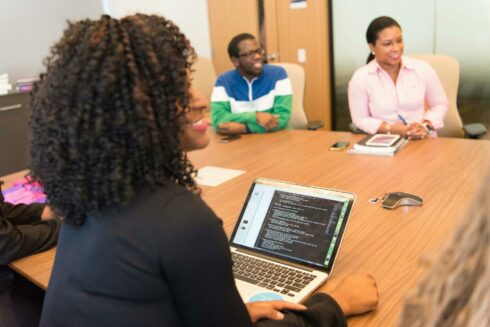
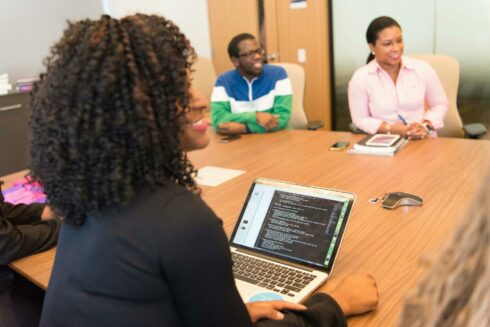
For the reason that 2001 Agile Manifesto, software program improvement has thrived on ideas like “people and interactions over processes,” steady supply, and embracing change. Over the next a long time, we watched Agile disrupt heavyweight, documentation-driven SDLCs by enabling iterative worth supply and adaptive planning. Now, quick ahead to 2025, and AI is drastically altering software program improvement. Fashions like GPT-4o and Claude 3.5 Sonnet can generate code in seconds, prompting a crucial query for trade veterans: Is Agile nonetheless related? Or have AI-driven workflows reshaped what “agile” needs to be?
AI Isn’t Killing Agile, It’s Reframing It
Generative AI instruments like Copilot and GPT-based techniques have turn out to be important in trendy developer workflows, automating routine duties and accelerating prototyping. For instance, Robinhood’s engineering groups report that nearly all of new code is generated by AI, with near-universal adoption amongst builders.
Nonetheless, this shift hasn’t spelled the top of Agile. As a substitute, it’s developed the roles of Agile practitioners like our engineers at Inflectra. Stand-up conferences, backlog grooming, and iteration planning now incorporate AI insights, which require new competencies like immediate engineering, AI validation, and threat governance.
The Productiveness Paradox
Research affirm builders utilizing AI full duties 56% sooner, whereas Atlassian experiences that builders save 10+ hours weekly due to AI, but nonetheless lose time to fragmented collaboration and data looking for.
The important thing with these modifications is to bear in mind that AI-driven velocity might breed complacency. Analysis signifies that speedy code technology can result in technical debt and lowered understanding, which manifests later in dash cycles. With out satisfactory human assessment and refactoring, your code high quality might degrade (regardless of Agile’s iterative guardrails).
The Father of Agile Weighs In
Kent Beck, co-author of the unique Agile Manifesto, compares AI brokers to genies — extremely highly effective however unpredictable. He emphasizes AI’s function in boosting creativity and enjoyable, however warns of its volatility. For Beck, Agile is now extra about imaginative and prescient, complexity administration, and human oversight than code syntax.
New AI-Pushed Agile Manifesto
Our personal Dr. Sriram Rajagopalan proposes a reimagined Agile framework tailor-made for AI-enabled improvement:
- Automated High quality Management OVER Software program Testing: AI can generate and replace check instances constantly, evolving QA as code evolves.
- Complete Documentation OVER Writing Code: With code ephemeral and reproducible on demand, human-readable architectural docs and traceability turn out to be pillars.
- Threat Administration OVER Burndown Charts: Threat scoring, compliance checks, and audit trails have to be baked into sprints for regulated industries.
- Architectural Governance OVER Code Evaluations: Sample drift and sustainability needs to be managed with automated structure enforcement (e.g. ModelOps).
This up to date framework flips Agile values to replicate present wants. AI can generate and rewrite code, so sustaining architectural integrity, security, and traceability is now crucial.
Sensible Implications for Groups
Shift in Roles & Skillsets
- Immediate Engineers turn out to be crucial for persistently getting helpful AI output. Expert prompts mix context, constraints, format, and validation directions utilizing layered buildings and function definitions. Actually, we’ve seen JSON prompts used for much more structured VEO 3 technology to reinforce replicability.
- AI Auditors / AI-Ops Specialists monitor hallucinations, correctness, drift, and safety points. They keep dashboards, post-crisis protocols, and function throughout DevOps and ModelOps domains.
