A collaboration between researchers in the US and Canada has discovered that giant language fashions (LLMs) corresponding to ChatGPT battle to breed historic idioms with out in depth pretraining – a expensive and labor-intensive course of that lies past the technique of most educational or leisure initiatives, making initiatives corresponding to finishing Charles Dickens’s ultimate, unfinished novel successfully by means of AI an unlikely proposition.
The researchers explored a spread of strategies for producing textual content that sounded traditionally correct, beginning with easy prompting utilizing early twentieth-century prose, and transferring to fine-tuning a business mannequin on a small assortment of books from that interval.
Additionally they in contrast the outcomes to a separate mannequin that had been skilled completely on books printed between 1880 and 1914.
Within the first of the exams, instructing ChatGPT-4o to imitate fin‑de‑siècle language produced fairly completely different outcomes from these of the smaller GPT2-based mannequin that had been fantastic‑tuned on literature from the interval:
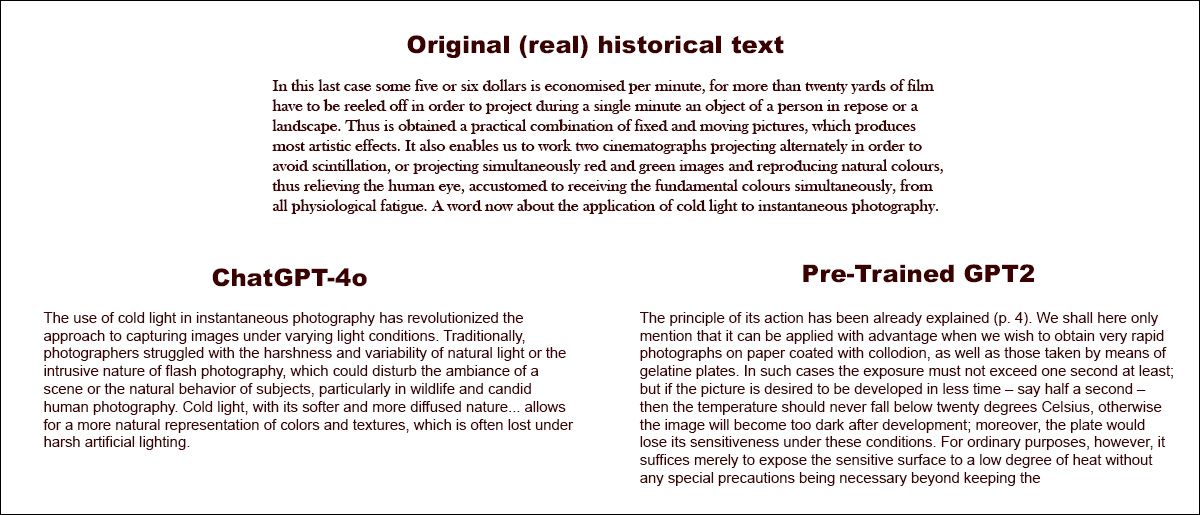
Requested to finish an actual historic textual content (top-center), even a well-primed ChatGPT-4o (decrease left) can not assist lapsing again into ‘weblog’ mode, failing to symbolize the requested idiom. In contrast, the fine-tuned GPT2 mannequin (decrease proper) captures the language fashion properly, however isn’t as correct in different methods. Supply: https://arxiv.org/pdf/2505.00030
Although fine-tuning brings the output nearer to the unique fashion, human readers had been nonetheless incessantly capable of detect traces of recent language or concepts, suggesting that even carefully-adjusted fashions proceed to mirror the affect of their up to date coaching knowledge.
The researchers arrive on the irritating conclusion that there are not any economical short-cuts in direction of the era of machine-produced idiomatically-correct historic textual content or dialogue. Additionally they conjecture that the problem itself is likely to be ill-posed:
‘[We] must also think about the chance that anachronism could also be in some sense unavoidable. Whether or not we symbolize the previous by instruction-tuning historic fashions to allow them to maintain conversations, or by educating up to date fashions to ventriloquize an older interval, some compromise could also be essential between the targets of authenticity and conversational fluency.
‘There are, in spite of everything, no “genuine” examples of a dialog between a twenty-first-century questioner and a respondent from 1914. Researchers making an attempt to create such a dialog might want to mirror on the [premise] that interpretation all the time includes a negotiation between current and [past].’
