Since generative AI started to garner public curiosity, the pc imaginative and prescient analysis area has deepened its curiosity in creating AI fashions able to understanding and replicating bodily legal guidelines; nonetheless, the problem of educating machine studying methods to simulate phenomena reminiscent of gravity and liquid dynamics has been a big focus of analysis efforts for no less than the previous 5 years.
Since latent diffusion fashions (LDMs) got here to dominate the generative AI scene in 2022, researchers have more and more centered on LDM structure’s restricted capability to know and reproduce bodily phenomena. Now, this problem has gained extra prominence with the landmark growth of OpenAI’s generative video mannequin Sora, and the (arguably) extra consequential latest launch of the open supply video fashions Hunyuan Video and Wan 2.1.
Reflecting Badly
Most analysis aimed toward bettering LDM understanding of physics has centered on areas reminiscent of gait simulation, particle physics, and different elements of Newtonian movement. These areas have attracted consideration as a result of inaccuracies in fundamental bodily behaviors would instantly undermine the authenticity of AI-generated video.
Nevertheless, a small however rising strand of analysis concentrates on certainly one of LDM’s largest weaknesses – it is relative incapacity to provide correct reflections.
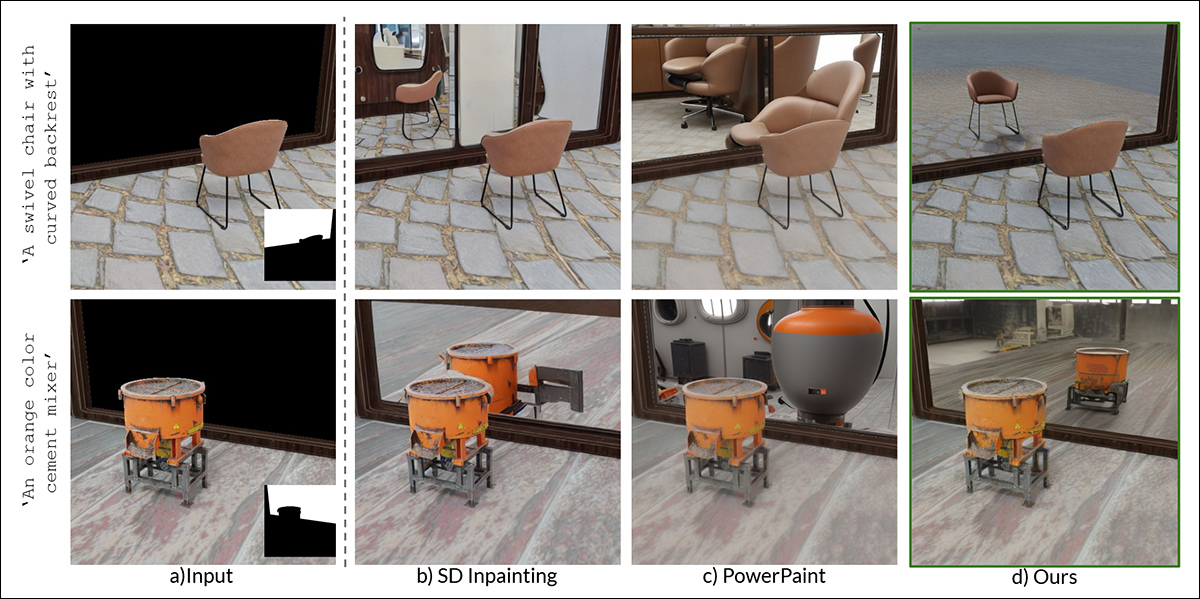
From the January 2025 paper ‘Reflecting Actuality: Enabling Diffusion Fashions to Produce Devoted Mirror Reflections’, examples of ‘reflection failure’ versus the researchers’ personal method. Supply: https://arxiv.org/pdf/2409.14677
This problem was additionally a problem through the CGI period and stays so within the area of video gaming, the place ray-tracing algorithms simulate the trail of sunshine because it interacts with surfaces. Ray-tracing calculates how digital mild rays bounce off or move via objects to create practical reflections, refractions, and shadows.
Nevertheless, as a result of every extra bounce drastically will increase computational value, real-time purposes should commerce off latency in opposition to accuracy by limiting the variety of allowed light-ray bounces.
![A representation of a virtually-calculated light-beam in a traditional 3D-based (i.e., CGI) scenario, using technologies and principles first developed in the 1960s, and which came to fulmination between 1982-93 (the span between Tron [1982] and Jurassic Park [1993]. Source: https://www.unrealengine.com/en-US/explainers/ray-tracing/what-is-real-time-ray-tracing](https://www.unite.ai/wp-content/uploads/2025/04/ray-tracing.jpg)
A illustration of a virtually-calculated light-beam in a conventional 3D-based (i.e., CGI) situation, utilizing applied sciences and ideas first developed within the Sixties, and which got here to fulmination between 1982-93 (the span between ‘Tron’ [1982] and ‘Jurassic Park’ [1993]. Supply: https://www.unrealengine.com/en-US/explainers/ray-tracing/what-is-real-time-ray-tracing
For example, depicting a chrome teapot in entrance of a mirror may contain a ray-tracing course of the place mild rays bounce repeatedly between reflective surfaces, creating an nearly infinite loop with little sensible profit to the ultimate picture. Generally, a mirrored image depth of two to 3 bounces already exceeds what the viewer can understand. A single bounce would end in a black mirror, because the mild should full no less than two journeys to kind a visual reflection.
Every extra bounce sharply will increase computational value, typically doubling render occasions, making quicker dealing with of reflections one of the vital important alternatives for bettering ray-traced rendering high quality.
Naturally, reflections happen, and are important to photorealism, in far much less apparent situations – such because the reflective floor of a metropolis road or a battlefield after the rain; the reflection of the opposing road in a store window or glass doorway; or within the glasses of depicted characters, the place objects and environments could also be required to look.