Conventional Agile roles can even evolve:
- Scrum Masters can depend on AI for assembly summaries and dash metrics, however stay important as facilitators and tradition stewards.
- Product House owners can generate person tales or prototype layouts mechanically, however they nonetheless refine, prioritize, and human-validate the output to make sure alignment with the imaginative and prescient.
Backlog & Discovery Work
AI can consider epic and story high quality utilizing LLM-based high quality metrics. One other latest case examine confirmed excessive satisfaction amongst product managers refining backlog artifacts with AI help, however adoption boundaries stay. For instance, LLM brokers can generate acceptability standards, edge-case assessments, or backlog refinements, accelerating grooming periods whereas requiring human assessment.
Coding, Testing & CI/CD
- AI pair programmers like GitHub Copilot assist builders full duties sooner, leading to total developer productiveness drastically rising.
- AI-generated assessments like static evaluation, code strategies, and check case technology are constructed into pipelines. Steady testing turns into automated high quality management, shifting testing earlier and extra fluidly into improvement cycles.
Governance, Threat & Safety
- ModelOps integration ensures AI-generated outputs are monitored for equity, compliance, drift, bias, and efficiency to align with enterprise insurance policies and requirements.
- Safety turns into central through agent identities, credential dealing with, and anomaly detection to keep away from knowledge leaks or unsafe patterns. We would additionally see centralized “agent safety managers” to stability autonomy and oversight.
- Moral bias have to be surfaced in recurring backlog periods. Groups ought to schedule ethics checkpoints and assessment AI-suggested options from a number of views.
Agile Ceremonies & Metrics
- Dash planning is augmented by AI forecasting capability, estimating threat, and floor dependencies. Nonetheless, planning nonetheless depends on human discretion to anchor AI output in product imaginative and prescient and stakeholder context.
- Stand-ups and retrospectives can floor traits like immediate efficacy, code rejection charges, hallucination incidents, and group sentiment. Whereas these are automated by brokers, they need to be reviewed by people for motion objects.
- Conventional metrics like velocity or burndown give solution to AI-specific KPIs. These embody immediate success charge, check drift, refactor frequency, architectural compliance, and governance gate cross/fail charges.
Collaboration & Workflow
- AI instruments scale back cognitive load, so trendy builders spend beneath 16% of their time coding — the remaining is spent on coordination, documentation, and context retrieval. It’s because AI can’t deal with data fragmentation or management readability fairly but.
- Cross-functional alignment nonetheless must be human-led. AI might determine dependencies or backlog gaps throughout groups, however resolving them calls for negotiation and planning past what AI fashions can present.
Productiveness Positive factors vs. Hidden Prices
Vital velocity enhancements can save time and sources which might be reinvested into additional innovation as a substitute of cost-cutting. Nonetheless, elevated integration and assessment time (challenges like coordination overhead per commit) are noticed in open-source evaluation. Groups must be cognizant of dependency threat, or changing into overly reliant on AI. This might end in degradation of crucial pondering capability, so groups want to take care of assessment rituals, pair programming, and deliberate code comprehension.
Agile Isn’t Useless, It’s Evolving
Agile’s true essence lies in a mindset of adaptability, steady suggestions, and human-centric collaboration. AI doesn’t take away these values, it amplifies execution whereas heightening the necessity for human oversight in structure, threat, and moral governance.
The way forward for software program improvement isn’t Agile vs. AI, it’s Agile with AI. Strategic alignment, mentorship, and sensible governance guarantee that AI’s energy enhances security, maintainability, and long-term product worth.
Actionable Suggestions for Agile + AI
- Outline AI Governance Insurance policies: Use a 5 W’s framework (Who, What, When, The place, Why) to make clear who can use AI, for what functions, and beneath what oversight.
- Launch Pilot Agentic Pods: Small groups ought to mix human roles with AI brokers (dev, QA, backlog refinement) to check workflows.
- Observe AI-Particular Metrics: Leverage KPIs like immediate accuracy, hallucinations, check drift, and governance gate passes/fails.
- Upskill Agile Professionals: Practice Scrum Masters, Product House owners, and Architects in immediate engineering, AI auditing, and context assessment.
- Embed Steady QA & Structure Checks: Mix model-driven architectural governance with AI QA automation in CI/CD pipelines.
Agility (and software program craftsmanship) is way from out of date. It’s evolving right into a framework the place human-guided, AI-accelerated supply turns into the norm. Practitioners who embrace this hybrid mannequin will form the long run.