The new examine is titled Can Language Fashions Signify the Previous with out Anachronism?, and comes from three researchers throughout College of Illinois, College of British Columbia, and Cornell College.
Full Catastrophe
Initially, in a three-part analysis strategy, the authors examined whether or not trendy language fashions could possibly be nudged into mimicking historic language by means of easy prompting. Utilizing actual excerpts from books printed between 1905 and 1914, they requested ChatGPT‑4o to proceed these passages in the identical idiom.
The unique interval textual content was:
‘On this final case some 5 or 6 {dollars} is economised per minute, for greater than twenty yards of movie must be reeled off so as to undertaking throughout a single minute an object of an individual in repose or a panorama. Thus is obtained a sensible mixture of fastened and transferring photos, which produces most creative results.
‘It additionally permits us to work two cinematographs projecting alternately so as to keep away from scintillation, or projecting concurrently purple and inexperienced photographs and reproducing pure colors, thus relieving the human eye, accustomed to receiving the elemental colors concurrently, from all physiological fatigue. A phrase now concerning the utility of chilly mild to instantaneous pictures.’
To guage whether or not the generated textual content matched the meant historic fashion, and aware that individuals are not particularly expert at guessing the date {that a} textual content was written, the researchers fantastic‑tuned a RoBERTa mannequin to estimate publication dates, utilizing a subset of the Corpus of Historic American English, masking materials from 1810 to 2009.
The RoBERTa classifier was then used to evaluate continuations produced by ChatGPT‑4o, which had been prompted with actual passages from books printed between 1905 and 1914.
The system immediate (i.e., contextual directions to ChatGPT about strategy the duty) was:
‘Your job is to finish passages from early twentieth-century books. You may be given a passage from a e book printed in 1913. Proceed this passage in the identical fashion, for no less than 200 phrases. Solely present this continuation; don’t make any framing remarks, like ’right here is the continuation:’.
Regardless of each one-shot and 20-shot prompting, ChatGPT-4o’s outputs persistently skewed towards a Twenty first-century stylistic register.
The instance given within the paper options considered one of ChatGPT-4o’s blog-like makes an attempt to proceed the true interval textual content about pictures:
‘Using chilly mild in instantaneous pictures has revolutionized the strategy to capturing photographs underneath various mild circumstances. Historically, photographers struggled with the harshness and variability of pure mild or the intrusive nature of flash pictures, which might disturb the ambiance of a scene or the pure conduct of topics, notably in wildlife and candid human pictures.
‘Chilly mild, with its softer and extra subtle nature… permits for a extra pure illustration of colours and textures, which is usually misplaced underneath harsh synthetic lighting.’
As we will simply see, this generic, Wiki-style textual content doesn’t match the prolix and elaborate fashion of the unique interval textual content. Additional, it does not likely proceed from the place the unique content material leaves off, however launches right into a tangential, summary musing on one of many sub-topics.
The staff additionally examined GPT‑1914, a smaller GPT‑2–class mannequin skilled from scratch on 26.5 billion tokens of literature dated between 1880 and 1914.
Although its output was much less coherent than that of ChatGPT‑4o, it was extra per the fashion of the supply interval. The only real instance supplied within the paper, once more as a completion of the real-world interval textual content on pictures, reads:
‘The precept of its motion has been already defined (p. 4). We will right here solely point out that it may be utilized with benefit after we want to get hold of very speedy pictures on paper coated with collodion, in addition to these taken via gelatine plates.
‘In such instances the publicity should not exceed one second no less than; but when the image is desired to be developed in much less time – say half a second – then the temperature ought to by no means fall under 20° C., in any other case the picture will turn out to be too darkish after growth; furthermore, the plate would lose its sensitiveness underneath these circumstances.
‘For strange functions, nonetheless, it suffices merely to reveal the delicate floor to a low diploma of warmth with none particular precautions being essential past maintaining the’ [sic]
Since even the true and authentic materials is arcane and fairly tough to comply with, it’s exhausting to grasp the extent to which GPT-1914 has precisely picked up from the unique; however the output actually sounds extra period-authentic.
Nonetheless, the authors concluded from this experiment that easy prompting does little to beat the up to date biases of a big pretrained mannequin corresponding to ChatGPT-4o.
The Plot Thickens
To measure how carefully the mannequin outputs resembled genuine historic writing, the researchers used a statistical classifier to estimate the doubtless publication date of every textual content pattern. They then visualized the outcomes utilizing a kernel density plot, which exhibits the place the mannequin thinks every passage falls on a historic timeline.
