The sort of content material that customers may need to create utilizing a generative mannequin comparable to Flux or Hunyuan Video will not be at all times be simply obtainable, even when the content material request is pretty generic, and one may guess that the generator might deal with it.
One instance, illustrated in a brand new paper that we’ll check out on this article, notes that the increasingly-eclipsed OpenAI Sora mannequin has some issue rendering an anatomically appropriate firefly, utilizing the immediate ‘A firefly is glowing on a grass’s leaf on a serene summer time night time’:
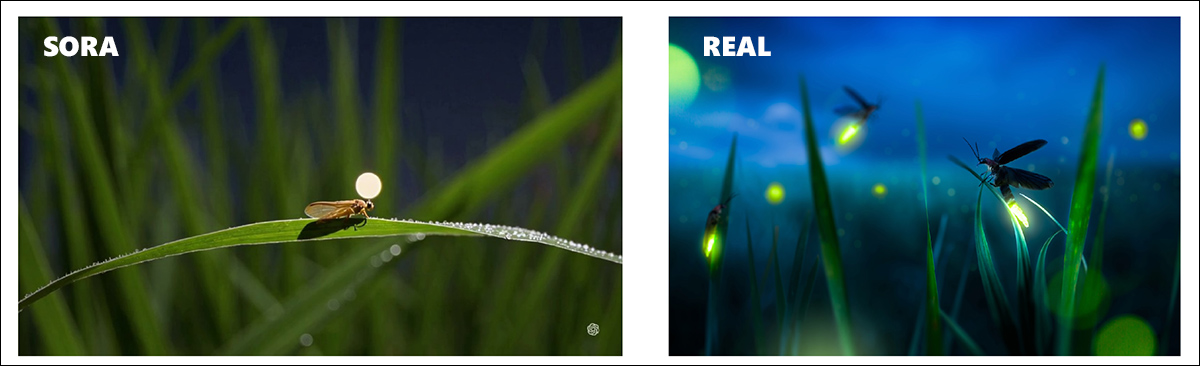
OpenAI’s Sora has a barely wonky understanding of firefly anatomy. Supply: https://arxiv.org/pdf/2503.01739
Since I not often take analysis claims at face worth, I examined the identical immediate on Sora in the present day and bought a barely higher consequence. Nonetheless, Sora nonetheless didn’t render the glow appropriately – fairly than illuminating the tip of the firefly’s tail, the place bioluminescence happens, it misplaced the glow close to the insect’s toes:
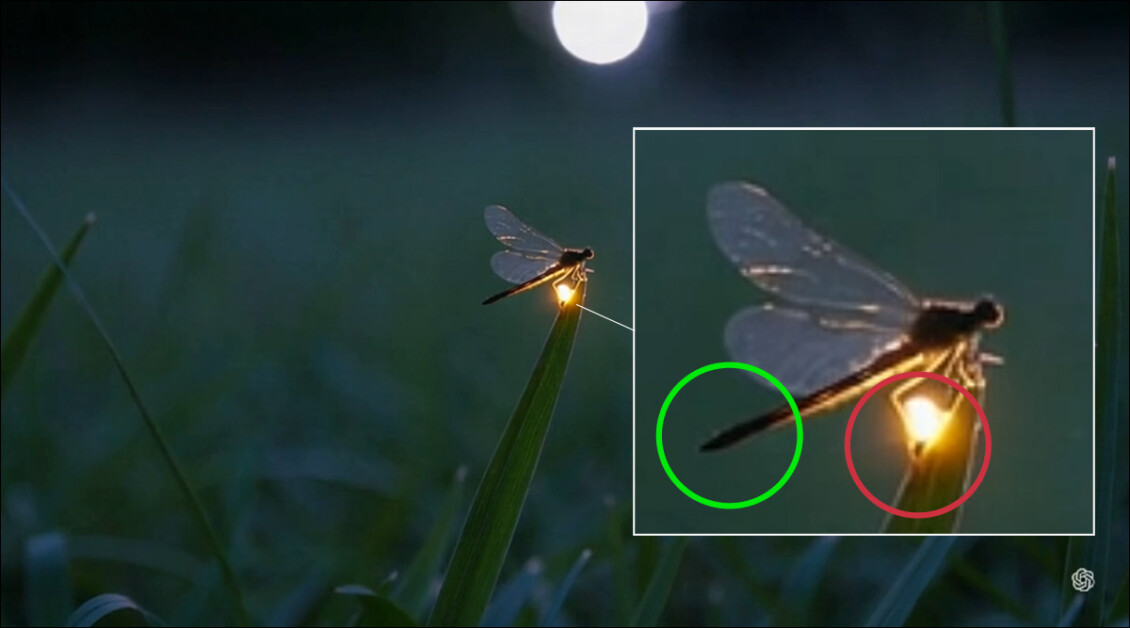
My very own check of the researchers’ immediate in Sora produces a consequence that exhibits Sora doesn’t perceive the place a Firefly’s gentle really comes from.