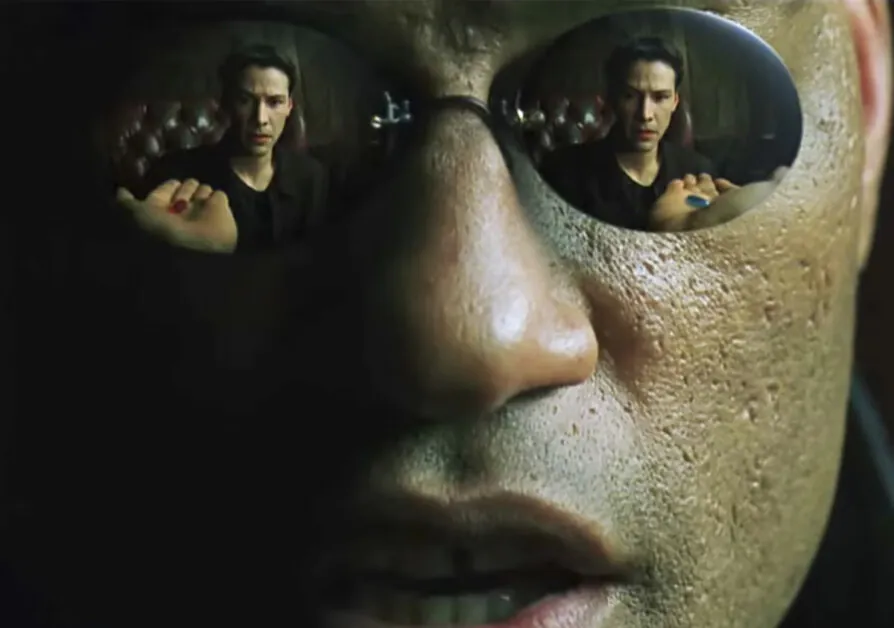
A simulated twin-reflection achieved by way of conventional compositing for an iconic scene in ‘The Matrix’ (1999).
Picture Issues
Because of this, frameworks that have been common previous to the appearance of diffusion fashions, reminiscent of Neural Radiance Fields (NeRF), and a few more moderen challengers reminiscent of Gaussian Splatting have maintained their very own struggles to enact reflections in a pure method.
The REF2-NeRF mission (pictured under) proposed a NeRF-based modeling technique for scenes containing a glass case. On this technique, refraction and reflection have been modeled utilizing parts that have been dependent and unbiased of the viewer’s perspective. This method allowed the researchers to estimate the surfaces the place refraction occurred, particularly glass surfaces, and enabled the separation and modeling of each direct and mirrored mild elements.
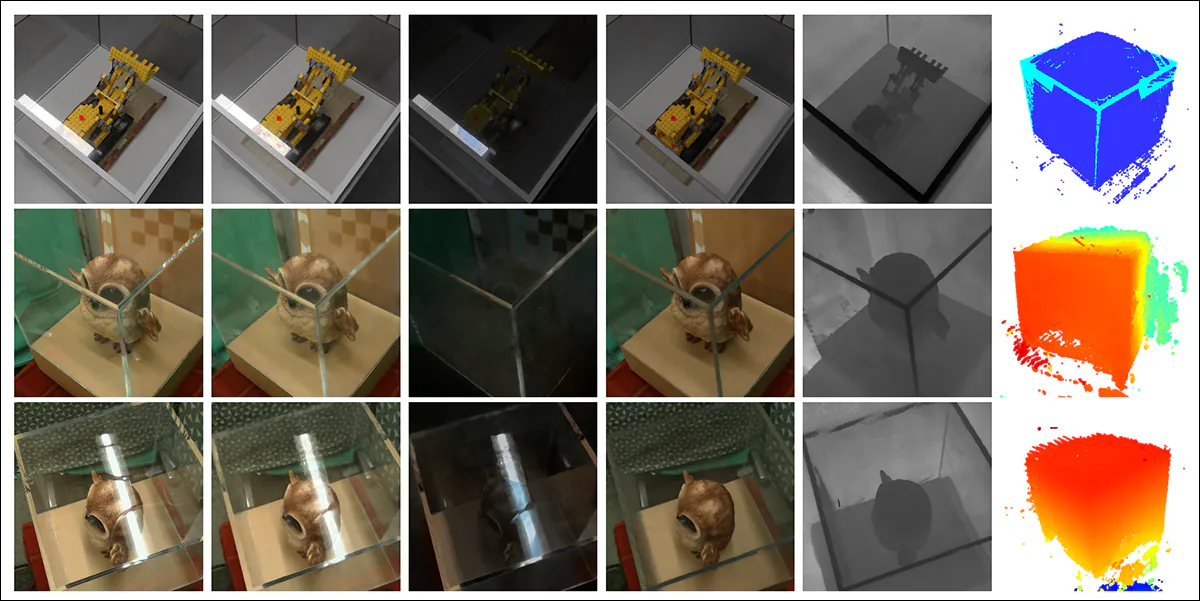
Examples from the Ref2Nerf paper. Supply: https://arxiv.org/pdf/2311.17116
Different NeRF-facing reflection options of the final 4-5 years have included NeRFReN, Reflecting Actuality, and Meta’s 2024 Planar Reflection-Conscious Neural Radiance Fields mission.
For GSplat, papers reminiscent of Mirror-3DGS, Reflective Gaussian Splatting, and RefGaussian have supplied options concerning the reflection drawback, whereas the 2023 Nero mission proposed a bespoke technique of incorporating reflective qualities into neural representations.
MirrorVerse
Getting a diffusion mannequin to respect reflection logic is arguably tougher than with explicitly structural, non-semantic approaches reminiscent of Gaussian Splatting and NeRF. In diffusion fashions, a rule of this type is simply prone to grow to be reliably embedded if the coaching information accommodates many assorted examples throughout a variety of situations, making it closely depending on the distribution and high quality of the unique dataset.