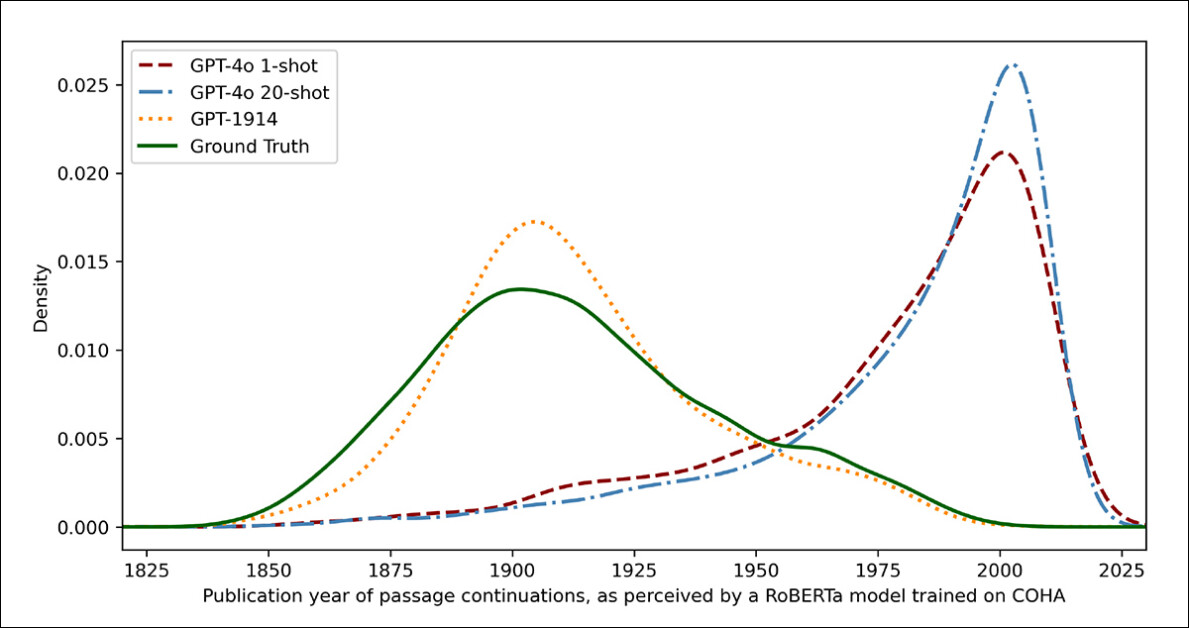
Estimated publication dates for actual and generated textual content, primarily based on a classifier skilled to acknowledge historic fashion (1905–1914 supply texts in contrast with continuations by GPT‑4o utilizing one-shot and 20-shot prompts, and by GPT‑1914 skilled solely on literature from 1880–1914).
The fantastic‑tuned RoBERTa mannequin used for this job, the authors word, isn’t flawless, however was nonetheless capable of spotlight normal stylistic developments. Passages written by GPT‑1914, the mannequin skilled completely on interval literature, clustered across the early twentieth century – much like the unique supply materials.
In contrast, ChatGPT-4o’s outputs, even when prompted with a number of historic examples, tended to resemble twenty‑first‑century writing, reflecting the information it was initially skilled on.
The researchers quantified this mismatch utilizing Jensen-Shannon divergence, a measure of how completely different two likelihood distributions are. GPT‑1914 scored a detailed 0.006 in comparison with actual historic textual content, whereas ChatGPT‑4o’s one-shot and 20-shot outputs confirmed a lot wider gaps, at 0.310 and 0.350 respectively.
The authors argue that these findings point out prompting alone, even with a number of examples, isn’t a dependable technique to produce textual content that convincingly simulates a historic fashion.
Finishing the Passage
The paper then investigates whether or not fine-tuning may produce a superior outcome, since this course of includes instantly affecting the usable weights of a mannequin by ‘persevering with’ its coaching on user-specified knowledge – a course of that may have an effect on the unique core performance of the mannequin, however considerably enhance its efficiency on the area that’s being ‘pushed’ into it or else emphasised throughout fine-training.
Within the first fine-tuning experiment, the staff skilled GPT‑4o‑mini on round two thousand passage-completion pairs drawn from books printed between 1905 and 1914, with the goal of seeing whether or not a smaller-scale fine-tuning might shift the mannequin’s outputs towards a extra traditionally correct fashion.