Satirically, the Adobe Firefly generative diffusion engine, skilled on the corporate’s copyright-secured inventory pictures and movies, solely managed a 1-in-3 success charge on this regard, after I tried the identical immediate in Photoshop’s generative AI characteristic:
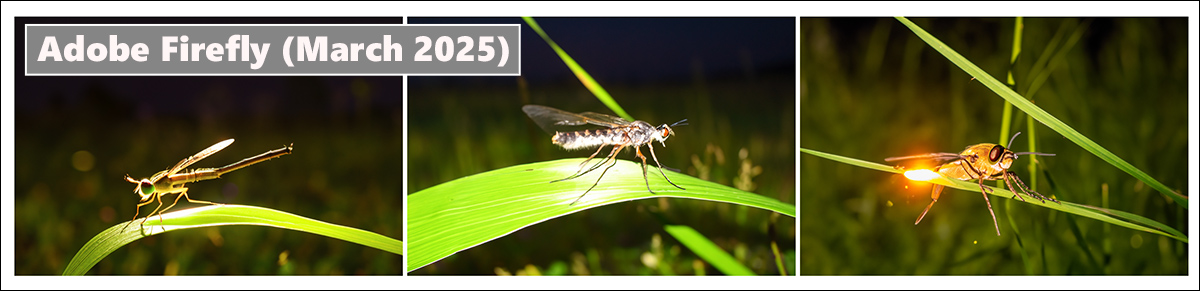
Solely the ultimate of three proposed generations of the researchers’ immediate produces a glow in any respect in Adobe Firefly (March 2025), although a minimum of the glow is located within the appropriate a part of the insect’s anatomy.
This instance was highlighted by the researchers of the brand new paper for example that the distribution, emphasis and protection in coaching units used to tell common basis fashions might not align with the person’s wants, even when the person just isn’t asking for something notably difficult – a subject that brings up the challenges concerned in adapting hyperscale coaching datasets to their most effective and performative outcomes as generative fashions.
The authors state:
‘[Sora] fails to seize the idea of a glowing firefly whereas efficiently producing grass and a summer time [night]. From the info perspective, we infer that is primarily as a result of [Sora] has not been skilled on firefly-related matters, whereas it has been skilled on grass and night time. Moreover, if [Sora had] seen the video proven in [above image], it would perceive what a glowing firefly ought to appear to be.’
They introduce a newly curated dataset and counsel that their methodology could possibly be refined in future work to create knowledge collections that higher align with person expectations than many present fashions.
Knowledge for the Individuals
Primarily their proposal posits an information curation method that falls someplace between the customized knowledge for a model-type comparable to a LoRA (and this method is much too particular for basic use); and the broad and comparatively indiscriminate high-volume collections (such because the LAION dataset powering Steady Diffusion) which aren’t particularly aligned with any end-use state of affairs.