Historically, including specific behaviors of this type is the purview of a LoRA or the fine-tuning of the bottom mannequin; however these will not be superb options, since a LoRA tends to skew output in direction of its personal coaching information, even with out prompting, whereas fine-tunes – apart from being costly – can fork a serious mannequin irrevocably away from the mainstream, and engender a bunch of associated customized instruments that can by no means work with any different pressure of the mannequin, together with the unique one.
On the whole, bettering diffusion fashions requires that the coaching information pay higher consideration to the physics of reflection. Nevertheless, many different areas are additionally in want of comparable particular consideration. Within the context of hyperscale datasets, the place customized curation is expensive and troublesome, addressing each single weak point on this method is impractical.
Nonetheless, options to the LDM reflection drawback do crop up every so often. One latest such effort, from India, is the MirrorVerse mission, which provides an improved dataset and coaching technique able to bettering of the state-of-the-art on this specific problem in diffusion analysis.
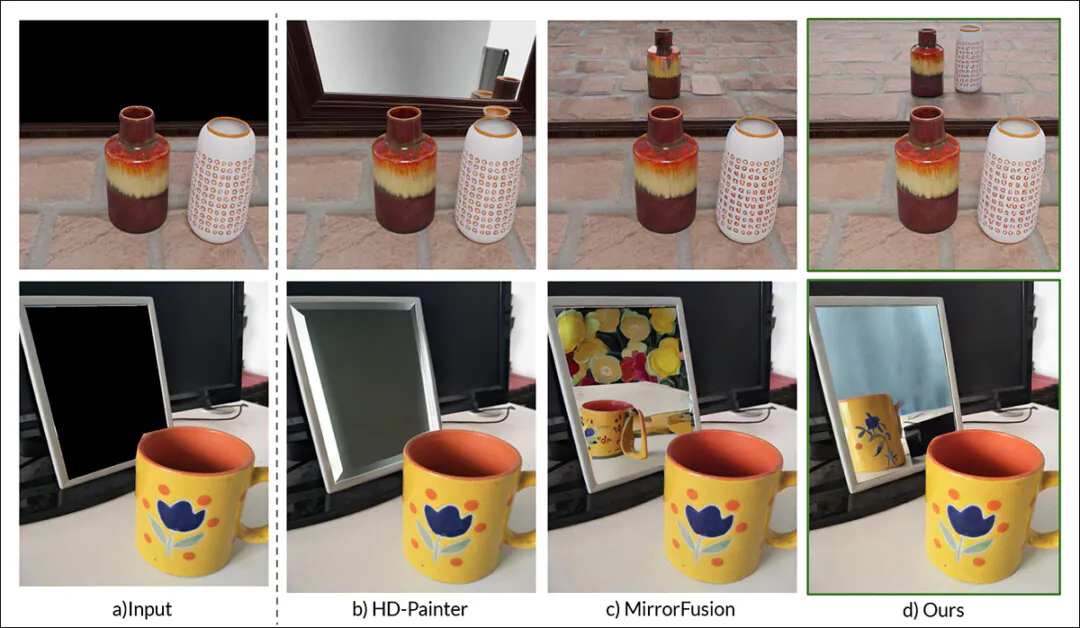
Rightmost, the outcomes from MirrorVerse pitted in opposition to two prior approaches (central two columns). Supply: https://arxiv.org/pdf/2504.15397
As we will see within the instance above (the function picture within the PDF of the brand new research), MirrorVerse improves on latest choices tackling the identical drawback, however is much from excellent.
Within the higher proper picture, we see that the ceramic jars are considerably to the correct of the place they need to be, and within the picture under, which ought to technically not function a mirrored image of the cup in any respect, an inaccurate reflection has been shoehorned into the correct–hand space, in opposition to the logic of pure reflective angles.
Due to this fact we’ll check out the brand new technique not a lot as a result of it could signify the present state-of-the-art in diffusion-based reflection, however equally as an example the extent to which this will likely show to be an intractable problem for latent diffusion fashions, static and video alike, because the requisite information examples of reflectivity are most probably to be entangled with specific actions and situations.
Due to this fact this specific perform of LDMs might proceed to fall in need of structure-specific approaches reminiscent of NeRF, GSplat, and likewise conventional CGI.
The new paper is titled MirrorVerse: Pushing Diffusion Fashions to Realistically Replicate the World, and comes from three researchers throughout Imaginative and prescient and AI Lab, IISc Bangalore, and the Samsung R&D Institute at Bangalore. The paper has an related mission web page, in addition to a dataset at Hugging Face, with supply code launched at GitHub.
Technique
The researchers be aware from the outset the issue that fashions reminiscent of Secure Diffusion and Flux have in respecting reflection-based prompts, illustrating the problem adroitly:
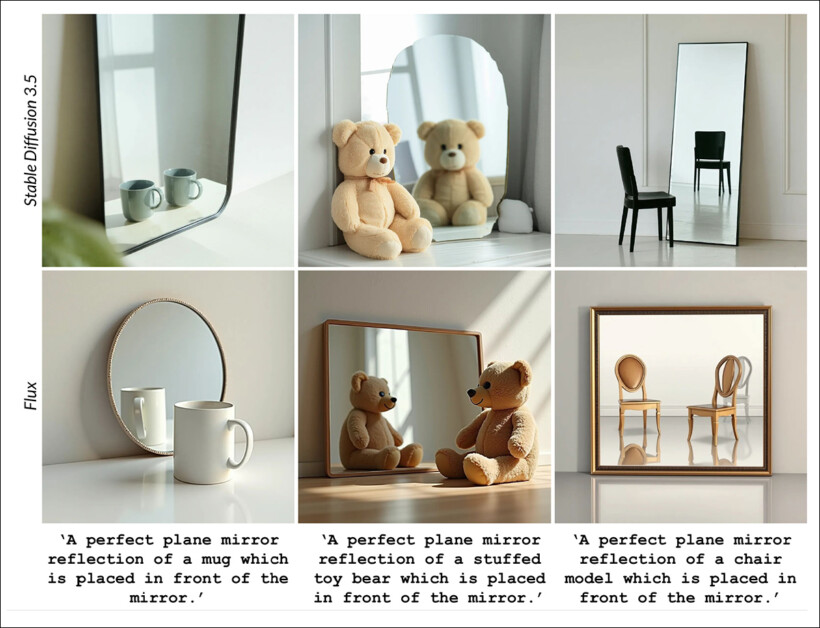
From the paper: Present state-of-the-art text-to-image fashions, SD3.5 and Flux, exhibiting important challenges in producing constant and geometrically correct reflections when prompted to generate them in a scene.
