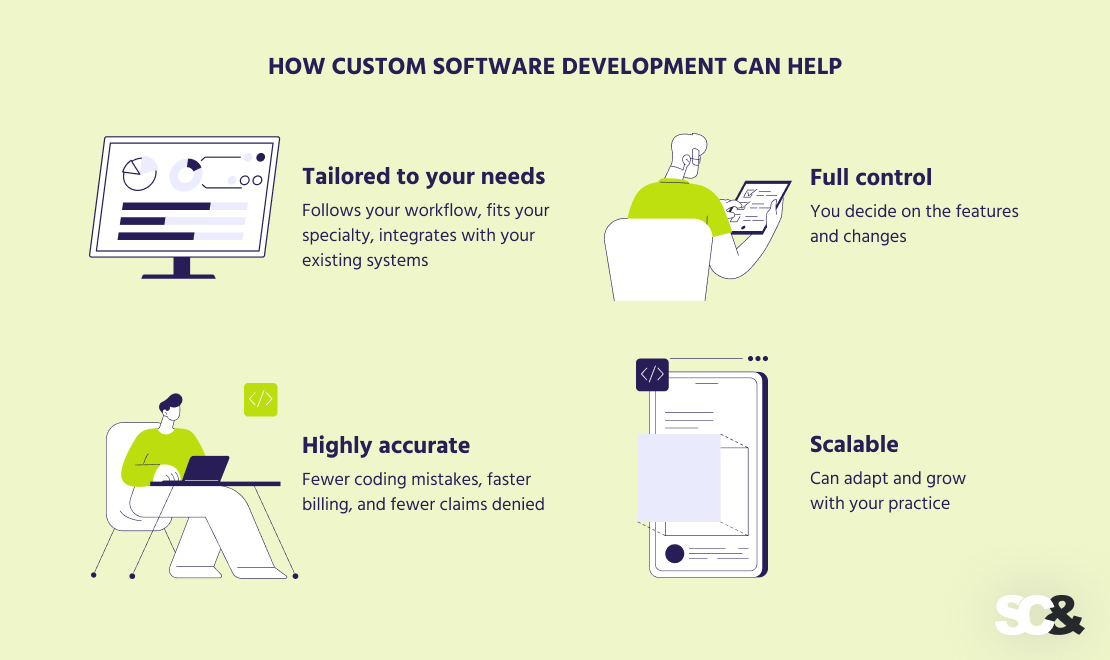
With bespoke software program, you get precisely what you want. It might probably observe your individual workflow, suit your specialty, and combine along with your present programs no matter they’re — EHR, billing system, and so on.
You’re additionally totally answerable for the options. Have to introduce one thing new? Change how one thing works? Refresh it immediately? You don’t have to attend for a vendor, you may implement a change at any time.
Personalized instruments will also be extra exact, particularly in the event that they’re skilled by yourself information. Meaning fewer coding errors, quicker billing, and fewer claims denied. That is particularly useful in case your apply handles complicated or out-of-the-ordinary instances.
One other important profit is that your software program can develop as what you are promoting does. Whether or not you’re increasing to new areas, providers, or staff, your system will be tailored and scaled to maintain up.
Sure, bespoke software program takes extra funding and time at first. However in the long term, it could possibly prevent time, cut back errors, and offer you a device that actually works the way in which you’re employed.
Key Options to Look For When Constructing Customized Software program
When you’re planning to construct your individual AI medical coding software program, it’s essential to incorporate the best options from the start.
The objective is to create a device that’s not solely sensible and correct, but additionally straightforward to make use of in real-world healthcare settings. Beneath are the options you must think about, defined in easy phrases with examples.
1. Pure Language Processing (NLP) Engine
Docs and nurses typically write medical notes in free textual content — not in neat, structured codecs. An NLP engine helps the software program learn and perceive these notes by selecting out essential particulars like signs, procedures and diagnoses, and coverings.
What it ought to do:
- Discover key phrases like “pneumonia,” “hip fracture,” or “insulin injection”
- Perceive medical abbreviations like “MI” (coronary heart assault)
- Catch context, like when a situation is barely being thought of, not confirmed
- Deal with alternative ways of claiming the identical factor (e.g., “coronary heart assault” and “myocardial infarction”)
Instance:
A physician writes: “Affected person reveals indicators of chest ache, ST elevation on ECG, and elevated troponin.”
The NLP engine ought to perceive this as a probable coronary heart assault and counsel the code for acute myocardial infarction.