The brand new method, each as methodology and a novel dataset, is (fairly tortuously) named Customers’ FOcus in text-to-video, or VideoUFO. The VideoUFO dataset includes 1.9 million video clips spanning 1291 user-focused matters. The matters themselves have been elaborately developed from an present video dataset, and parsed via numerous language fashions and Pure Language Processing (NLP) strategies:
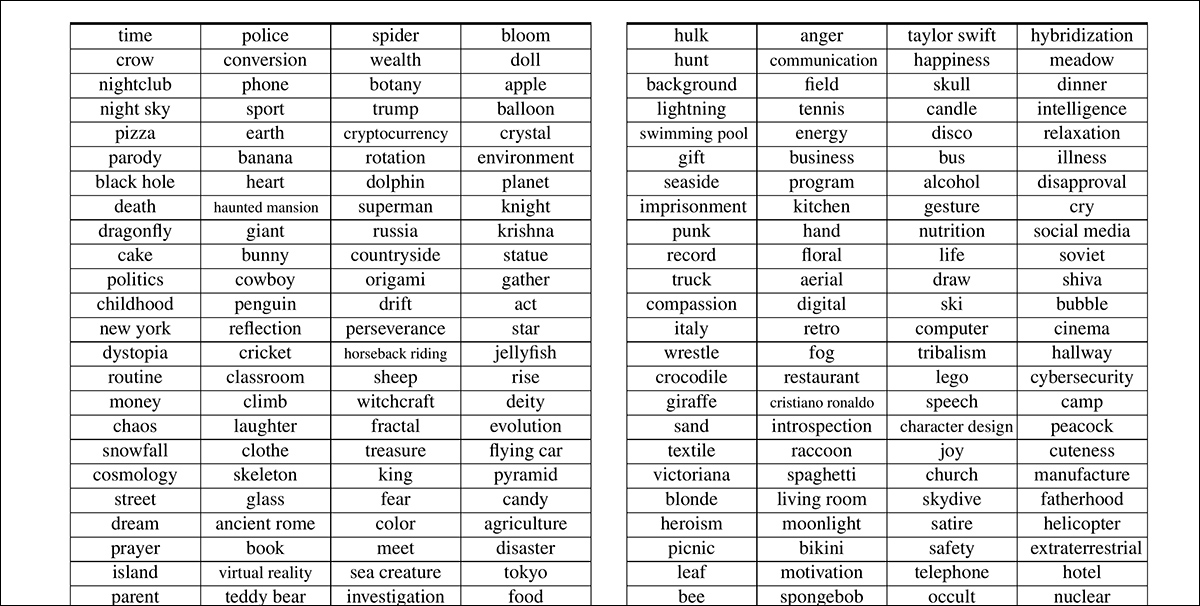
Samples of the distilled matters introduced within the new paper.
The VideoUFO dataset includes a excessive quantity of novel movies trawled from YouTube – ‘novel’ within the sense that the movies in query don’t characteristic in video datasets which might be presently common within the literature, and subsequently within the many subsets which were curated from them (and lots of the movies have been in actual fact uploaded subsequent to the creation of the older datasets thar the paper mentions).
In reality, the authors declare that there’s solely 0.29% overlap with present video datasets – a powerful demonstration of novelty.
One cause for this may be that the authors would solely settle for YouTube movies with a Inventive Commons license that might be much less prone to hamstring customers additional down the road: it is doable that this class of movies has been much less prioritized in prior sweeps of YouTube and different high-volume platforms.
Secondly, the movies have been requested on the idea of pre-estimated user-need (see picture above), and never indiscriminately trawled. These two elements together might result in such a novel assortment. Moreover, the researchers checked the YouTube IDs of any contributing movies (i.e., movies that will later have been break up up and re-imagined for the VideoUFO assortment) towards these featured in present collections, lending credence to the declare.
Although not every thing within the new paper is kind of as convincing, it is an fascinating learn that emphasizes the extent to which we’re nonetheless fairly on the mercy of uneven distributions in datasets, when it comes to the obstacles the analysis scene is commonly confronted with in dataset curation.
The new work is titled VideoUFO: A Million-Scale Person-Targeted Dataset for Textual content-to-Video Era, and comes from two researchers, respectively from the College of Know-how Sydney in Australia, and Zhejiang College in China.
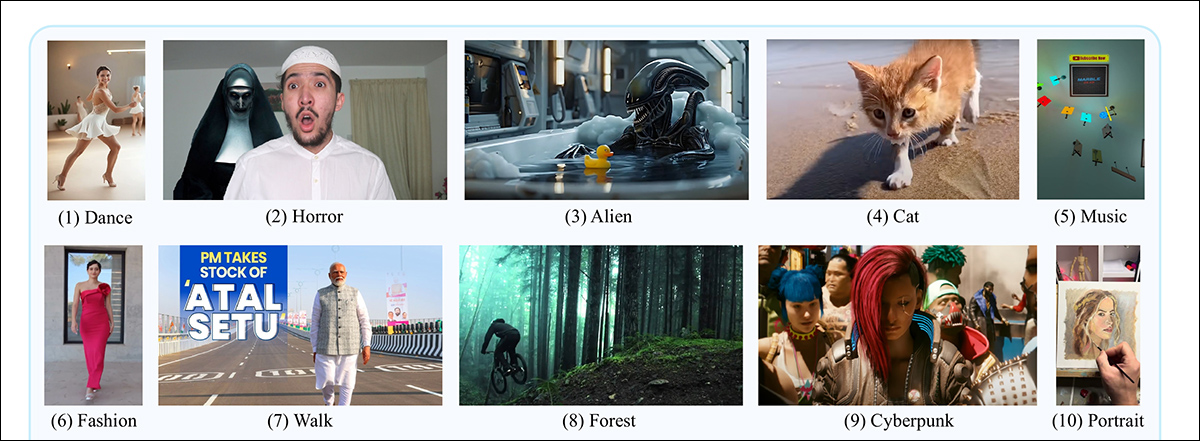
Choose examples from the ultimate obtained dataset.