The researchers have developed MirrorFusion 2.0, a diffusion-based generative mannequin aimed toward bettering the photorealism and geometric accuracy of mirror reflections in artificial imagery. Coaching for the mannequin was based mostly on the researchers’ personal newly-curated dataset, titled MirrorGen2, designed to deal with the generalization weaknesses noticed in earlier approaches.
MirrorGen2 expands on earlier methodologies by introducing random object positioning, randomized rotations, and express object grounding, with the aim of making certain that reflections stay believable throughout a wider vary of object poses and placements relative to the mirror floor.
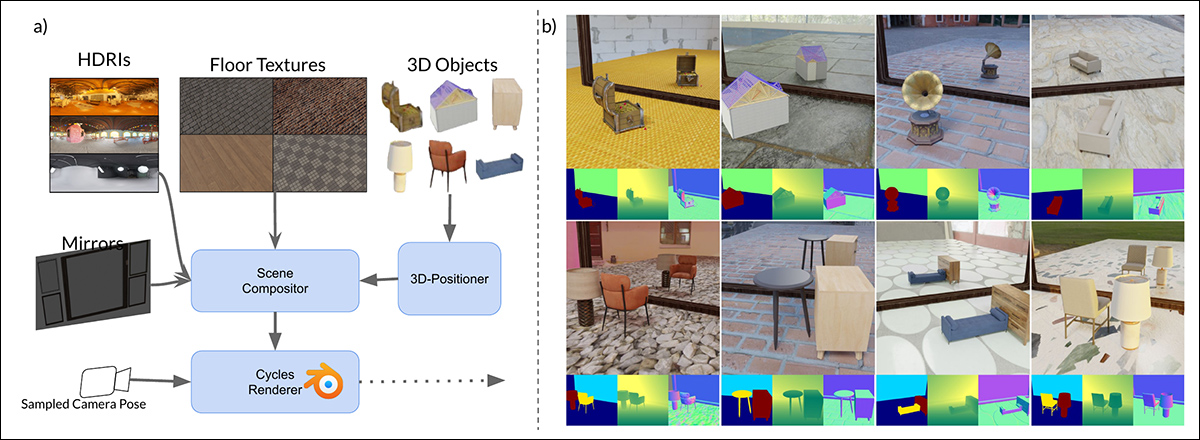
Schema for the era of artificial information in MirrorVerse: the dataset era pipeline utilized key augmentations by randomly positioning, rotating, and grounding objects inside the scene utilizing the 3D-Positioner. Objects are additionally paired in semantically constant mixtures to simulate advanced spatial relationships and occlusions, permitting the dataset to seize extra practical interactions in multi-object scenes.
To additional strengthen the mannequin’s skill to deal with advanced spatial preparations, the MirrorGen2 pipeline incorporates paired object scenes, enabling the system to raised signify occlusions and interactions between a number of parts in reflective settings.
The paper states:
‘Classes are manually paired to make sure semantic coherence – for example, pairing a chair with a desk. Throughout rendering, after positioning and rotating the first [object], an extra [object] from the paired class is sampled and organized to stop overlap, making certain distinct spatial areas inside the scene.’
In regard to express object grounding, right here the authors ensured that the generated objects have been ‘anchored’ to the bottom within the output artificial information, somewhat than ‘hovering’ inappropriately, which may happen when artificial information is generated at scale, or with extremely automated strategies.
Since dataset innovation is central to the novelty of the paper, we’ll proceed sooner than typical to this part of the protection.
Information and Checks
SynMirrorV2
The researchers’ SynMirrorV2 dataset was conceived to enhance the range and realism of mirror reflection coaching information, that includes 3D objects sourced from the Objaverse and Amazon Berkeley Objects (ABO) datasets, with these picks subsequently refined via OBJECT 3DIT, in addition to the filtering course of from the V1 MirrorFusion mission, to remove low-quality asset. This resulted in a refined pool of 66,062 objects.