Utilizing the identical RoBERTa-based classifier that acted as a decide within the earlier exams to estimate the stylistic ‘date’ of every output, the researchers discovered that within the new experiment, the fine-tuned mannequin produced textual content carefully aligned with the bottom reality.
Its stylistic divergence from the unique texts, measured by Jensen-Shannon divergence, dropped to 0.002, usually in step with GPT‑1914:
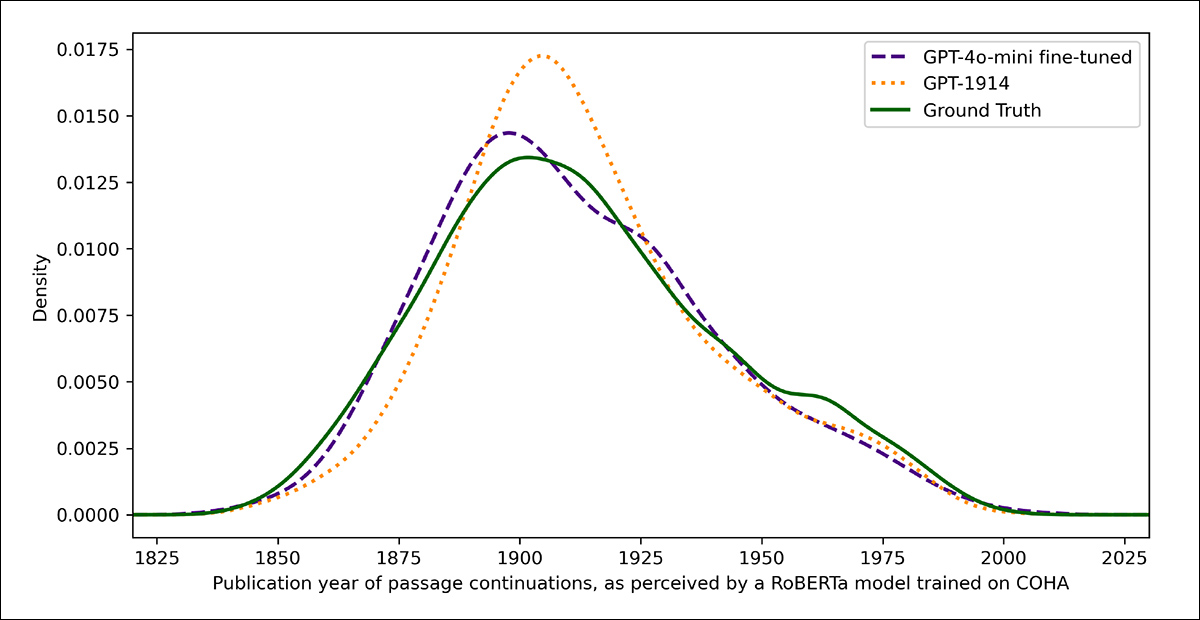
Estimated publication dates for actual and generated textual content, exhibiting how carefully GPT‑1914 and a fine-tuned model of GPT‑4o‑mini match the fashion of early twentieth-century writing (primarily based on books printed between 1905 and 1914).
Nonetheless, the researchers warning that this metric could solely seize superficial options of historic fashion, and never deeper conceptual or factual anachronisms.
‘[This] isn’t a really delicate take a look at. The RoBERTa mannequin used as a decide right here is barely skilled to foretell a date, to not discriminate genuine passages from anachronistic ones. It in all probability makes use of coarse stylistic proof to make that prediction. Human readers, or bigger fashions, may nonetheless be capable to detect anachronistic content material in passages that superficially sound “in-period.”‘
Human Contact
Lastly, the researchers carried out human analysis exams utilizing 250 hand-selected passages from books printed between 1905 and 1914, and so they observe that many of those texts would doubtless be interpreted fairly otherwise at the moment than they had been on the time of writing:
‘Our listing included, for example, an encyclopedia entry on Alsace (which was then a part of Germany) and one on beri-beri (which was then usually defined as a fungal illness slightly than a dietary deficiency). Whereas these are variations of truth, we additionally chosen passages that may show subtler variations of angle, rhetoric, or creativeness.
‘For example, descriptions of non-European locations within the early twentieth century have a tendency to slip into racial generalization. An outline of dawn on the moon written in 1913 imagines wealthy chromatic phenomena, as a result of nobody had but seen pictures of a world with out an [atmosphere].’