A ‘Private Shopper’ for AI Knowledge
The subject material and ideas featured within the complete sum of web pictures and movies don’t essentially replicate what the typical finish person might find yourself asking for from a generative system; even the place content material and demand do are likely to collide (as with porn, which is plentifully obtainable on the web and of nice curiosity to many gen AI customers), this may occasionally not align with the builders’ intent and requirements for a brand new generative system.
In addition to the excessive quantity of NSFW materials uploaded each day, a disproportionate quantity of net-available materials is prone to be from advertisers and people making an attempt to govern website positioning. Industrial self-interest of this sort makes the distribution of material removed from neutral; worse, it’s tough to develop AI-based filtering programs that may deal with the issue, since algorithms and fashions developed from significant hyperscale knowledge might in themselves replicate the supply knowledge’s tendencies and priorities.
Subsequently the authors of the brand new work have approached the issue by reversing the proposition, via figuring out what customers are prone to need, and acquiring movies that align with these wants.
On the floor, this method appears simply as prone to set off a semantic race to the underside as to realize a balanced, Wikipedia-style neutrality. Calibrating knowledge curation round person demand dangers amplifying the preferences of the lowest-common-denominator whereas marginalizing area of interest customers, since majority pursuits will inevitably carry larger weight.
Nonetheless, let’s check out how the paper tackles the problem.
Distilling Ideas with Discretion
The researchers used the 2024 VidProM dataset because the supply for matter evaluation that might later inform the mission’s web-scraping.
This dataset was chosen, the authors state, as a result of it’s the solely publicly-available 1m+ dataset ‘written by actual customers’ – and it ought to be said that this dataset was itself curated by the 2 authors of the brand new paper.
The paper explains*:
‘First, we embed all 1.67 million prompts from VidProM into 384-dimensional vectors utilizing SentenceTransformers Subsequent, we cluster these vectors with Okay-means. Observe that right here we preset the variety of clusters to a comparatively massive worth, i.e., 2, 000, and merge related clusters within the subsequent step.
‘Lastly, for every cluster, we ask GPT-4o to conclude a subject [one or two words].’
The authors level out that sure ideas are distinct however notably adjoining, comparable to church and cathedral. Too granular a standards for instances of this sort would result in idea embeddings (for example) for every sort of canine breed, as a substitute of the time period canine; whereas too broad a standards might corral an extreme variety of sub-concepts right into a single over-crowded idea; subsequently the paper notes the balancing act crucial to judge such instances.
Singular and plural types have been merged, and verbs restored to their base (infinitive) types. Excessively broad phrases – comparable to animation, scene, movie and motion – have been eliminated.
Thus 1,291 matters have been obtained (with the total record obtainable within the supply paper’s supplementary part).
Choose Internet-Scraping
Subsequent, the researchers used the official YouTube API to hunt movies primarily based on the factors distilled from the 2024 dataset, looking for to acquire 500 movies for every matter. In addition to the requisite artistic commons license, every video needed to have a decision of 720p or larger, and needed to be shorter than 4 minutes.
On this means 586,490 movies have been scraped from YouTube.
The authors in contrast the YouTube ID of the downloaded movies to various common datasets: OpenVid-1M; HD-VILA-100M; Intern-Vid; Koala-36M; LVD-2M; MiraData; Panda-70M; VidGen-1M; and WebVid-10M.
They discovered that only one,675 IDs (the aforementioned 0.29%) of the VideoUFO clips featured in these older collections, and it must be conceded that whereas the dataset comparability record just isn’t exhaustive, it does embrace all the most important and most influential gamers within the generative video scene.
Splits and Evaluation
The obtained movies have been subsequently segmented into a number of clips, in line with the methodology outlined within the Panda-70M paper cited above. Shot boundaries have been estimated, assemblies stitched, and the concatenated movies divided into single clips, with temporary and detailed captions offered.