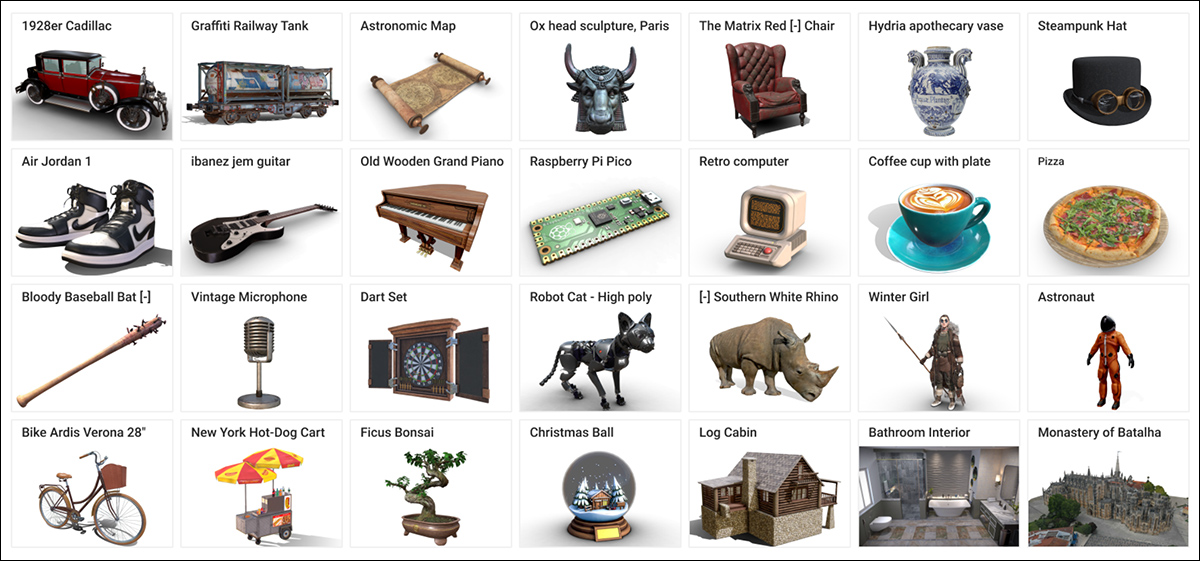
Examples from the Objaverse dataset, used within the creation of the curated dataset for the brand new system. Supply: https://arxiv.org/pdf/2212.08051
Scene development concerned inserting these objects onto textured flooring from CC-Textures and HDRI backgrounds from the PolyHaven CGI repository, utilizing both full-wall or tall rectangular mirrors. Lighting was standardized with an area-light positioned above and behind the objects, at a forty-five diploma angle. Objects have been scaled to suit inside a unit dice and positioned utilizing a precomputed intersection of the mirror and digital camera viewing frustums, making certain visibility.
Randomized rotations have been utilized across the y-axis, and a grounding method used to stop ‘floating artifacts’.
To simulate extra advanced scenes, the dataset additionally integrated a number of objects organized in accordance with semantically coherent pairings based mostly on ABO classes. Secondary objects have been positioned to keep away from overlap, creating 3,140 multi-object scenes designed to seize assorted occlusions and depth relationships.
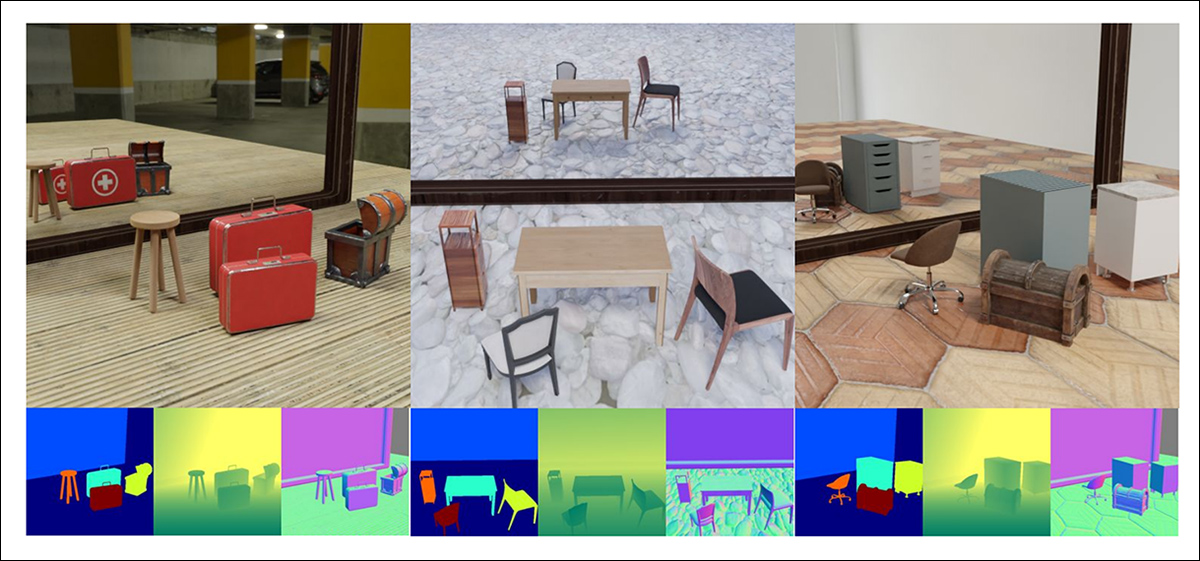
Examples of rendered views from the authors’ dataset containing a number of (greater than two) objects, with illustrations of object segmentation and depth map visualizations seen under.
Coaching Course of
Acknowledging that artificial realism alone was inadequate for sturdy generalization to real-world information, the researchers developed a three-stage curriculum studying course of for coaching MirrorFusion 2.0.
In Stage 1, the authors initialized the weights of each the conditioning and era branches with the Secure Diffusion v1.5 checkpoint, and fine-tuned the mannequin on the single-object coaching break up of the SynMirrorV2 dataset. Not like the above-mentioned Reflecting Actuality mission, the researchers didn’t freeze the era department. They then educated the mannequin for 40,000 iterations.
In Stage 2, the mannequin was fine-tuned for an extra 10,000 iterations, on the multiple-object coaching break up of SynMirrorV2, with a view to train the system to deal with occlusions, and the extra advanced spatial preparations present in practical scenes.
Lastly, In Stage 3, an extra 10,000 iterations of finetuning have been performed utilizing real-world information from the MSD dataset, utilizing depth maps generated by the Matterport3D monocular depth estimator.