2. Automated Code Strategies
Trying up medical codes manually can take a variety of time. With AI, the software program can counsel the best codes routinely, serving to coders work quicker and make fewer errors.
What it ought to do:
- Counsel the almost definitely ICD-10, CPT, or HCPCS codes
- Present a number of choices if wanted (for instance, left vs. proper facet)
- Rank codes by how assured the AI is
- Let customers approve or change ideas
Instance:
For the word “Affected person acquired steroid injection in proper shoulder,” the software program would possibly counsel:
- ICD-10: M25.511 (ache in proper shoulder)
- CPT: 20610 (injection of main joint)
3. Actual-Time Validation
Sending in claims with the improper or lacking codes can result in delays or rejections. Actual-time validation checks the codes whilst you’re working, so you may repair issues immediately.
What it ought to do:
- Warn if info is lacking
- Catch invalid code mixtures
- Comply with insurance coverage or payer guidelines
- Counsel corrections earlier than the declare is submitted
Instance:
If a process code requires an identical analysis code for approval, the system will provide you with a warning if it’s lacking earlier than the declare goes out.
4. Integration with EHR, PMS, and Billing Programs
Your coding software program ought to work effectively with the programs you already use resembling your digital well being report, apply administration system, and billing instruments to time and stop errors.
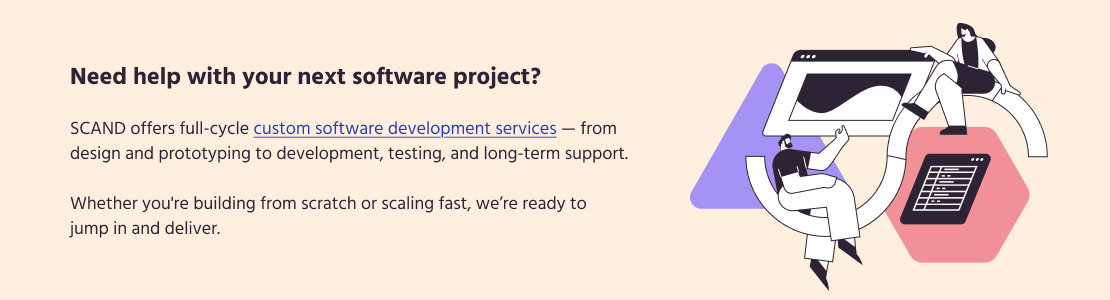
What it ought to do:
- Pull notes and affected person data from the EHR
- Push chosen codes into billing routinely
- Sync affected person information along with your apply administration system
- Preserve the whole lot related securely
Instance:
As soon as a physician finishes writing notes within the EHR, the AI device can pull the small print, counsel codes, and ship them on to billing with out anybody needing to re-enter the info.
5. Audit Path and Compliance Monitoring
In case of an audit, you might want to present how and why every code was chosen. The software program ought to observe all modifications and choices to assist show you’re following the principles.
What it ought to do:
- Document which codes have been advised and chosen
- Monitor modifications and who made them
- Retailer notes explaining why codes have been used
- Enable you to keep compliant with guidelines like HIPAA
Instance:
If a coder modifications a code advised by AI, the system saves a word like: “Modified code based mostly on physician’s clarification,” together with the date and consumer’s title.
6. Self-Studying Capabilities (Adaptive AI)
The very best AI instruments enhance over time. In case your software program can be taught out of your workforce’s corrections and suggestions, it will get smarter and extra correct and environment friendly the extra you employ it.
What it ought to do:
- Discover patterns like which codes get corrected typically
- Enhance its ideas based mostly on these corrections
- Find out how totally different suppliers doc care
- Keep updated with modifications in coding and therapy types
Instance:
If coders typically change a advised “unspecified diabetes” code to a extra particular one with problems, the software program ought to begin making that higher suggestion in future instances.
Bonus: Further Options That Can Assist
- Straightforward-to-use dashboard – So coders and managers can rapidly assessment and edit work
- Position-based entry – So totally different customers (like coders, auditors, and medical doctors) see what they want
- Multi-language assist – Helpful in clinics that serve sufferers in several languages
- Messaging instruments – Let coders and medical doctors talk contained in the platform to clear up documentation questions
Construct AI Medical Coding Software program: Step-by-Step
Creating AI-powered medical coding software program can appear overwhelming, however with the best strategy, it’s way more inside attain.