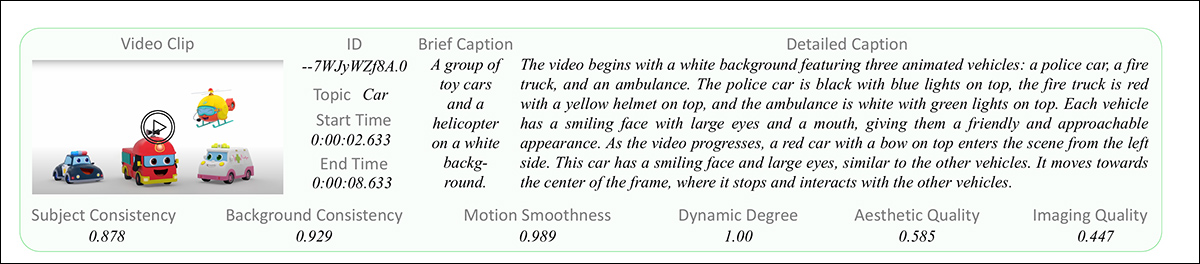
Every knowledge entry within the VideoUFO dataset includes a clip, an ID, begin and finish instances, and a quick and an in depth caption.
The temporary captions have been dealt with by the Panda-70M technique, and the detailed video captions by Qwen2-VL-7B, alongside the rules established by Open-Sora-Plan. In instances the place clips didn’t efficiently embody the meant goal idea, the detailed captions for every such clip have been fed into GPT-4o mini, to be able to verify whether or not it was really a match for the subject. Although the authors would have most popular analysis through GPT-4o, this might have been too costly for hundreds of thousands of video clips.
Video high quality evaluation was dealt with with six strategies from the VBench mission .
Comparisons
The authors repeated the subject extraction course of on the aforementioned prior datasets. For this, it was essential to semantically-match the derived classes of VideoUFO to the inevitably totally different classes within the different collections; it must be conceded that such processes provide solely approximated equal classes, and subsequently this can be too subjective a course of to vouchsafe empirical comparisons.
Nonetheless, within the picture under we see the outcomes the researchers obtained by this technique:
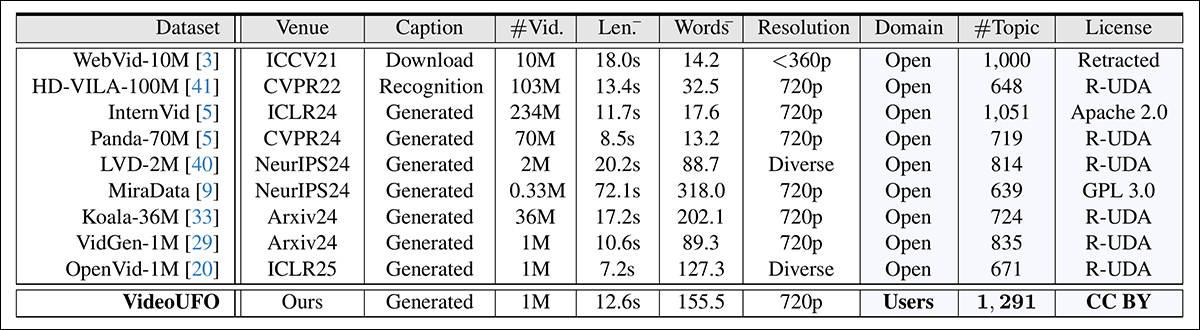
Comparability of the basic attributes derived throughout VideoUFO and the prior datasets.
The researchers acknowledge that their evaluation relied on the prevailing captions and descriptions offered in every dataset. They admit that re-captioning older datasets utilizing the identical technique as VideoUFO might have provided a extra direct comparability. Nonetheless, given the sheer quantity of knowledge factors, their conclusion that this method could be prohibitively costly appears justified.
Era
The authors developed a benchmark to judge text-to-video fashions’ efficiency on user-focused ideas, titled BenchUFO. This entailed deciding on 791 nouns from the 1,291 distilled person matters in VideoUFO. For every chosen matter, ten textual content prompts from VidProM have been then randomly chosen.
Every immediate was handed via to a text-to-video mannequin, with the aforementioned Qwen2-VL-7B captioner used to judge the generated outcomes. With all generated movies thus captioned, SentenceTransformers was used to calculate cosine similarity for each the enter immediate and output (inferred) description in every case.