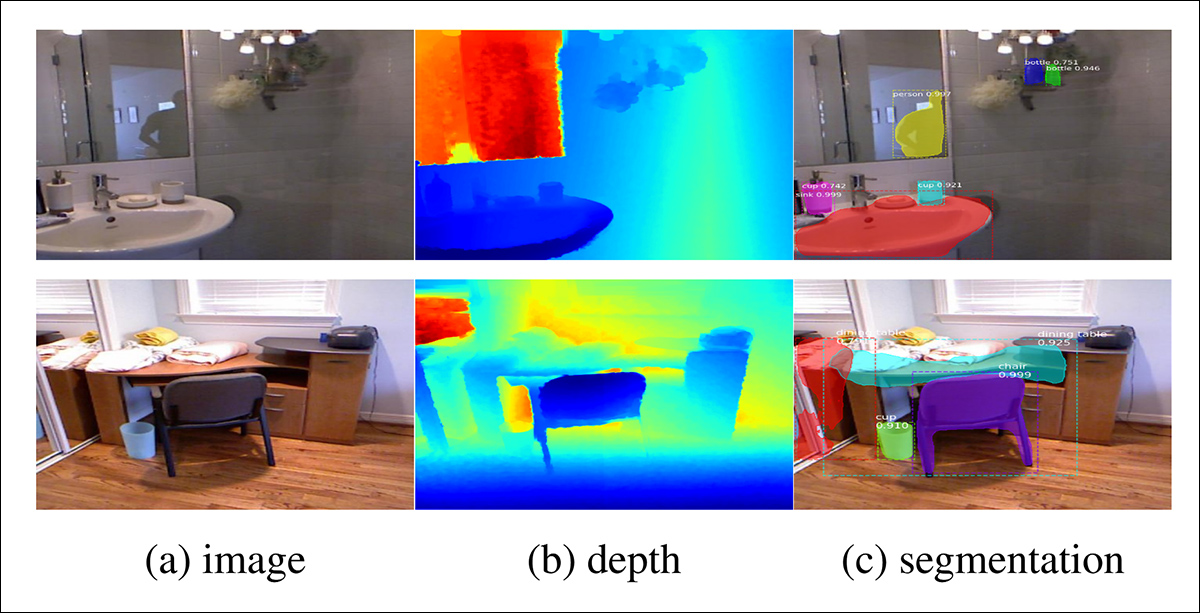
Examples from the MSD dataset, with real-world scenes analyzed into depth and segmentation maps. Supply: https://arxiv.org/pdf/1908.09101
Throughout coaching, textual content prompts have been omitted for 20 p.c of the coaching time with a view to encourage the mannequin to make optimum use of the out there depth data (i.e., a ‘masked’ method).
Coaching happened on 4 NVIDIA A100 GPUs for all phases (the VRAM spec is just not equipped, although it might have been 40GB or 80GB per card). A studying fee of 1e-5 was used on a batch measurement of 4 per GPU, beneath the AdamW optimizer.
This coaching scheme progressively elevated the issue of duties introduced to the mannequin, starting with easier artificial scenes and advancing towards more difficult compositions, with the intention of creating sturdy real-world transferability.
Testing
The authors evaluated MirrorFusion 2.0 in opposition to the earlier state-of-the-art, MirrorFusion, which served because the baseline, and performed experiments on the MirrorBenchV2 dataset, overlaying each single and multi-object scenes.
Further qualitative exams have been performed on samples from the MSD dataset, and the Google Scanned Objects (GSO) dataset.
The analysis used 2,991 single-object pictures from seen and unseen classes, and 300 two-object scenes from ABO. Efficiency was measured utilizing Peak Sign-to-Noise Ratio (PSNR); Structural Similarity Index (SSIM); and Discovered Perceptual Picture Patch Similarity (LPIPS) scores, to evaluate reflection high quality on the masked mirror area. CLIP similarity was used to judge textual alignment with the enter prompts.
In quantitative exams, the authors generated pictures utilizing 4 seeds for a selected immediate, and deciding on the ensuing picture with one of the best SSIM rating. The 2 reported tables of outcomes for the quantitative exams are proven under.

Left, Quantitative outcomes for single object reflection era high quality on the MirrorBenchV2 single object break up. MirrorFusion 2.0 outperformed the baseline, with one of the best outcomes proven in daring. Proper, quantitative outcomes for a number of object reflection era high quality on the MirrorBenchV2 a number of object break up. MirrorFusion 2.0 educated with a number of objects outperformed the model educated with out them, with one of the best outcomes proven in daring.
The authors remark:
‘[The results] present that our technique outperforms the baseline technique and finetuning on a number of objects improves the outcomes on advanced scenes.’
The majority of outcomes, and people emphasised by the authors, regard qualitative testing. As a result of dimensions of those illustrations, we will solely partially reproduce the paper’s examples.