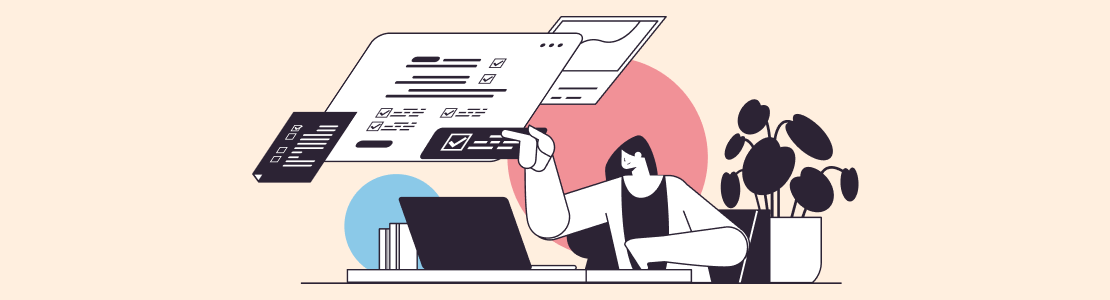
The very best factor to do is to collaborate with a software program growth firm that has expertise in healthcare and AI. With an skilled growth companion, you will be guided via each section of the method, from the very starting idea to a completed, working device.
It begins with an understanding of your wants. Your objectives might be talked about by the event workforce with you, how your workforce works, what programs you employ now, and how much coding assist you want.
This step helps create the final imaginative and prescient and element of what’s purported to be achieved by the software program.
The second step is information preparation and assortment. Since AI learns by instance, the workforce gathers actual medical paperwork and annotates them with the related medical codes.
Then, the workforce chooses essentially the most promising AI fashions and trains them. Right here, builders make the software program be taught the language of drugs and counsel the best codes relying on the contents of every word.
When the AI half is working effectively, the main target shifts to growing the consumer interface. That is the a part of this system that programmers, medical doctors, or billing workers will work together with instantly.
It should be clear, logical, and easy to navigate so folks can rapidly view ideas, enter modifications, and ship codes alongside.
The workforce checks the software program completely previous to launch. They confirm the device returns correct outcomes, meets all coding requirements, and can match into your day-to-day work.
Then comes integration and deployment. Builders combine the software program along with your different programs, say your EHR or billing system. As soon as all that’s full, it’s time to roll it out and begin utilizing it.
Lastly, even after the software program is dwell, the work doesn’t finish. A very good growth companion will assist with monitoring the device, debugging, updating, and refining the AI as you progress ahead. The extra it’s used, the smarter and extra useful it may be.
Our Experience in Medical Software program Growth
We at SCAND create in-house AI options for the healthcare trade with stress on the medical coding course of, medical processes, and direct integration with programs like EHRs and billing programs.
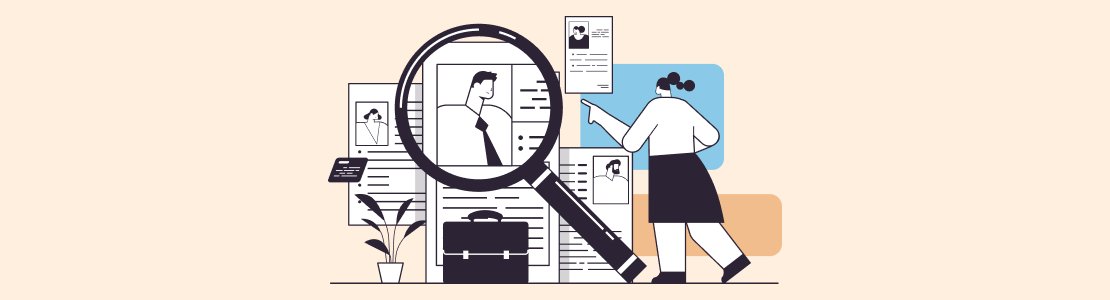
Our workforce has important publicity to healthcare and AI, permitting us to create instruments that increase coding precision, cut back guide labor, and permit medical workers to work extra productively.
We additionally observe stringent safety requirements, resembling HIPAA and GDPR to verify affected person information is safe. Concept from begin and past we empower all the growth lifecycle.
One of many tasks we undertook just lately was a lab take a look at supply sensible courier system. We constructed an internet dashboard and cell apps that make it straightforward for clinics to schedule, observe, and handle lab deliveries.
The system delivers couriers, optimizes routes, and updates everybody in actual time. This eradicated errors, saved time, and made the entire course of extra clear and dependable.
If you wish to create an analogous system or streamline any medical course of in your group, we’d be completely satisfied that will help you create an answer that matches your wants.