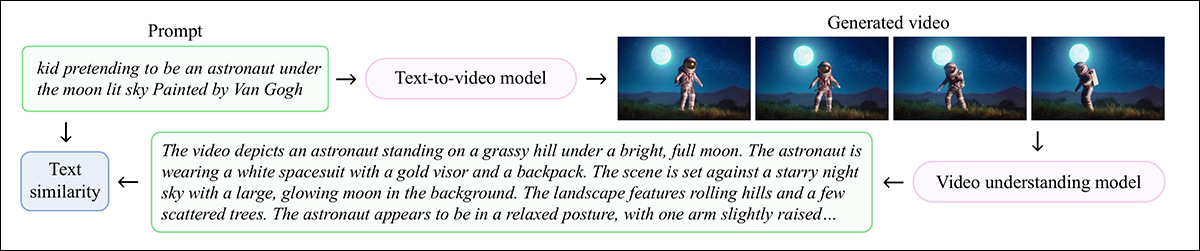
Schema for the BenchUFO course of.
The evaluated generative fashions have been: Mira; Present-1; LTX-Video; Open-Sora-Plan; Open Sora; TF-T2V; Mochi-1; HiGen; Pika; RepVideo; T2V-Zero; CogVideoX; Latte-1; Hunyuan Video; LaVie; and Pyramidal.
In addition to VideoUFO, MVDiT-VidGen and MVDit-OpenVid have been the choice coaching datasets.
The outcomes contemplate the Tenth-Fiftieth worst-performing and best-performing matters throughout the architectures and datasets.
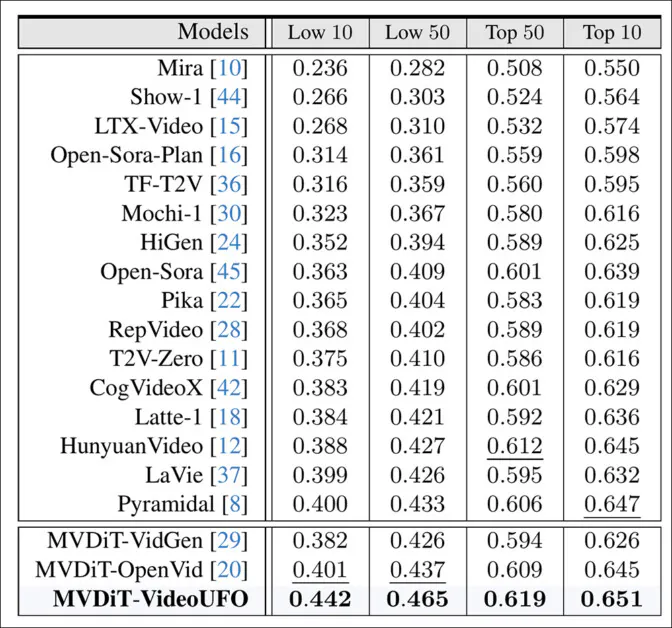
Outcomes for the efficiency of public T2V fashions vs. the authors’ skilled fashions, on BenchUFO.
Right here the authors remark:
‘Present text-to-video fashions don’t constantly carry out nicely throughout all user-focused matters. Particularly, there’s a rating distinction starting from 0.233 to 0.314 between the top-10 and low-10 matters. These fashions might not successfully perceive matters comparable to “big squid”, “animal cell”, “Van Gogh”, and “historic Egyptian” as a consequence of inadequate coaching on such movies.
‘Present text-to-video fashions present a sure diploma of consistency of their best-performing matters. We uncover that the majority text-to-video fashions excel at producing movies on animal-related matters, comparable to ‘seagull’, ‘panda’, ‘dolphin’, ‘camel’, and ‘owl’. We infer that that is partly as a consequence of a bias in direction of animals in present video datasets.’
Conclusion
VideoUFO is an excellent providing if solely from the standpoint of contemporary knowledge. If there was no error in evaluating and eliminating YouTube IDs, and if the dataset accommodates a lot materials that’s new to the analysis scene, it’s a uncommon and probably worthwhile proposition.
The draw back is that one wants to provide credence to the core methodology; if you happen to do not imagine that person demand ought to inform web-scraping formulation, you would be shopping for right into a dataset that comes with its personal units of troubling biases.
Additional, the utility of the distilled matters depends upon each the reliability of the distilling technique used (which is mostly hampered by funds constraints), and in addition the formulation strategies for the 2024 dataset that gives the supply materials.
That stated, VideoUFO definitely deserves additional investigation – and it’s obtainable at Hugging Face.
* My substitution of the authors’ citations for hyperlinks.
First revealed Wednesday, March 5, 2025