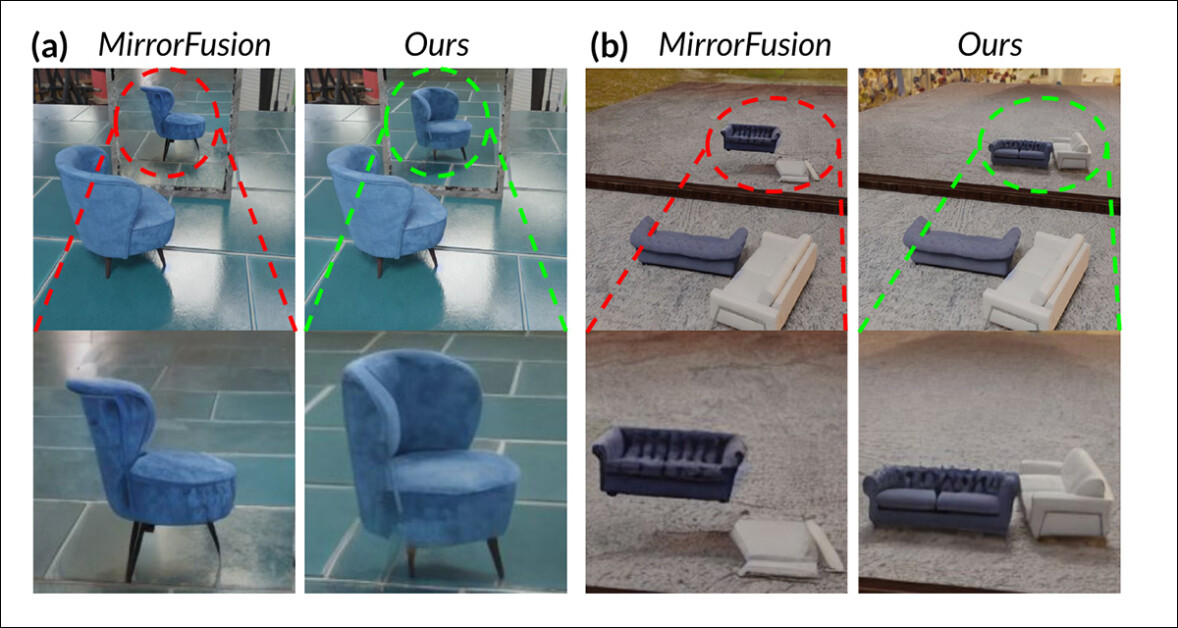
Comparability on MirrorBenchV2: the baseline failed to keep up correct reflections and spatial consistency, displaying incorrect chair orientation and distorted reflections of a number of objects, whereas (the authors contend) MirrorFusion 2.0 accurately renders the chair and the sofas, with correct place, orientation, and construction.
Of those subjective outcomes, the researchers opine that the baseline mannequin didn’t precisely render object orientation and spatial relationships in reflections, typically producing artifacts reminiscent of incorrect rotation and floating objects. MirrorFusion 2.0, educated on SynMirrorV2, the authors contend, preserves appropriate object orientation and positioning in each single-object and multi-object scenes, leading to extra practical and coherent reflections.
Under we see qualitative outcomes on the aforementioned GSO dataset:
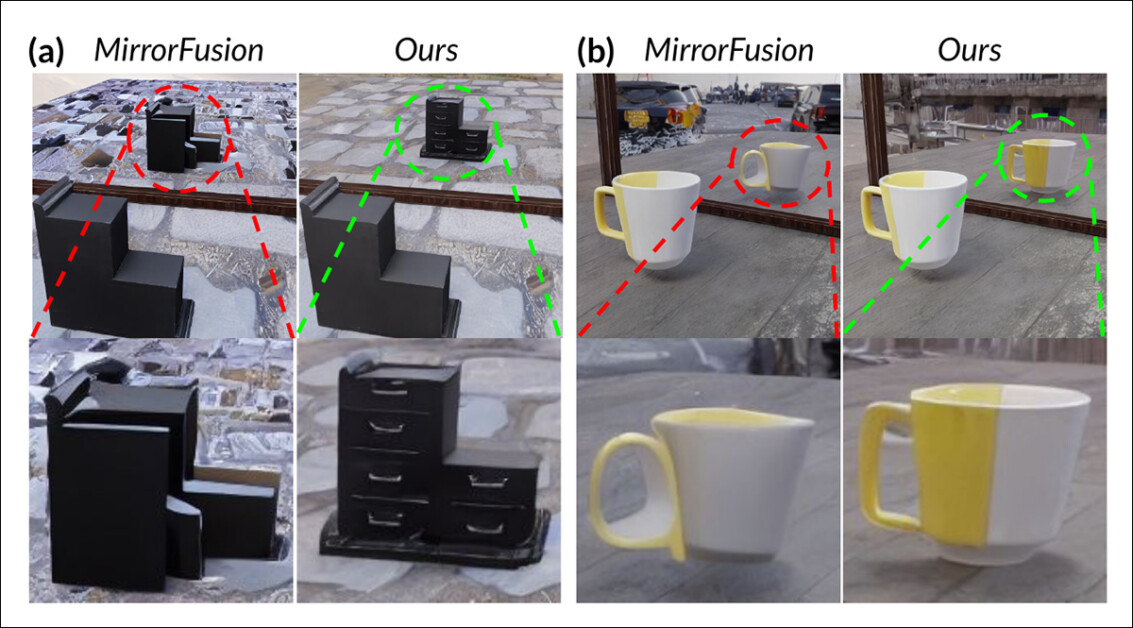
Comparability on the GSO dataset. The baseline misrepresents object construction and produced incomplete, distorted reflections, whereas MirrorFusion 2.0, the authors contend, preserves spatial integrity and generates correct geometry, colour, and element, even on out-of-distribution objects.
Right here the authors remark:
‘MirrorFusion 2.0 generates considerably extra correct and practical reflections. For example, in Fig. 5 (a – above), MirrorFusion 2.0 accurately displays the drawer handles (highlighted in inexperienced), whereas the baseline mannequin produces an implausible reflection (highlighted in purple).
‘Likewise, for the “White-Yellow mug” in Fig. 5 (b), MirrorFusion 2.0 delivers a convincing geometry with minimal artifacts, in contrast to the baseline, which fails to precisely seize the item’s geometry and look.’