The researchers created brief questions that every historic passage might plausibly reply, then fine-tuned GPT‑4o‑mini on these query–reply pairs. To strengthen the analysis, they skilled 5 separate variations of the mannequin, every time holding out a special portion of the information for testing.
They then produced responses utilizing each the default variations of GPT-4o and GPT-4o‑mini, in addition to the fantastic‑tuned variants, every evaluated on the portion it had not seen throughout coaching.
Misplaced in Time
To evaluate how convincingly the fashions might imitate historic language, the researchers requested three skilled annotators to evaluate 120 AI-generated completions, and decide whether or not every one appeared believable for a author in 1914.
This direct analysis strategy proved tougher than anticipated: though the annotators agreed on their assessments practically eighty % of the time, the imbalance of their judgments (with ‘believable’ chosen twice as usually as ‘not believable’) meant that their precise degree of settlement was solely reasonable, as measured by a Cohen’s kappa rating of 0.554.
The raters themselves described the duty as tough, usually requiring extra analysis to judge whether or not a press release aligned with what was identified or believed in 1914.
Some passages raised tough questions on tone and perspective – for instance, whether or not a response was appropriately restricted in its worldview to mirror what would have been typical in 1914. This type of judgment usually hinged on the extent of ethnocentrism (i.e., the tendency to view different cultures by means of the assumptions or biases of 1’s personal).
On this context, the problem was to determine whether or not a passage expressed simply sufficient cultural bias to appear traditionally believable with out sounding too trendy, or too overtly offensive by at the moment’s requirements. The authors word that even for students aware of the interval, it was tough to attract a pointy line between language that felt traditionally correct and language that mirrored present-day concepts.
Nonetheless, the outcomes confirmed a transparent rating of the fashions, with the fine-tuned model of GPT‑4o‑mini judged most believable general:
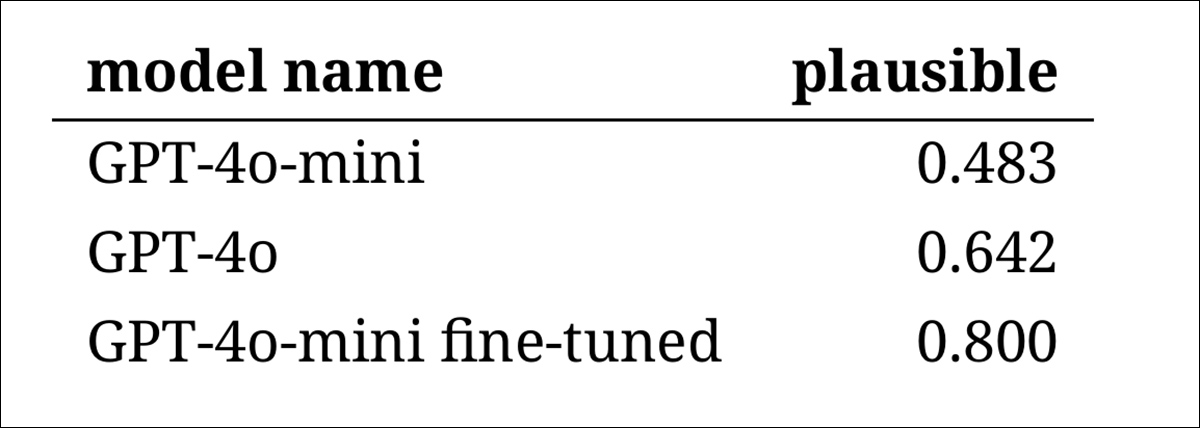
Annotators’ assessments of how believable every mannequin’s output appeared
Whether or not this degree of efficiency, rated believable in eighty % of instances, is dependable sufficient for historic analysis stays unclear – notably for the reason that examine didn’t embody a baseline measure of how usually real interval texts is likely to be misclassified.
Intruder Alert
Subsequent got here an ‘intruder take a look at’, whereby skilled annotators had been proven 4 nameless passages answering the identical historic query. Three of the responses got here from language fashions, whereas one was an actual and real excerpt from an precise early twentieth-century supply.
The duty was to establish which passage was the unique one, genuinely written throughout the interval.
This strategy didn’t ask the annotators to charge plausibility instantly, however slightly measured how usually the true passage stood out from the AI-generated responses, in impact, testing whether or not the fashions might idiot readers into pondering their output was genuine.
The rating of the fashions matched the outcomes from the sooner judgment job: the fine-tuned model of GPT‑4o‑mini was probably the most convincing among the many fashions, however nonetheless fell in need of the true factor.
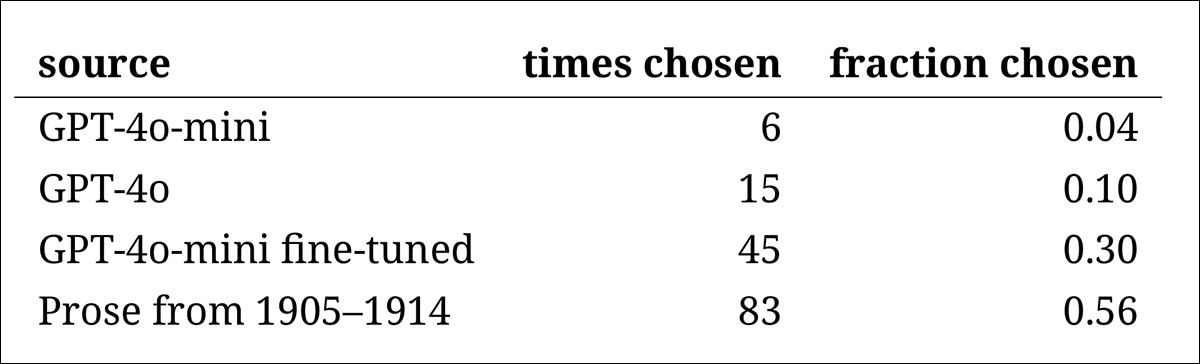
The frequency with which every supply was appropriately recognized because the genuine historic passage.
This take a look at additionally served as a helpful benchmark, since, with the real passage recognized greater than half the time, the hole between genuine and artificial prose remained noticeable to human readers.
A statistical evaluation referred to as McNemar’s take a look at confirmed that the variations between the fashions had been significant, besides within the case of the 2 untuned variations (GPT‑4o and GPT‑4o‑mini), which carried out equally.
The Way forward for the Previous
The authors discovered that prompting trendy language fashions to undertake a historic voice didn’t reliably produce convincing outcomes: fewer than two-thirds of the outputs had been judged believable by human readers, and even this determine doubtless overstates efficiency.
In lots of instances, the responses included specific alerts that the mannequin was talking from a present-day perspective – phrases corresponding to ‘in 1914, it’s not but identified that…’ or ‘as of 1914, I’m not aware of…’ had been widespread sufficient to seem in as many as one-fifth of completions. Disclaimers of this sort made it clear that the mannequin was simulating historical past from the skin, slightly than writing from inside it.
The authors state:
‘The poor efficiency of in-context studying is unlucky, as a result of these strategies are the best and least expensive ones for AI-based historic analysis. We emphasize that now we have not explored these approaches exhaustively.
‘It could end up that in-context studying is satisfactory—now or sooner or later—for a subset of analysis areas. However our preliminary proof isn’t encouraging.’
The authors conclude that whereas fine-tuning a business mannequin on historic passages can produce stylistically convincing output at minimal price, it doesn’t totally get rid of traces of recent perspective. Pretraining a mannequin completely on interval materials avoids anachronism however calls for far higher assets, and ends in much less fluent output.
Neither technique affords a whole resolution, and, for now, any try and simulate historic voices seems to contain a tradeoff between authenticity and coherence. The authors conclude that additional analysis shall be wanted to make clear how finest to navigate that stress.
Conclusion
Maybe probably the most fascinating inquiries to come up out of the brand new paper is that of authenticity. Whereas they don’t seem to be good instruments, loss capabilities and metrics corresponding to LPIPS and SSIM give pc imaginative and prescient researchers no less than a like-on-like methodology for evaluating in opposition to floor reality.
When producing new textual content within the fashion of a bygone period, against this, there isn’t a floor reality – solely an try and inhabit a vanished cultural perspective. Attempting to reconstruct that mindset from literary traces is itself an act of quantization, since such traces are merely proof, whereas the cultural consciousness from which they emerge stays past inference, and certain past creativeness.
On a sensible degree too, the foundations of recent language fashions, formed by present-day norms and knowledge, threat to reinterpret or suppress concepts that may have appeared cheap or unremarkable to an Edwardian reader, however which now register as (incessantly offensive) artifacts of prejudice, inequality or injustice.
One wonders, due to this fact, even when we might create such a colloquy, whether or not it won’t repel us.
First printed Friday, Might 2, 2025