The ultimate qualitative take a look at was in opposition to the aforementioned real-world MSD dataset (partial outcomes proven under):
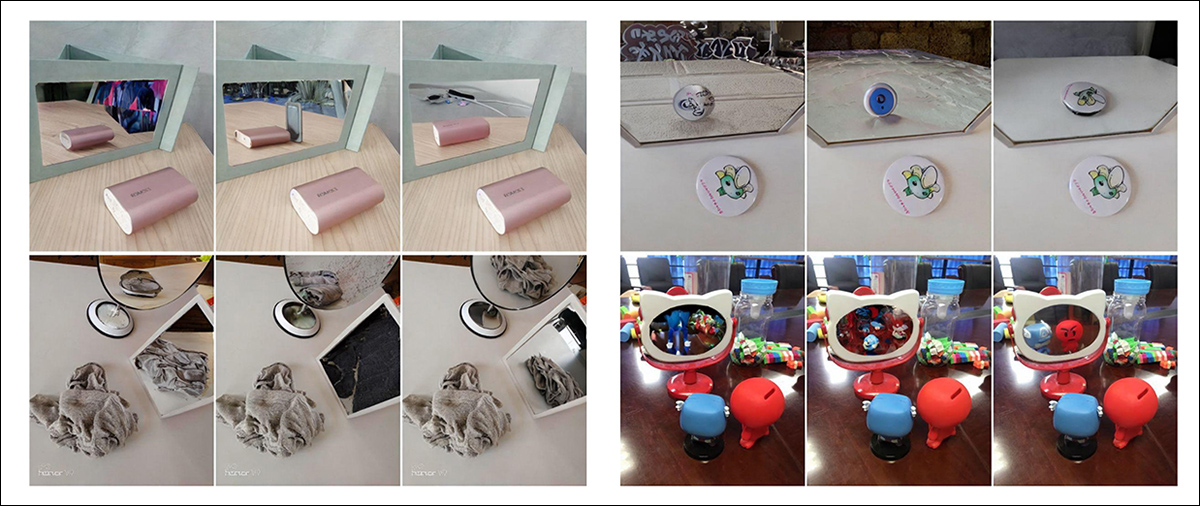
Actual-world scene outcomes evaluating MirrorFusion, MirrorFusion 2.0, and MirrorFusion 2.0, fine-tuned on the MSD dataset. MirrorFusion 2.0, the authors contend, captures advanced scene particulars extra precisely, together with cluttered objects on a desk, and the presence of a number of mirrors inside a three-dimensional surroundings. Solely partial outcomes are proven right here, as a result of dimensions of the ends in the unique paper, to which we refer the reader for full outcomes and higher decision.
Right here the authors observe that whereas MirrorFusion 2.0 carried out properly on MirrorBenchV2 and GSO information, it initially struggled with advanced real-world scenes within the MSD dataset. Advantageous-tuning the mannequin on a subset of MSD improved its skill to deal with cluttered environments and a number of mirrors, leading to extra coherent and detailed reflections on the held-out take a look at break up.
Moreover, a consumer research was performed, the place 84% of customers are reported to have most well-liked generations from MirrorFusion 2.0 over the baseline technique.
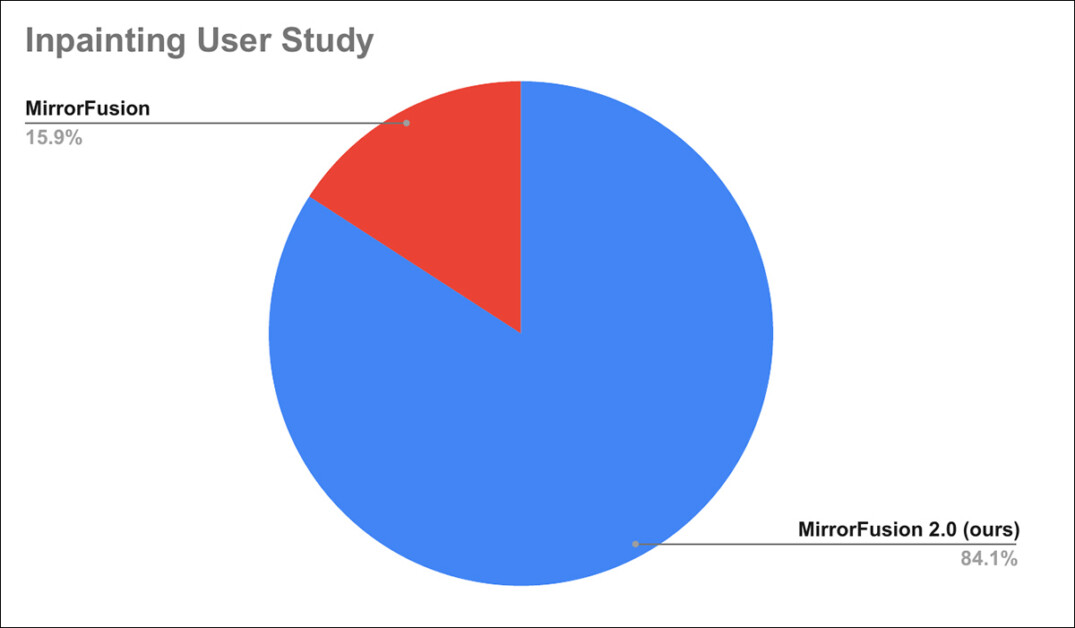
Outcomes of the consumer research.
Since particulars of the consumer research have been relegated to the appendix of the paper, we refer the reader to that for the specifics of the research.
Conclusion
Though a number of of the outcomes proven within the paper are spectacular enhancements on the state-of-the-art, the state-of-the-art for this specific pursuit is so abysmal that even an unconvincing mixture answer can win out with a modicum of effort. The elemental structure of a diffusion mannequin is so inimical to the dependable studying and demonstration of constant physics, that the issue itself is really posed, and never apparently not disposed towards a chic answer.
Additional, including information to current fashions is already the usual technique of remedying shortfalls in LDM efficiency, with all of the disadvantages listed earlier. It’s affordable to imagine that if future high-scale datasets have been to pay extra consideration to the distribution (and annotation) of reflection-related information factors, we may anticipate that the ensuing fashions would deal with this situation higher.
But the identical is true of a number of different bugbears in LDM output – who can say which ones most deserves the trouble and cash concerned within the form of answer that the authors of the brand new paper suggest right here?
First printed Monday, April 28, 2025